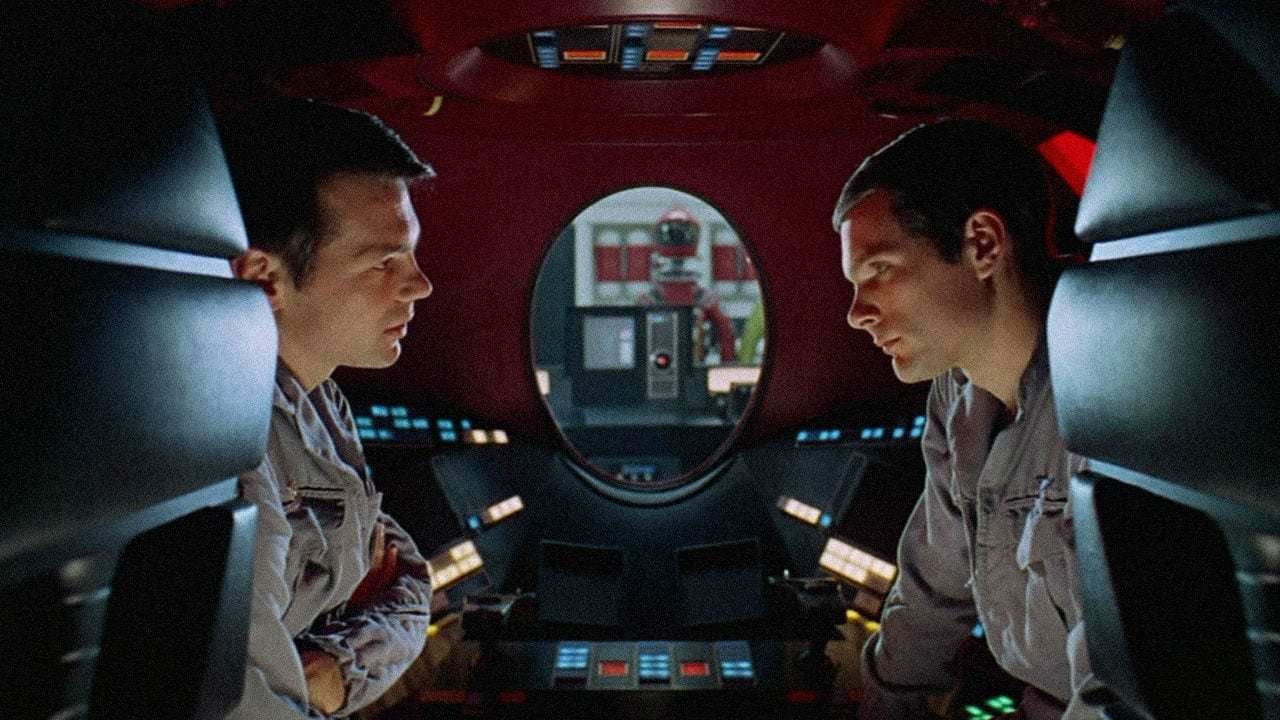
Hal 9000 read lips perfectly, though in English
Neural networks are able to do a lot now, and gradually they are being taught more and more skills. The other day it became known that a joint team of researchers from the USA and China was able to train the neural network to recognize speech by the lips with a high degree of accuracy.
This was achieved thanks to an additional element - the speech recognition algorithm for audio recordings . Further, the algorithm was used as a training system for the second algorithm, which recognized speech by video recordings.
According to scientists, this method makes it possible to master additional lip reading techniques that a neural network trained by traditional methods cannot โlearnโ. A simple sequence of images makes it possible to master only basic lip reading techniques.
In addition, the developers used a method of training neural networks, which is called "knowledge distillation." It allows you to save the small size of a model that performs a complex task. In a normal situation, a neural network that can read lips would have reached significant sizes, which would have made it difficult to use it on smartphones or other mobile devices.
But a model called knowledge distillation makes it possible to remove these limitations. In the course of working with this model, the developer needs to use a basic neural network that is already trained, and on its basis creates a much smaller model, which is "trained" on the basis of the first. Both networks receive almost the same source data. But the smaller network is trying to repeat the results of the larger one, both on the output layer and on all intermediate ones. The idea was first introduced by Caruana in 2006.
Scientists led by Mingli Song from Zhejiang University have used โdistillationโ to teach the neural network to read lips. As mentioned above, the teacher here is a speech recognition algorithm for audio recordings. It provides ample opportunity to study a number of subtle lip movements and speech patterns.

The final circuit is symmetrical, with two recurrent neural networks located parallel to each other. One convolutional neural network processes video frames and provides data for another. The researcher can only imagine the distillation of knowledge in the form of several blocks, each of which was responsible for a specific task. One of them is per frame, the second is for a sequence of data, the third is for the largest overall sequence.
Of course, such a neural network requires careful training on tens of thousands of elements for normal operation. Scientists have used the LRS2 dataset, which contains about 50,000 individual sentences spoken by the BBC announcers, as well as the CMLR dataset, the most comprehensive set for teaching neural networks to read lips in Mandarin. The database of the latter contains about 100 thousand offers from CNTV.

The recognition accuracy of the resulting system is approximately 8% higher than that of other neural networks that trained on CMLR, and 3% better than that of neural networks that trained on LRS2.