After successfully predicting earthquakes in the laboratory, a team of geophysicists applied the machine learning algorithm to earthquakes in the northwest Pacific coast

The remains of a 2000-year-old coniferous forest on Nescovin Beach, Oregon - one of dozens of "ghost forests" located along the coasts of Oregon and Washington. It is believed that a large-scale earthquake once shook the subduction zone of Cascadia , and stumps were buried under the debris brought by the tsunami
Last May, after a 13-month nap, the land under Puget Gulf of Washington came into motion with a roar. The earthquake began at a depth of more than 30 km under the Olympic Mountains, and within a few weeks moved northwest, reaching the Canadian island of Vancouver. Then it turned its course briefly, crawled back along the US border, and then fell silent. A monthly earthquake released enough energy so that its magnitude could be estimated at 6. By the end of the earthquake, the southern end of Vancouver Island advanced an extra centimeter into the Pacific Ocean.
Since the earthquake was so blurred in space and time, most likely, no one felt it. Such ghostly earthquakes that occur deeper underground than ordinary, fast earthquakes are known as “slow slippages”. About once a year, they occur in the Pacific Northwest, along a fault along which the Juan de Fuca plate slowly creeps under the northern part of the western edge of the North American Plate . Since 2003, over a dozen slow slips have been recorded in a wide network of seismological stations in the region. And over the past year and a half, these events have been the focus of the earthquake prediction project, led by geophysicist Paul Johnson .
Johnson's team is one of several groups of scientists using machine learning to try to uncover the secrets of earthquake physics and isolate the signs of an emerging earthquake. Two years ago, Johnson and colleagues successfully predicted earthquakes in a laboratory model using a pattern-seeking algorithm similar to the ones used during recent breakthroughs in image and speech recognition and other applications of artificial intelligence. Since then, this achievement has been repeated by scientists from Europe.
And now, in a paper published this September on the preprint site arxiv.org, Johnson and his team report testing their algorithm for slow earthquakes in the Pacific Northwest. The work has yet to be tested by independent experts, but they are already reporting that the results have been promising. Johnson argues that the algorithm can predict the onset of a slow earthquake "in a few days - and possibly earlier."
“This is a very interesting development,” said Maarten de Hoop , a seismologist at Rice University who is not involved in this work. “For the first time, the moment has come when we have made progress” in predicting earthquakes.
Mostafa Mousavi, a geophysicist at Stanford University, called the new results “interesting and motivating.” He, de Hoop and other experts in this field emphasize that machine learning still has a long way to go before it begins to predict catastrophic earthquakes - and that some obstacles to this path can be very difficult, and possibly insurmountable. Nevertheless, machine learning may turn out to be the best chance for scientists in the field in which they have stagnated for decades and have hardly seen any glimmers of hope.
Jams and Slips
The late seismologist Charles Richter , who was named after the earthquake strength rating scale, noted in 1977 that earthquake prediction could be “excellent soil for hobbyists, loonies and public scammers.” Today, many seismologists will confirm to you that they have met quite a lot of representatives of all three types.
However, it happened that respected scientists gave out ideas that, in retrospect, seem far from the truth, and sometimes just crazy. A geophysicist at the University of Athens, Panagiotis Varotsos, stated that he was able to recognize impending earthquakes by measuring "seismic electrical signals." Brian Brady, a physicist at the U.S. Department of Mines in the early 1980s, raised a false alarm several times about the impending earthquakes in Peru, basing his findings on unconfirmed conclusions that cracking stones in mines was a sign of impending earthquakes.
Paul Johnson is aware of this controversial story. He knows that in many places it’s even indecent to talk about “earthquake predictions”. He knows that six Italian scientists were convicted of unintentional killing of 29 people in 2012, downplaying the chances of an earthquake in the Italian city of L'Aquila a few days before the region was almost destroyed by an earthquake of magnitude 6.3 (then the court of appeal canceled this verdict). He knows about prominent seismologists who have convincingly stated that "earthquakes cannot be predicted."
However, Johnson also knows that earthquakes are physical processes that do not essentially differ from the collapse of a dying star or a change in wind direction. And although he emphasizes that the main goal of his research is to better understand the physics of faults, he does not refuse the task of predictions.

Paul Johnson, a seismologist from the Los Alamos National Laboratory with a sample of acrylic plastic in his hands - one of the materials used by the team to simulate earthquakes in the laboratory
More than a decade ago, Johnson began to study “laboratory earthquakes,” which are simulated using blocks gliding along thin layers of granular material. These blocks, like tectonic plates, do not slide smoothly, but with snatches and stops. Sometimes for a few seconds they freeze, held by friction, and then the increasing force is sufficient so that they suddenly begin to slide further. This slippage - the laboratory version of the earthquake - releases stress, after which the cycle of ragged movements begins anew.
When Johnson and colleagues recorded the acoustic signal that occurs during this intermittent movement, they noticed sharp peaks that appear before each slippage. These events prior to the movement became the laboratory equivalent of seismic waves that produce shocks that precede earthquakes. However, just as seismologists unsuccessfully tried to turn preliminary shocks into a prediction of the moment of the onset of the main earthquake, Johnson and colleagues could not figure out how to turn these pre-events into reliable predictions of laboratory earthquakes. “We are at a dead end,” Johnson recalls. “I have not seen a way to continue.”
At a meeting in Los Alamos a few years ago, Johnson explained this dilemma to a group of theorists. They proposed to reanalyze the data using machine learning algorithms - this approach by that time was already known for its ability to well recognize patterns in audio data.
Scientists have jointly developed a plan. They decided to take five minutes of audio recorded during the experiments - which included about 20 cycles of slipping and getting stuck - and cut them into many small segments. For each segment, the researchers calculated more than 80 statistical features, including the average signal, deviations from the average, and information on whether this segment contains sound preceding the shift. As the researchers analyzed the data retroactively, they knew how much time passed between each segment with sound and the subsequent push in the laboratory.
Armed with this data for training, they used a machine learning algorithm called " random forest " to systematically search for combinations of attributes that are clearly related to the amount of time left before the shift. After studying several minutes of experimental data, the algorithm could begin to predict shear time based on acoustic features.
Johnson and colleagues chose the random forest algorithm to predict the time remaining until a new shift, in particular because (compared with neural networks and other popular machine learning algorithms) the random forest is relatively easy to interpret. The algorithm works, in essence, as a decision tree, in which each branch shares a data set based on some statistical feature. Therefore, the tree keeps records of what signs the algorithm used for predictions - and the relative importance of each of the signs that helped the algorithm come to a certain prediction.

Polarized lenses show accumulation of stress before the tectonic plate model moves sideways along the fault line.
When researchers from Los Alamos studied the details of their algorithm, they were surprised. For the most part, the algorithm relied on a statistical feature not related to events that occurred immediately before the laboratory earthquake. Its greater variance is a measure of the deviation of the signal from the average — moreover, it’s spread over the entire cycle of braking and sliding, and not concentrated in the moments immediately preceding the shift. The dispersion began with small values, and then gradually accumulated during the approach to the shift, probably because the grains between the blocks more and more collided with each other as the voltage accumulated. Knowing this variance, the algorithm was able to predict well the start time of the shift; and information about immediately preceding events helped clarify these conjectures.
This discovery can have serious consequences. For decades, people have been trying to predict earthquakes based on preliminary shocks and other isolated seismic events. The result from Los Alamos suggests that they were all looking in the wrong place - and that the key to the predictions was less explicit information that could be collected during relatively quiet periods between major seismic events.
Of course, the sliding blocks of plastic do not closely describe the chemical, thermal and morphological complexity of real geological faults. To demonstrate the power of machine learning in predicting real earthquakes, Johnson needed to test it on real faults. Is there a better place, he thought, than the Pacific Northwest Coast?
Exit from the laboratory
Most of the places on Earth where earthquakes of magnitude 9 may occur are subduction zones where one tectonic plate creeps under another. The subduction zone east of Japan is responsible for the Tohoku earthquake and subsequent tsunami that destroyed the country's coast in 2011. Once, the Cascadia subduction zone, in which the Juan de Fuca plate creeps under the northern part of the western edge of the North American plate, will also destroy the bay Puget, Vancouver Island and the surrounding Pacific Northwest.
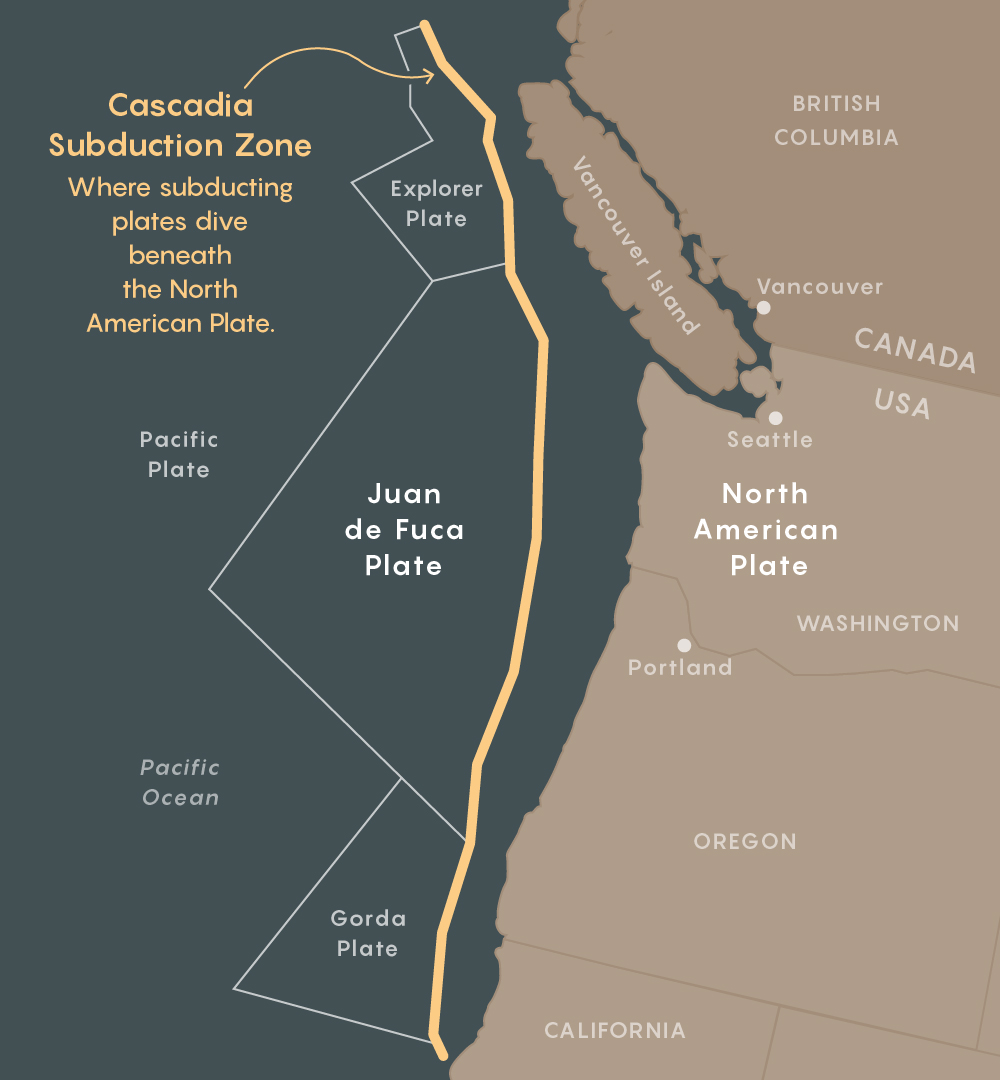
The Cascadia subduction zone extends for 1000 km along the Pacific coast from Cape Mendochino in California to Vancouver Island. The last time there was an earthquake in January 1700, it caused tremors with a magnitude of 9 points and a tsunami that reached the coast of Japan. Geological surveys indicate that during the Holocene, this fault generated similar mega-earthquakes about once every half a million years, plus or minus several hundred years. Statistically, the following can happen in any century.
This is one of the reasons seismologists are paying close attention to the slow earthquakes in this region. It is believed that slow earthquakes in the lower part of the subduction zone carry a small amount of stress into the brittle crust located above, where fast and destructive shocks occur. With every slow earthquake, the chances of a mega-earthquake in the Puget Bay region - Vancouver Islands are slightly higher. Indeed, in Japan a slow earthquake was observed several months before the Tohoku earthquake.
But Johnson has another reason to keep track of slow earthquakes: they produce a huge amount of data. For comparison, over the past 12 years, there has not been a single large rapid earthquake in the fault of Puget Bay - Vancouver Island. But over the same period, this fault provoked more than ten slow earthquakes, and each of them was carefully recorded in the seismic catalog.
This seismic catalog is a real copy of the acoustic records obtained in Johnson's laboratory experiments with earthquakes. Johnson and colleagues, in the same way as in the case of their laboratory acoustic recordings, broke the seismic data into small segments, and described each of them with a set of statistical features. Then they fed this data and information about when the previous slow earthquakes occurred to their machine learning algorithm.
Having trained on the data from 2007 to 2013, the algorithm was able to successfully predict the slow earthquakes that occurred from 2013 to 2018 based on the data recorded several months before each event. The key factor was seismic energy, a value closely related to the dispersion of the acoustic signal in laboratory experiments. Like dispersion, seismic energy characteristically grew in anticipation of every slow earthquake.
The predictions for the Cascadia subduction zone were not as accurate as for laboratory earthquakes. Correlation coefficients, characterizing the quality of coincidence of predictions with observations, were significantly less in the new results than in the laboratory. And yet, the algorithm was able to predict all but one earthquake from 2013 to 2018, indicating the start dates, according to Johnson, to within a few days (the August 2019 slow earthquake was not included in the study).
For de Hoop, the main conclusion is that "machine learning technologies have given us an entry point, a data analysis method for finding things that we have not seen or searched before." However, he warns that there is still much work to be done. “We have taken an important step - an extremely important one. However, this is a tiny step in the right direction. "
Sobering truth
The purpose of earthquake prediction has never been to predict slow earthquakes. Everyone needs to predict sudden and catastrophic tremors that threaten life and health. For machine learning, this seemed to represent a paradox: the largest earthquakes that seismologists would most like to predict are the least likely to occur. How can a machine learning algorithm get enough training data to confidently predict it?
The Los Alamos group believes that, in principle, their algorithm will not need to be trained on data obtained from records of catastrophic earthquakes in order to successfully predict them. Recent studies suggest that seismic patterns that precede small earthquakes are statistically similar to those that precede large ones, and dozens of small earthquakes can occur in a single fault any day. Having learned from thousands of these small shocks, the computer may be able to predict large ones. Also, machine learning algorithms may be able to learn from computer simulations of fast earthquakes, which one day can become a substitute for real data.
But all the same, scientists are confronted with a sobering truth: although the physical processes that lead to a fault on the verge of an earthquake can become predictable, the very occurrence of an earthquake - the growth of small seismic disturbances, leading to a full-scale fault break - in the opinion of most scientists, contains an element of chance. If so, then regardless of the quality of machine learning, they may never be able to predict earthquakes the way scientists were able to predict other natural disasters.
“We don’t yet know how accurate the dates will be to make predictions,” Johnson said. - Will it be like a prediction of hurricanes? No I do not think so".
At best, predictions of large earthquakes will give time frames of weeks, months, or years. Such predictions cannot be used, for example, to organize the mass evacuation of cities on the eve of tremors. But they can improve preparation for this event, help officials focus on strengthening unsafe buildings, and otherwise reduce the risk of a catastrophic earthquake.
Johnson believes that such a goal is worth achieving. But, being a realist, he realizes that it will take a lot of time. “I am not saying that we will learn to predict earthquakes in my lifetime,” he said, “but we will make tremendous progress in this direction.”