I had a week for experiments, our data analysis engines, 16 thousand Russian novels and novels of the XIX century and 15 thousand modern long works. And, of course, there was no tagged data.
The main idea was to extract fragments of descriptions of beautiful women from this mountain of texts, and then to extract frequency lines of appearance from these fragments.
Here is a visualization of what happened. More precisely, one of the common options.
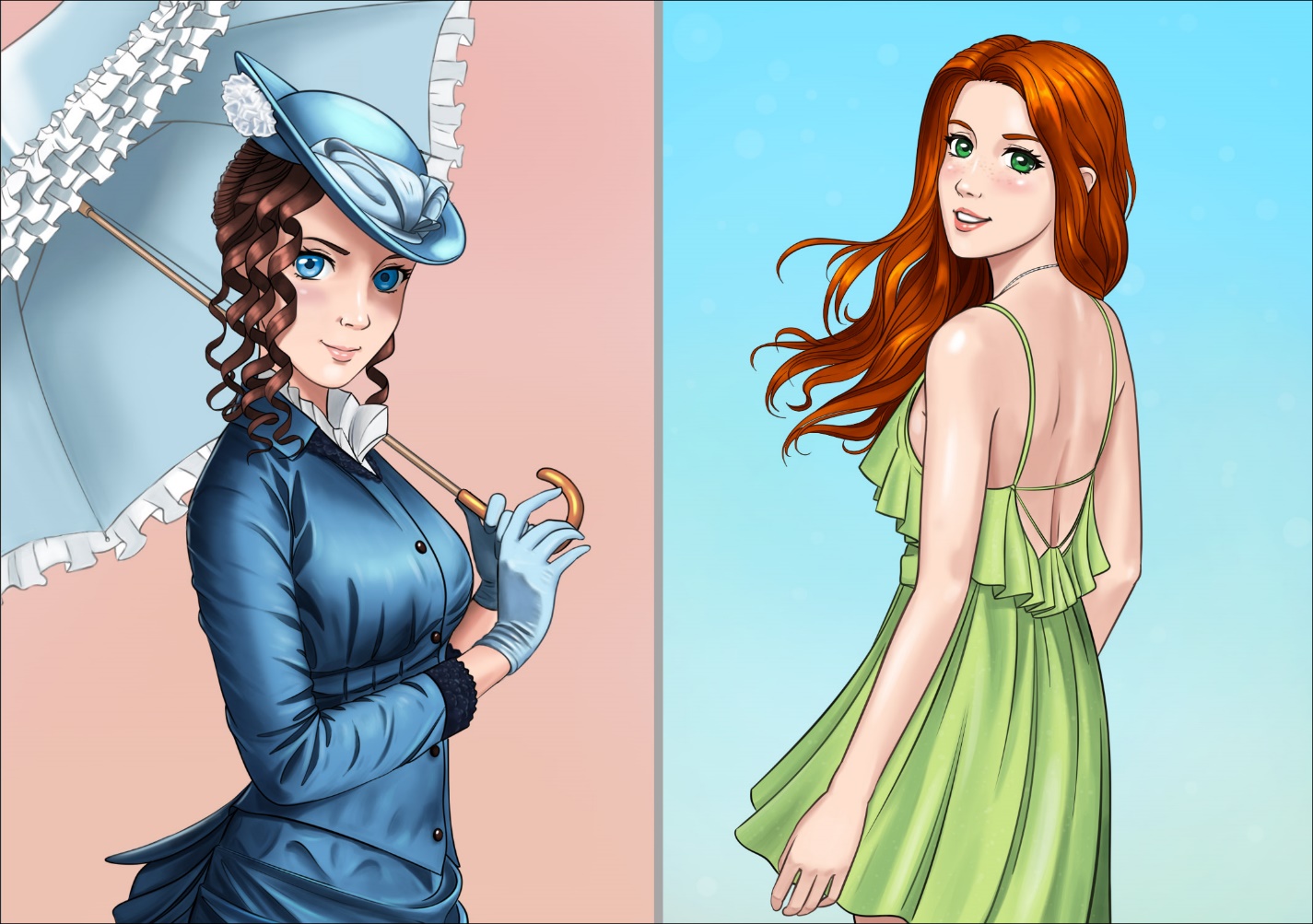
The color of eyes, hair, dress, growth, education - all this can be distinguished from the body of texts.
Of course, not everything is so simple and unambiguous as in the figures, but you have already received a rough idea. Now let's talk about the details and sequence of actions.
Text Corpus
I managed to find resources with an open license for the distribution of texts. Thanks to those people who collected and posted all this.
Both the 19th century and the present included only original Russian-language texts in the corps, that is, there is no translated literature.
I did all the analysis using a bunch of SAS Visual Text Analytics and Python libraries (pymorphy2, gensim, tensorflow).
Step 1. Linguistic rules
So, first it was necessary to highlight fragments with descriptions of female appearance. There was no marked data, so I started with simple rules in the spirit of “girl AND (eyes OR hair OR face)”. The rules were written in SAS Visual Text Analytics, so that they took into account morphological forms, typos (for the modern case it was relevant), simple syntax, the distance between tokens and filtered unwanted contexts.
Simplified rule
PREDICATE_RULE: (arg1, arg2, arg3): (UNLESS, "bad_contexts", (SENT_5, "_arg1 {beauty}", "_arg2 {woman}", "_arg3 {traits}"))
In other words, within the five sentences there should be a mention of a woman, a mention of the fact of her attractiveness, a description of any appearance, and there should not be any undesirable contexts.
Writing such a rule is not difficult, the problem is in the details. How, for example, to collect all possible references to women? Indeed, in the text it can be “mistress”, and “girl”, and “Margarita”, and “cousin”. Simple synonyms are indispensable here, not a single dictionary will give out “typist” or “student” as a synonym for “woman”. You can list "from the head", while there is enough imagination, but the list will be incomplete (and it’s boring).
To expand the rules and search for contextual synonyms, we connect vector representations.
Step 2. The word2vec Model
Word2vec is a word vectorization tool that is based on the idea of "tell me who is standing next to you and I will tell who you are." For example, in the sentence “I ___ her at first sight”, most would replace the pass with a word like “loved”. The idea is simple - similar words are found in similar contexts. For the Russian language, there are ready-made pre-trained models that are posted here . Experience on projects shows that models trained in the subject area work better than models "for the whole language", so I trained two models on my bodies.
First, she broke the corps with python into words, brought the words to the initial form (thanks to pymorphy2), extracted frequency verbose expressions like a cousin, lion's mane, wasp waist (thanks to phrases from gensim). On the processed data, I learned the word2vec model (skipgram algorithm, window - 3, dimension - 300).
Using the trained model, iteratively compiled lists of similar words. The most_similar function in gensim takes a word as input and returns a list of words / expressions whose vectors are close in cosine measure to the vector of the original word.
Vectors close to the vector of the word "beauty" on the body of the XXI century. The second value is the cosine measure.
('beauty', 0.6690341234207153)
('pretty', 0.6438576579093933)
('charming', 0.6156517267227173)
('smart girl', 0.6063219308853149)
('handsome', 0.6044491529464722)
('girly', 0.5829722285270691)
('blue-eyed', 0.5814758539199829)
('young lady', 0.5773882865905762)
('princess', 0.5754760503768921)
('bright', 0.5743755102157593)
('blond', 0.5731547474861145)
('blue-eyed', 0.5724368095397949)
('pretty', 0.6438576579093933)
('charming', 0.6156517267227173)
('smart girl', 0.6063219308853149)
('handsome', 0.6044491529464722)
('girly', 0.5829722285270691)
('blue-eyed', 0.5814758539199829)
('young lady', 0.5773882865905762)
('princess', 0.5754760503768921)
('bright', 0.5743755102157593)
('blond', 0.5731547474861145)
('blue-eyed', 0.5724368095397949)
The problem here was that among similar vectors, antonyms can come across, since they can be in the same contexts. For example, at the place of the pass in the example about “fell in love at first sight” it may well be the antonym “hated at first sight”. In our case, for example, the word “young man” is closest to the word “girl”, and only after it comes “women”, “ladies”, etc. The problem with antonyms was solved simply by manual selection. But there were few antonyms, so it took a little effort.
By the way, it is funny that similar words to a woman of the 19th century are all sorts of family concepts (daughter, sister, cousin) or serving professions (maid, maid, cook), social status by husband (admiral, general, baroness). In the 21st century, the spectrum is expanding: there is a student, classmate, athlete, laboratory assistant, Komsomol member, translator, and leader.
Women of the XIX century:
Katerina
Katya
Claudia
Clotilde
princess
princess
yoke
companion
nurse
beauty
peasant woman
lace maker
cousin
chrysalis
cummy
merchant woman
cook
Katya
Claudia
Clotilde
princess
princess
yoke
companion
nurse
beauty
peasant woman
lace maker
cousin
chrysalis
cummy
merchant woman
cook
Women of the XXI century:
Karen
Karina
cashier
Katerina
Katrina
Katka
Katya
tenant
Kira
Clara
client
yoke
Komsomol member
queen
beauty
beautiful girl
Kristina
Kseniya
Ksenia
cousin
Karina
cashier
Katerina
Katrina
Katka
Katya
tenant
Kira
Clara
client
yoke
Komsomol member
queen
beauty
beautiful girl
Kristina
Kseniya
Ksenia
cousin
Used the same principle to expand the remaining rules.
For example, to extract hair contexts:
mane
curl
mop
scythe
pigtail
curls
curly hair
curl
hairstyle
strand
lock
beam
a haircut
bang
bang
hair
tail
tail
curl
mop
scythe
pigtail
curls
curly hair
curl
hairstyle
strand
lock
beam
a haircut
bang
bang
hair
tail
tail
Step 3. Unwanted contexts
So, I have long detailed rules, which quite successfully catch a description of appearance, mentioning a woman and mentioning the fact of her attractiveness. I prescribe obvious restrictions in linguistic rules: one must take into account negatives, modality, conditional mood so that contexts such as “not distinguished by beauty”, “far from beauty” are not caught.
We don’t need this.
In her youth she was not at all a beauty, but rather a well-fed girl with a wide duck nose. She was very worried about her nose, and according to the stories of her sisters, she often slept with a wooden pluck for laundry on her nose in order to narrow it.
P. Rebenina, “Unfortunate Zinka”.
P. Rebenina, “Unfortunate Zinka”.
In addition, surprisingly often, authors manage to describe generally repulsive characters that have one nice touch. These contexts are difficult to handle, they can make noise, so I just remove them from consideration.
Now I have in my hands fragments of texts with markup based on rules and vector representations. Although it took a couple of days to clarify the rules, the contexts found have an error, which suits me quite well for this task. For example, some descriptions of the appearance were not extracted due to the fact that it is not clear about the woman or the man in question: “Vali had gray-blue eyes hiding under the thin glasses of glasses.” In principle, this ambiguity could be resolved on the basis of a larger fragment of the text, but I had only a week, so I left all the inaccuracies to wait in the wings.
Here is the markup.
Examples of parsing, context is highlighted in bold , underlining are facts about appearance. Except some. And this link is also not a link and does not click!
Alina , after all, was from a different circle, and in general everything else. She was very beautiful : a brunette with gray-blue eyes , a sloping forehead , a neat nose , a chiseled face , thin wrists, which dangled the most stylish baubles I have ever seen in my life. She was a cut above my head, her figure was ... well, no kidding, cool .K. Belozyorova, “A Friend Who Is Not”.
She was not one of those who climb into her pocket for a word, her natural beauty and attractiveness fascinated and beckoned. The high forehead was half covered by a smooth bang , smooth black hair , gleaming in the light of the bistro lamps, reached the shoulders , gently flowing along the graceful tanned neck . Her green eyes showed a clear interest in my person: Alena kept rubbing the thin nose bridge with the index finger of her right hand, which indicated her embarrassment. At my next joke, the girl laughed, and this made her sensual lips stretch out into a smile, and dimples appeared near the corners of her lips. I caught myself thinking that I really want this evening to never end.D. Ilyin, “Crossroads of Fate.”
There was something mysterious and attractive in her , she was slim and pretty . Long, slightly curly blonde hair, regular features , very lively blue eyes made Lena charming . Boris liked her mischievous smile, sensual mouth, her gaiety. Both her appearance and her manner of holding seemed irresistibly attractive .A. Bolshakov, “Outcast”.
She was a very beautiful woman with sharp features, a sharp nose and a chiseled chin , her name was no less impressive - Adelaide. She went out to meet me in a long bright green dress, and on her chest and hands hung numerous some outlandish ethnic ornaments. “You can just Ida,” she said affably, and the corners of her thin mouth went asymmetrically apart. “What a beauty with a twist!” I thought.O. Pavlenko, "The Tale of the Witches."
At the door of the next room stood a young woman with a candle in her hands ... I looked and was amazed - she was so beautiful in a white hood, with her hair loose over her shoulders. What a lovely trait , despite the fact that they were distorted by anger! Blue eyes with dilated pupils shone with an ominous brilliance ... The figure is slender , flexible.K. Stanyukovich, “The Original Couple”.
And Jacob had something to love his young woman: a woman - a hardworking woman , not empty, not a teardrop, a healthy and beautiful woman . Her face is oblong , with a straight, thin nose and with puffy, scarlet lips . Her blue eyes gaze openly at the white light. And above them, like a brush, held dark eyebrows . A thick blush plays on her tanned cheeks .P. Zasodimsky, "From the plow to the gun."
Step 4. Assembling the result
It remains to collect Frankenstein and combine the most frequency features. Some signs had a very close frequency, so we allowed ourselves to fantasize a little and collect a few characters.
The first two types of trait:
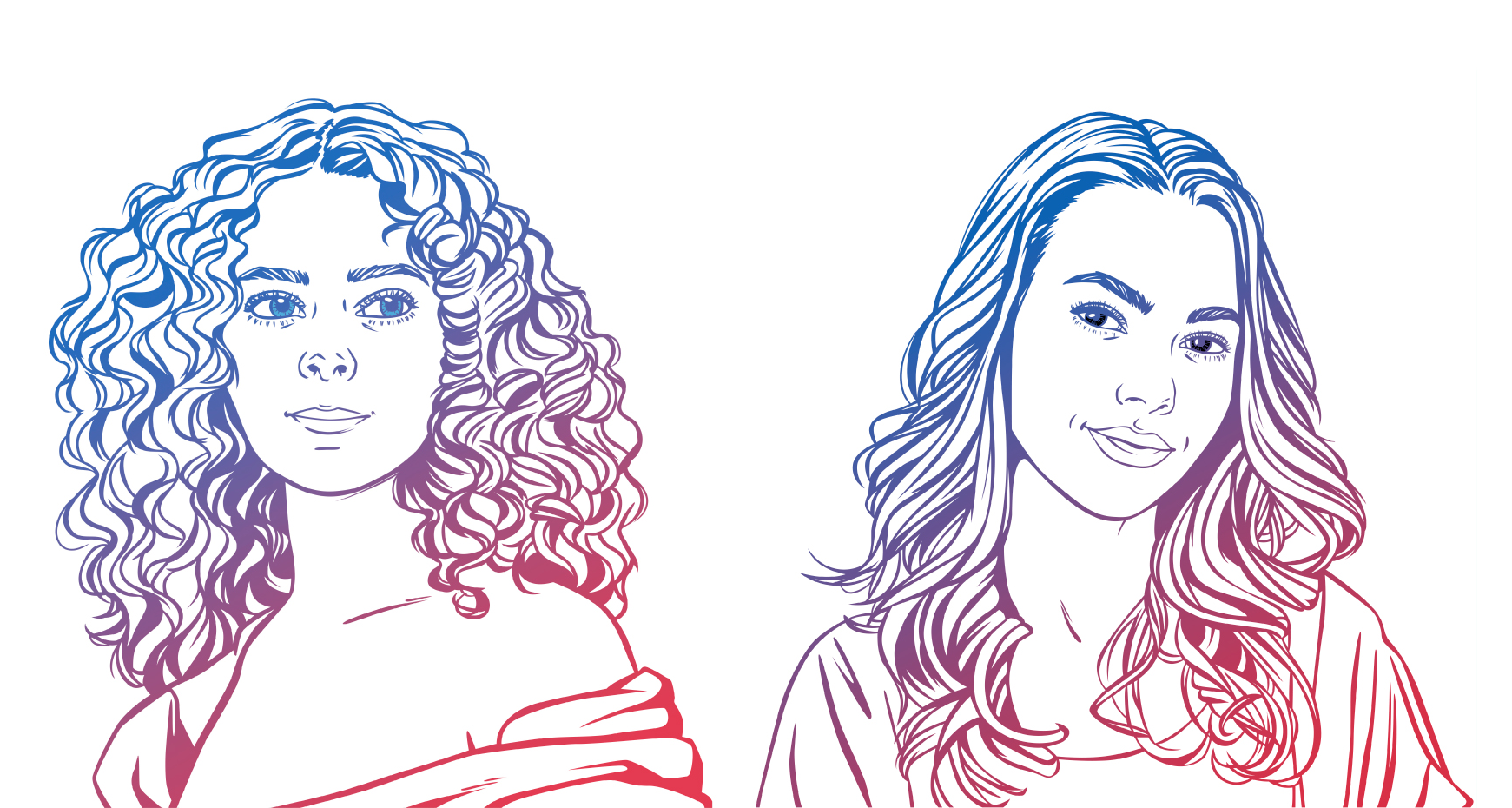
Lady of the 19th century. VS Lady of the 21st century.
It was: a tall and thin blonde with very fair, almost pale skin and huge blue eyes. Most likely, with "scattered on the shoulders" curls. Perhaps she is pampered, wayward and a little moody. Approximately the corresponding modern analogue: a curly brown-eyed lady with long dark hair, plump lips stand out on a tanned face. Maybe she is flirty and relaxed, but at the same time romantic and vulnerable.
The second type. You are already familiar with this picture:
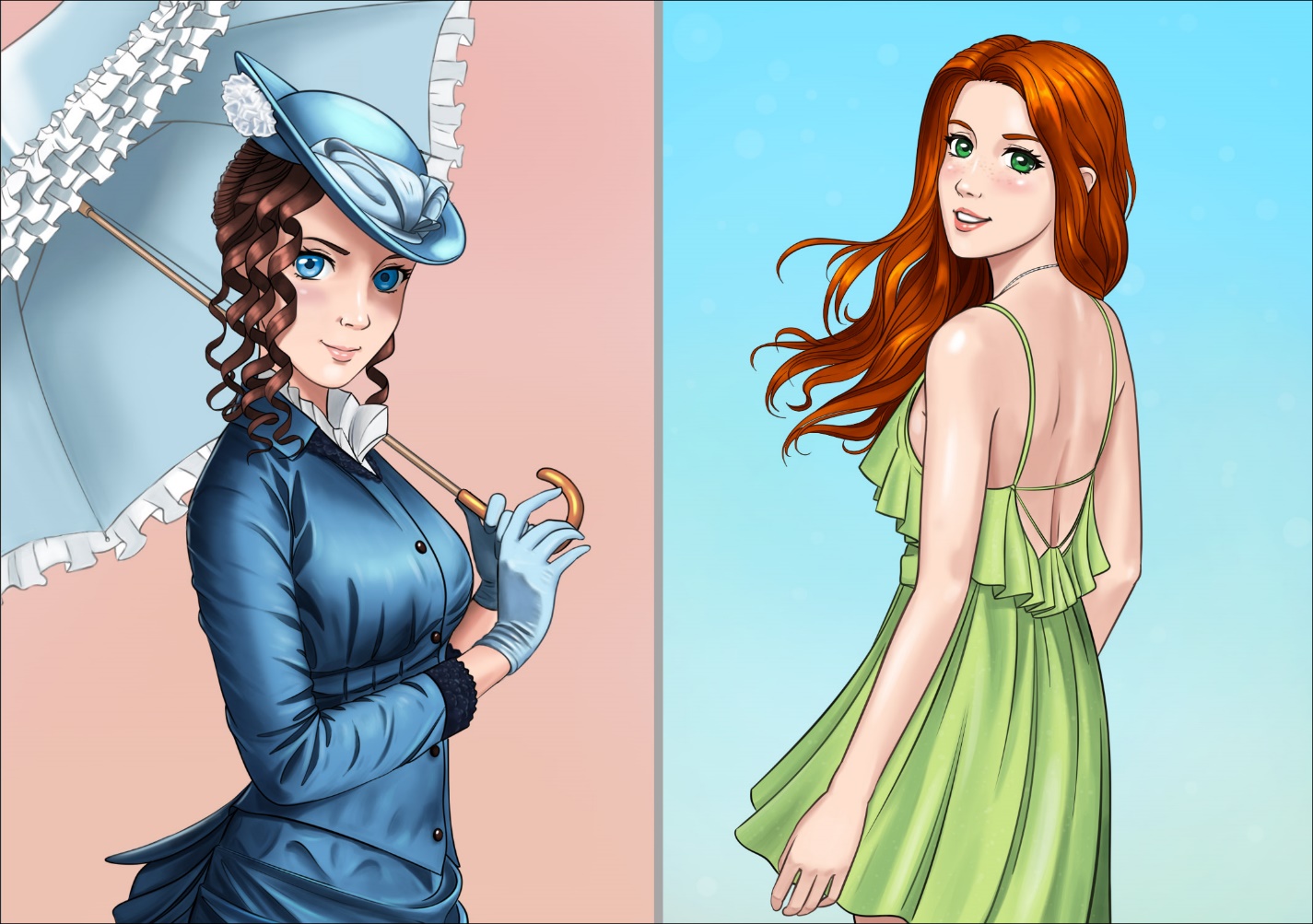
It was: a young tender brunette with blue eyes who smiles warmly. Much attention is paid to neat thin fingers. She is thoughtful, meek, compliant, even shy. Often she looks from behind a curl. The modern beauty will be different. Blue eyes are still a sign of beauty along with black ones, but green eyes appear that previously were not there at all. It turns out a young, green-eyed, red-haired (this is also a completely new sign!) Girl, with good makeup, she is also slim, tall, wears a light, light dress. She is optimistic, calm and smart.
Visualizations are more likely to show differences: artists see my arrays of parameters in this way. Fantasies of character also emerged from the frequency epithets found in the extracted fragments.
Why is this all?
Just practice between projects. In the same way, I can look for signs of trade secrets in your correspondence, even if you describe it in a very veiled way. In the same way, I can monitor the news to look for specific events or events related to your company. In the same way, I can monitor brand mentions and divide them into categories by department, tonality and reason for contact. I can parse applications for technical support from very inadequate users. I can analyze in which city which dialogues are being conducted. I can set the platform on all your payments from the inside of the bank and for all the bank’s counterparties make a list of manufactured products, a list of supplied products and understand what is interesting to the manager. In general, fear me!
Well, or I can just see anything in the texts. Analyze descriptions of houses and interiors. Find side effects on the medicine. Find out that the waffles are crunching somehow wrong, and the sugar in the cookies is not sweet enough. Find out that blondes are still almost twice as popular as brunettes, and blue eyes do not go out of style. And so on…
And here’s the practical application: how we looked for signs of medical errors .