- 1:
- 2: Manifold learning (latent)
- 3: (VAE)
- 4: Conditional VAE
- 5: GAN (Generative Adversarial Networks) tensorflow
- 6: VAE + GAN
(-、、 。。)
VAE、、 :-、 、(、)。
、 - GAN「。
GAN', , , , . .
GAN
GAN’ [1, Generative Adversarial Nets, Goodfellow et al, 2014] . state-of-the-art adversarial.
GAN:
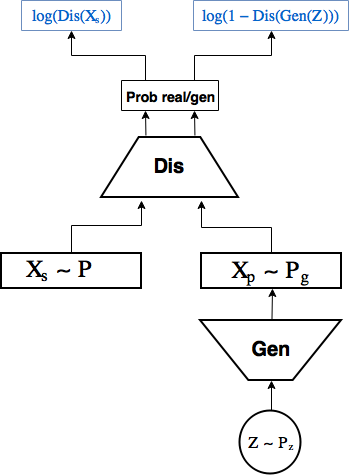
GAN’ 2 :
- 1- — -
,
, ,
- 2番目-弁別
器はサンプルから入力を受け取り、ジェネレーターによって作成され、
特定のオブジェクトが実在する確率を予測することを学習し、スカラーを発行し
ます。
この場合、ジェネレーターは、識別器が実際のオブジェクトと区別しないオブジェクトを作成するようにトレーニングします。GAN
学習プロセスを検討してください。
ジェネレーターとディスクリミネーターは別々にトレーニングされますが、同じネットワーク内にあります。
弁別器トレーニングのkステップを実行します。弁別器トレーニングステップでは、
次はジェネレーターのトレーニングステップです
トレーニングスキーム:
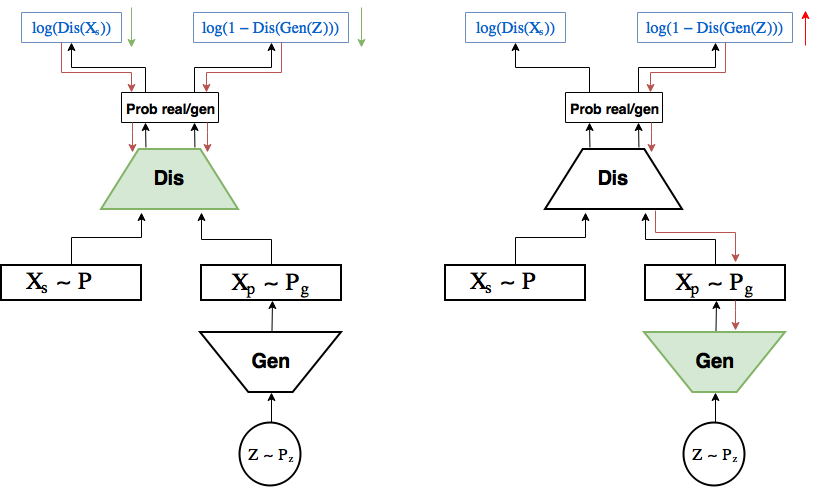
左の写真の弁別器のトレーニングステップ:勾配(赤い矢印)は損失から弁別器のみに流れ、損失
解決するタスクは次のように定式化されます。
与えられたジェネレーターで、最適な識別器はほとんど明白な確率を与えます。ちょっと考えてみることをお勧めします。
[1]では、両方のネットワークの十分なパワーで、この問題は、ジェネレーターが分布を生成することを学習した最適条件を持っていることが示されています

[1]からの図
指定:
- 黒い点線曲線-真の分布
、
-
、
- 青-確率分布
弁別器は、実際のオブジェクトのクラスを予測します。
- 下と上の直線はすべての集合です
そして多くのすべて
、矢印は表示を表します
。
写真で:
- (a)
そして
まったく異なりますが、識別者は一方を他方と不確かに区別します。
-
- (c)これにより、ジェネレーター
良好な弁別器勾配によって導かれる
、2つの分布の境界線上を移動する
に近い
、
- (d)ステップ(a)、(b)、(c)を何度も繰り返した結果
、そして弁別器はもう一方を区別することができません:
。 最適なポイントに到達しました。
条件付きGAN
最後の部分で条件付きVAEを行ったように、ラベルをエンコーダー番号とデコーダー番号に渡すだけで、ここでジェネレーターとディスクリミネーターに渡します[2]
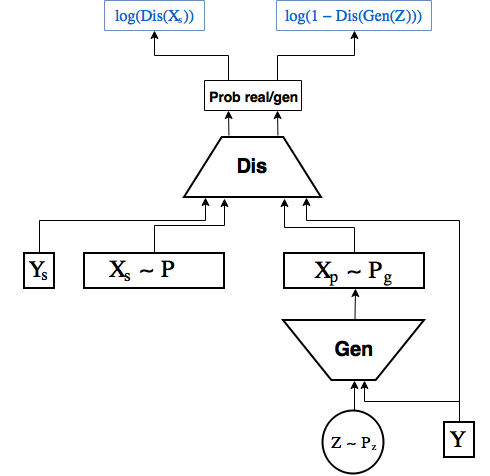
コード
1つのケラで管理することが可能であった以前の部分とは異なり、ここに問題があります。 つまり、同じネットワーク内でジェネレーターのパラメーターのみ、またはディスクリミネーターのみを更新する必要があります。 工夫すれば、純粋にkerasで行うことができますが、私にとってはここにテンソルフローを接続する方が簡単で便利です。
kerasブログには、これを行う方法に関する小さなチュートリアル [3]があります。
kerasの利点は、簡単にtensorflowと組み合わされます-tensorflow.contribに追加された何も理由ではありません。
必要なモジュールをインポートし、データセットをロードすることから始めましょう。
from IPython.display import clear_output
import numpy as np
import matplotlib.pyplot as plt
%matplotlib inline
from keras.layers import Dropout, BatchNormalization, Reshape, Flatten, RepeatVector
from keras.layers import Lambda, Dense, Input, Conv2D, MaxPool2D, UpSampling2D, concatenate
from keras.layers.advanced_activations import LeakyReLU
from keras.models import Model, load_model
from keras.datasets import mnist
from keras.utils import to_categorical
(x_train, y_train), (x_test, y_test) = mnist.load_data()
x_train = x_train.astype('float32') / 255.
x_test = x_test .astype('float32') / 255.
x_train = np.reshape(x_train, (len(x_train), 28, 28, 1))
x_test = np.reshape(x_test, (len(x_test), 28, 28, 1))
y_train_cat = to_categorical(y_train).astype(np.float32)
y_test_cat = to_categorical(y_test).astype(np.float32)
で動作するようにkerasとtensorflowそれを登録する必要があると同時にtensorflowセッションkerasを、それがする必要があるkerasセッションの枠組みの中で使用されるすべての内部変数を作成します。
from keras import backend as K
import tensorflow as tf
sess = tf.Session()
K.set_session(sess)
batch_size = 256
batch_shape = (batch_size, 28, 28, 1)
latent_dim = 2
num_classes = 10
dropout_rate = 0.3
.fit メソッドを使用してモデルをトレーニングするのではなく、tensorflowから直接トレーニングするため、次のバッチを返すイテレーターを作成します。
def gen_batch(x, y):
n_batches = x.shape[0] // batch_size
while(True):
for i in range(n_batches):
yield x[batch_size*i: batch_size*(i+1)], y[batch_size*i: batch_size*(i+1)]
idxs = np.random.permutation(y.shape[0])
x = x[idxs]
y = y[idxs]
train_batches_it = gen_batch(x_train, y_train_cat)
test_batches_it = gen_batch(x_test, y_test_cat)
ケラスモデルの入力レイヤーの写真、ラベル、隠し変数 のプレースホルダーをラップします:
x_ = tf.placeholder(tf.float32, shape=(None, 28, 28, 1), name='image')
y_ = tf.placeholder(tf.float32, shape=(None, num_classes), name='labels')
z_ = tf.placeholder(tf.float32, shape=(None, latent_dim), name='z')
img = Input(tensor=x_)
lbl = Input(tensor=y_)
z = Input(tensor=z_)
CGAN, .
. Keras scope’, , -
with tf.variable_scope('generator'):
x = concatenate([z, lbl])
x = Dense(7*7*64, activation='relu')(x)
x = Dropout(dropout_rate)(x)
x = Reshape((7, 7, 64))(x)
x = UpSampling2D(size=(2, 2))(x)
x = Conv2D(64, kernel_size=(5, 5), activation='relu', padding='same')(x)
x = Dropout(dropout_rate)(x)
x = Conv2D(32, kernel_size=(3, 3), activation='relu', padding='same')(x)
x = Dropout(dropout_rate)(x)
x = UpSampling2D(size=(2, 2))(x)
generated = Conv2D(1, kernel_size=(5, 5), activation='sigmoid', padding='same')(x)
generator = Model([z, lbl], generated, name='generator')
def add_units_to_conv2d(conv2, units):
dim1 = int(conv2.shape[1])
dim2 = int(conv2.shape[2])
dimc = int(units.shape[1])
repeat_n = dim1*dim2
units_repeat = RepeatVector(repeat_n)(lbl)
units_repeat = Reshape((dim1, dim2, dimc))(units_repeat)
return concatenate([conv2, units_repeat])
with tf.variable_scope('discrim'):
x = Conv2D(128, kernel_size=(7, 7), strides=(2, 2), padding='same')(img)
x = add_units_to_conv2d(x, lbl)
x = LeakyReLU()(x)
x = Dropout(dropout_rate)(x)
x = MaxPool2D((2, 2), padding='same')(x)
l = Conv2D(128, kernel_size=(3, 3), padding='same')(x)
x = LeakyReLU()(l)
x = Dropout(dropout_rate)(x)
h = Flatten()(x)
d = Dense(1, activation='sigmoid')(h)
discrim = Model([img, lbl], d, name='Discriminator')
, placeholder’ tensorflow .
generated_z = generator([z, lbl])
discr_img = discrim([img, lbl])
discr_gen_z = discrim([generated_z, lbl])
gan_model = Model([z, lbl], discr_gen_z, name='GAN')
gan = gan_model([z, lbl])
log_dis_img = tf.reduce_mean(-tf.log(discr_img + 1e-10))
log_dis_gen_z = tf.reduce_mean(-tf.log(1. - discr_gen_z + 1e-10))
L_gen = -log_dis_gen_z
L_dis = 0.5*(log_dis_gen_z + log_dis_img)
通常、tensorflowでは、損失をオプティマイザーに渡し、依存するすべての変数をすぐに最小化しようとします。これは今は必要ありません。ジェネレーターをトレーニングするとき、エラーはディスクリミネーターに触れてはなりません。
これを行うには、オプティマイザーに加えて、最適化する変数のリストを転送する必要があります。tf.get_collectionを使用して、目的のスコープからこれらの変数を取得します
optimizer_gen = tf.train.RMSPropOptimizer(0.0003)
optimizer_dis = tf.train.RMSPropOptimizer(0.0001)
# ()
generator_vars = tf.get_collection(tf.GraphKeys.TRAINABLE_VARIABLES, "generator")
discrim_vars = tf.get_collection(tf.GraphKeys.TRAINABLE_VARIABLES, "discrim")
step_gen = optimizer_gen.minimize(L_gen, var_list=generator_vars)
step_dis = optimizer_dis.minimize(L_dis, var_list=discrim_vars)
sess.run(tf.global_variables_initializer())
#
def step(image, label, zp):
l_dis, _ = sess.run([L_dis, step_gen], feed_dict={z:zp, lbl:label, img:image, K.learning_phase():1})
return l_dis
#
def step_d(image, label, zp):
l_dis, _ = sess.run([L_dis, step_dis], feed_dict={z:zp, lbl:label, img:image, K.learning_phase():1})
return l_dis
# , ,
figs = [[] for x in range(num_classes)]
periods = []
save_periods = list(range(100)) + list(range(100, 1000, 10))
n = 15 # 15x15
from scipy.stats import norm
# N(0, I), , ,
grid_x = norm.ppf(np.linspace(0.05, 0.95, n))
grid_y = norm.ppf(np.linspace(0.05, 0.95, n))
grid_y = norm.ppf(np.linspace(0.05, 0.95, n))
def draw_manifold(label, show=True):
#
figure = np.zeros((28 * n, 28 * n))
input_lbl = np.zeros((1, 10))
input_lbl[0, label] = 1.
for i, yi in enumerate(grid_x):
for j, xi in enumerate(grid_y):
z_sample = np.zeros((1, latent_dim))
z_sample[:, :2] = np.array([[xi, yi]])
x_generated = sess.run(generated_z, feed_dict={z:z_sample, lbl:input_lbl, K.learning_phase():0})
digit = x_generated[0].squeeze()
figure[i * 28: (i + 1) * 28,
j * 28: (j + 1) * 28] = digit
if show:
#
plt.figure(figsize=(10, 10))
plt.imshow(figure, cmap='Greys')
plt.grid(False)
ax = plt.gca()
ax.get_xaxis().set_visible(False)
ax.get_yaxis().set_visible(False)
plt.show()
return figure
n_compare = 10
def on_n_period(period):
clear_output() # output
# y
draw_lbl = np.random.randint(0, num_classes)
print(draw_lbl)
for label in range(num_classes):
figs[label].append(draw_manifold(label, show=label==draw_lbl))
periods.append(period)
CGANを使用してください。
、、。、
、、、。
batches_per_period = 20 #
k_step = 5 # ,
for i in range(5000):
print('.', end='')
#
b0, b1 = next(train_batches_it)
zp = np.random.randn(batch_size, latent_dim)
#
for j in range(k_step):
l_d = step_d(b0, b1, zp)
b0, b1 = next(train_batches_it)
zp = np.random.randn(batch_size, latent_dim)
if l_d < 1.0:
break
#
for j in range(k_step):
l_d = step(b0, b1, zp)
if l_d > 0.4:
break
b0, b1 = next(train_batches_it)
zp = np.random.randn(batch_size, latent_dim)
#
if not i % batches_per_period:
period = i // batches_per_period
if period in save_periods:
on_n_period(period)
print(l_d)
from matplotlib.animation import FuncAnimation
from matplotlib import cm
import matplotlib
def make_2d_figs_gif(figs, periods, c, fname, fig, batches_per_period):
norm = matplotlib.colors.Normalize(vmin=0, vmax=1, clip=False)
im = plt.imshow(np.zeros((28,28)), cmap='Greys', norm=norm)
plt.grid(None)
plt.title("Label: {}\nBatch: {}".format(c, 0))
def update(i):
im.set_array(figs[i])
im.axes.set_title("Label: {}\nBatch: {}".format(c, periods[i]*batches_per_period))
im.axes.get_xaxis().set_visible(False)
im.axes.get_yaxis().set_visible(False)
return im
anim = FuncAnimation(fig, update, frames=range(len(figs)), interval=100)
anim.save(fname, dpi=80, writer='imagemagick')
for label in range(num_classes):
make_2d_figs_gif(figs[label], periods, label, "./figs4_5/manifold_{}.gif".format(label), plt.figure(figsize=(10,10)), batches_per_period)
:
ガン
GAN() 、、 VAE()

CGAN

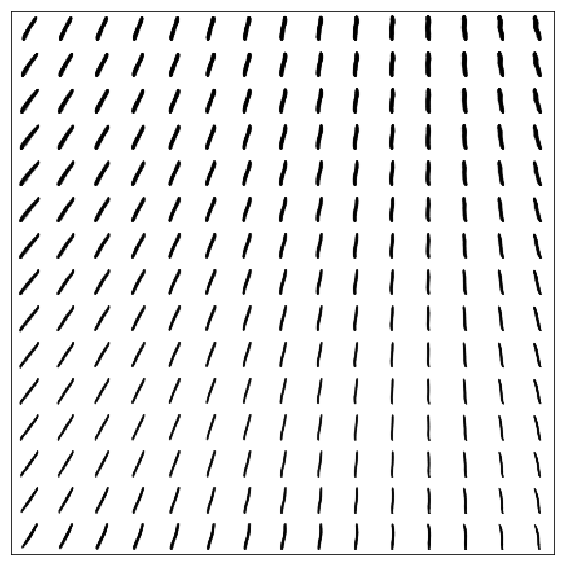





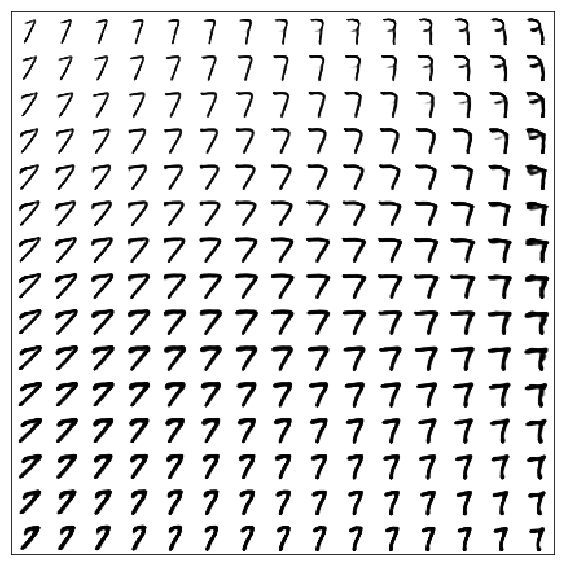


:
[1] Generative Adversarial Nets、Goodfellow et al、2014、https
://arxiv.org/abs/1406.2661 Conditional GANs:
[2] Conditional Generative Adversarial Nets、Mirza、Osindero、2014、https:// arxiv。 ORG / ABS / 1411.1784
keras tensorflow:
[3] https://blog.keras.io/keras-as-a-simplified-interface-to-tensorflow-tutorial.html