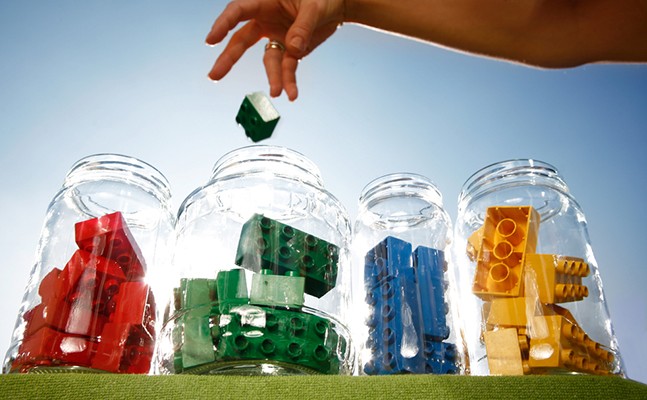
原則として、キャッシュに関する記事は正常性のために始まり、 LRUキャッシュで終わります。 この傾向を逆転させようか? そもそも、LRUが悪いのは何なのか、そして最終的には健康のためです。 そう願っています。
数百万人の訪問者向けの高負荷サービスを構築するか、モバイルアプリケーションを設計するかに関係なく、オペレーティングシステムまたはDBMSを記述します。システムの最終コストとインターフェース/サービスの応答性に影響する重要なリンクはキャッシュです。
インタビューで知っているキャッシングアルゴリズムの種類を尋ねると、通常は答えが聞こえます。mmm... LRUキャッシュ...しかし、アルゴリズムの並べ替えについて尋ねると、「バブル」以外の何かを聞く可能性がはるかに高くなります。 画像やWebフレームワークのサイズを変更するための最適なライブラリを検索するのに数日費やす準備ができていますが、効果的なキャッシュを実装していることに気づかずに、原則として、類似した特性を持つライブラリを使用できます。
Relap.ioの場合、高負荷サービスに関しては、キャッシングが特に重要です。 たとえば、昨日、さまざまなサイトで789301033回の推奨事項を示しました。 そのため、推奨事項、写真、広告など、すべてがキャッシュで密にカバーされています。
すべてのキャッシュが同じように役立つわけではありません。
LRUキャッシュの良い例。
通常、アルゴリズムは彼を競技会に連れて行きません。 敗者とは何の関係もありません。 より非効率的なアルゴリズムを考案することは困難です。 LRUキャッシュがパフォーマンスの点で優れている唯一のアルゴリズムは、おそらくFIFO(FIFOなど)だけです。 それでも、LRUはデフォルトとしてどこにでも、そして残念ながら、多くの場合唯一のアルゴリズムとして構築されます。これは実装が簡単だからです。
速度が遅く、非効率的であり、リソースを使い果たしていないかのように消費するWebサイト、アプリケーション、またはオペレーティングシステムを使用しますか?ただし、条件付き基本などの実装しやすい言語で記述されていますか? そうでない場合は、猫へようこそ。
パレート規則が大好きです。 統計的に重要なサンプルでは、絶対にすべてに適用できます。
試してみましょう:
- 努力の20%が結果の80%をもたらし、
- 商品の20%が利益の80%をもたらし、
- ビューの80%はURLの20%を占め、
- コードの20%が機能の80%を実装しています。
これは、大規模なデータセットに有効な、かなり興味深いパターンです。 パレートはどこですか?
<歌詞の余談>
数年前、SurfingbirdのAndroidアプリケーションを作成しました。 RX Javaに切り替えました。 可能なすべての非同期。 すべてのトランジションはアニメーションでスムーズにされましたが、1つの未解決の問題が残っていました。これらは絶え間ない画像のリロードでした。 そして、あなたのアプリケーションは文字通り写真でいっぱいであり、それらは絶えず回転し、変化し、あなたはそれらを置くのに十分なメモリを持っていません。
最初は、すべてがimagloaderにあると思いました。 効果的で出来上がりを選択するだけで十分です。 すべてを見直しました。 ピカソ、Facebookのフレスコ画、UILすべての名前を覚えているわけではありません。 しかし、問題は残った。 写真はどこか少し速く、どこか少し滑らかにロードされましたが、ロードされました。 それから私は座って私に書いた 。 シンプル。 きれい。 軽量。 そして、それは助けにはなりませんでした。 愚かな成虫ローダーは、ユーザーを不安にさせるイメージを常にいじり続け、穀物をもみ殻から分離できませんでした。 その後、パレート規則を思い出しました。
</叙情的な余談>
画像の20%-時間の80%を示していると仮定すると、すべてが適切に配置されます。 理解しておくべきことは、どの写真を保存する必要があるかだけです。
LRUキャッシュはどのように機能しますか?
真空中の球状のアプリケーションを見てみましょう。 それをメッセンジャーにしましょう、想像するのが最も簡単です。
telegram_ screenshot.jpg
スクリーンショットを注意深く見ると、ユーザーメッセージにアバターが付いており、メッセージ本文に写真があることがわかります。 あなたの仕事は、インターフェースを可能な限り滑らかにすることです。 上のスクリーンショットをもう一度見てみましょう。 ダイアログに2つの繰り返しの秋が表示され、ユーザー1が大きな画像を送信しました。
- 1-100 x 100ピクセルのアバターが来たので、100 * 100 * 4バイトをキャッシュに書き込みました。
- 2-100 x 100ピクセルのアバターが来たので、100 * 100 * 4バイトをキャッシュに書き込みました。
- アバター1が来ました-ラインを持ち上げました。
これまでのところ、とても良い。
画像は1024 x 768ピクセルで、キャッシュに1024 * 768 * 4バイトを書き込みました-そしてBAM! 私たちの美しいアバターはキャッシュから完全にノックアウトされました。 今では、一度表示する必要があり、キャッシュする必要のない写真が厳liesにあります。
勝つ方法
たとえば、AQueryライブラリを見ると、開発者はキャッシュをいくつかのヒープに分割することを提案しています。 小さなアバターには別の山、大きな写真には別の山。 ところで、すでにパンですが、このソリューションは普遍的ではなく、プログラミングと注意が必要ですが、すべてを一度に欲しいです。 興味深いものはすべて私たちの前ですでに発明されているので、今度は他のキャッシングアルゴリズムが存在するかどうかを見てみましょう。
ウィキの記事
ここで少し台無しにしたことを許してください。非常に簡潔な真実を説明します。
LRU-最長時間使用されずにキャッシュから飛び出します。
MRU-最後に使用されたものがキャッシュから飛び出します(特定の場合、ジャンクの世話をします)。
LFU-最も頻繁に使用されないクラッシュ。
これがベースです。 計算のコストはアルゴリズムの複雑さとともに増加することを恐れる場合がありますが、重要ではありません。 メモリ内のキーによるルックアップの時間を、画像を1024〜768でレンダリングする時間と比較してみてください。つまり、これは、アルゴリズムが「欠落」した場合に発生します。
SNLRU (セグメント化されたLRU) -LRUでいくつかの「ボックス」を開始します。 最初に、それを最初のボックスに入れ、リクエストを繰り返したときに、2番目の2番目-3番目に転送します。
ボックスに名前を付けると、より明確になります。
- 寒さは最初の箱です
- 暖かい-2番目、
- ホットは3番目です。
これはすでに良いアルゴリズムです。間違えなければfreebsdの腸で使用されます。 少なくとも私はこの文脈で彼に出会いました。
中間点LRU -2つのボックスしかないセグメント化されたLRU。
MQ-記憶されているセグメント化されたLRU。 要素が離陸した位置は記憶されており、繰り返し要求されると、記憶された位置のラインから飛び出なかった場合には元の位置に戻ります。 実際、要素の周期的な回転が発生すると、キャッシュはより速くウォームアップします(うんちが普及する可能性があります)。 かなり奇妙なユーザーケースのように見えます。
ARC、GCLOCK-およびその他のより複雑なアルゴリズムは、しばらく時間を空ける必要があります。 それらが悪いまたは面白くないというわけではなく、同じARCがpostgreSQLで使用されます(より正確には、この痛みを伴う記事www.varlena.com/GeneralBits/96.phpから判断して、おそらく使用されました )。 私は小さな引用に抵抗することはできません:
多くの場合、データベースシステムはLRUアルゴリズムを使用しますが、多くはLRUで適切に処理されないさまざまな動作をより適切に処理するために他のアルゴリズムに切り替えています。 たとえば、1回限りの非常に大きなシーケンシャルスキャンでは、すぐに再び使用されることのないページでキャッシュがあふれることがあります。 キャッシュは、より一般的に使用されるページが再配置されるまで役に立ちません。
2Q-または2つのキュー。実装の容易さを維持しながら、完全に適応することは注目に値します。 キャッシュは、セグメント化されたLRUのように3つの部分に分割されますが、より複雑な戦略があります。
- Inの最初の部分は、新しい要素が配置されるFIFO着信キャッシュです。
- Outの2番目の部分は、FIFOの発信キャッシュで、Inボックスから要素がプッシュされます。
- ホットLRUキャッシュの3番目の部分は、Outから要求されたアイテム用です。
キャッシュワイプ戦略:
- Inからリクエストされたアイテムはどこにも移動しません。 In要素から移動-Outに移動します。
- アウトからリクエストされたアイテム-メインボックスで天国に行きます。 Outから強制的に使用(未使用)-地獄に直行(ヌル)。
正規の説明へのリンク。
まず、美しいです。 メインボックス-たとえば、20%(パレートについて覚えていますか?)アバターが蓄積されるのはここです。 しかし、アウト-あなたはもっと、60パーセントをする必要があります。
Inの魅力-新しい要素は、インバウンドからアウトへのFIFOパイプを穏やかに下降します。バウンスせず、要求に応じてどこにも移動しません。 しかし、彼らが再び要求した場合(たとえば、ユーザーが上にスクロールした場合)、画像がInからOutに切り替わりました-ここが勝者です。 アーキテクチャレベルのキャッシュは、日常生活に存在するいくつかの典型的な相関関係を修正します。 実装後、メモリサイズが限られている状況では、一定の再起動が消えました。 パレートは働いた。 しかし、パレートに何度も戻ります。
まず、ニュアンスに移りましょう。 3つのボックスについて読むと、3つのリンクリストを作成し、それらの周りのアイテムを駆動するだけの愚かな誘惑があります。 しかし、これは非効率的な方法であり、どういうわけかジェダイの方法ではありません。 実際、キーがどのボックスにあるのかを知るだけでよく、その時点で値自体はsomeいヒープにある可能性があります。 むしろプログラミングに移りましょう。
Javaでの実装、bam!
package com.squareup.picasso; import java.util.ArrayList; import java.util.HashMap; import java.util.Iterator; import java.util.LinkedHashSet; import java.util.List; import java.util.Map; /** * 2Q: A Low Overhead High Performance Buffer Management Replacement Algorith * Based on description: http://www.vldb.org/conf/1994/P439.PDF * Created by recoilme on 22/08/15. * email: vadim-kulibaba@yandex.ru */ public class TwoQCache<K, V> { /** * Primary container */ final HashMap<K, V> map; /** * Sets for 2Q algorithm */ private final LinkedHashSet<K> mapIn, mapOut, mapHot; protected float quarter = .25f; /** * Size of this cache in units. Not necessarily the number of elements. */ //private int size; private int sizeIn; private int sizeOut; private int sizeHot; private int maxSizeIn; private int maxSizeOut; private int maxSizeHot; private int putCount; private int createCount; private int evictionCount; private int hitCount; private int missCount; /** * Two queues cache * * @param maxSize for caches that do not override {@link #sizeOf}, this is * this is the maximum sum of the sizes of the entries in this cache. */ public TwoQCache(int maxSize) { if (maxSize <= 0) { throw new IllegalArgumentException("maxSize <= 0"); } calcMaxSizes(maxSize); map = new HashMap<K, V>(0, 0.75f); mapIn = new LinkedHashSet<K>(); mapOut = new LinkedHashSet<K>(); mapHot = new LinkedHashSet<K>(); } /** * Sets sizes: * mapIn ~ 25% // 1st lvl - store for input keys, FIFO * mapOut ~ 50% // 2nd lvl - store for keys goes from input to output, FIFO * mapHot ~ 25% // hot lvl - store for keys goes from output to hot, LRU * * @param maxSize if mapIn/mapOut sizes == 0, works like LRU for mapHot */ private void calcMaxSizes(int maxSize) { if (maxSize <= 0) { throw new IllegalArgumentException("maxSize <= 0"); } synchronized (this) { //sizes maxSizeIn = (int) (maxSize * quarter); maxSizeOut = maxSizeIn * 2; maxSizeHot = maxSize - maxSizeOut - maxSizeIn; } } /** * Sets the size of the cache. * * @param maxSize The new maximum size. */ public void resize(int maxSize) { calcMaxSizes(maxSize); synchronized (this) { HashMap<K, V> copy = new HashMap<K, V>(map); evictAll(); Iterator<K> it = copy.keySet().iterator(); while (it.hasNext()) { K key = it.next(); put(key, copy.get(key)); } } } /** * Returns the value for {@code key} if it exists in the cache or can be * created by {@code #create}. If a value was returned, it is moved to the * head of the queue. This returns null if a value is not cached and cannot * be created. */ public V get(K key) { if (key == null) { throw new NullPointerException("key == null"); } V mapValue; synchronized (this) { mapValue = map.get(key); if (mapValue != null) { hitCount++; if (mapHot.contains(key)) { // add & trim (LRU) mapHot.remove(key); mapHot.add(key); } else { if (mapOut.contains(key)) { mapHot.add(key); sizeHot += safeSizeOf(key, mapValue); trimMapHot(); sizeOut -= safeSizeOf(key, mapValue); mapOut.remove(key); } } return mapValue; } missCount++; } /* * Attempt to create a value. This may take a long time, and the map * may be different when create() returns. If a conflicting value was * added to the map while create() was working, we leave that value in * the map and release the created value. */ V createdValue = create(key); if (createdValue == null) { return null; } synchronized (this) { createCount++; if (!map.containsKey(key)) { // There was no conflict, create return put(key, createdValue); } else { return map.get(key); } } } /** * Caches {@code value} for {@code key}. * * @return the previous value mapped by {@code key}. */ public V put(K key, V value) { if (key == null || value == null) { throw new NullPointerException("key == null || value == null"); } if (map.containsKey(key)) { // if already have - replace it. // Cache size may be overheaded at this moment synchronized (this) { V oldValue = map.get(key); if (mapIn.contains(key)) { sizeIn -= safeSizeOf(key, oldValue); sizeIn += safeSizeOf(key, value); } if (mapOut.contains(key)) { sizeOut -= safeSizeOf(key, oldValue); sizeOut += safeSizeOf(key, value); } if (mapHot.contains(key)) { sizeHot -= safeSizeOf(key, oldValue); sizeHot += safeSizeOf(key, value); } } return map.put(key, value); } V result; synchronized (this) { putCount++; final int sizeOfValue = safeSizeOf(key, value); //if there are free page slots then put value into a free page slot boolean hasFreeSlot = add2slot(key, safeSizeOf(key, value)); if (hasFreeSlot) { // add 2 free slot & exit map.put(key, value); result = value; } else { // no free slot, go to trim mapIn/mapOut if (trimMapIn(sizeOfValue)) { //put X into the reclaimed page slot map.put(key, value); result = value; } else { map.put(key, value); mapHot.add(key); sizeHot += safeSizeOf(key, value); trimMapHot(); result = value; } } } return result; } /** * Remove items by LRU from mapHot */ public void trimMapHot() { while (true) { K key; V value; synchronized (this) { if (sizeHot < 0 || (mapHot.isEmpty() && sizeHot != 0)) { throw new IllegalStateException(getClass().getName() + ".sizeOf() is reporting inconsistent results!"); } if (sizeHot <= maxSizeHot || mapHot.isEmpty()) { break; } // we add new item before, so next return first (LRU) item key = mapHot.iterator().next(); mapHot.remove(key); value = map.get(key); sizeHot -= safeSizeOf(key, value); map.remove(key); evictionCount++; } entryRemoved(true, key, value, null); } } /** * Remove items by FIFO from mapIn & mapOut * * @param sizeOfValue size of * @return boolean is trim */ private boolean trimMapIn(final int sizeOfValue) { boolean result = false; if (maxSizeIn < sizeOfValue) { return result; } else { while (mapIn.iterator().hasNext()) { K keyIn; V valueIn; if (!mapIn.iterator().hasNext()) { System.out.print("err"); } keyIn = mapIn.iterator().next(); valueIn = map.get(keyIn); if ((sizeIn + sizeOfValue) <= maxSizeIn || mapIn.isEmpty()) { //put X into the reclaimed page slot if (keyIn == null) { System.out.print("err"); } mapIn.add(keyIn); sizeIn += sizeOfValue; result = true; break; } //page out the tail of mapIn, call it Y mapIn.remove(keyIn); final int removedItemSize = safeSizeOf(keyIn, valueIn); sizeIn -= removedItemSize; // add identifier of Y to the head of mapOut while (mapOut.iterator().hasNext()) { K keyOut; V valueOut; if ((sizeOut + removedItemSize) <= maxSizeOut || mapOut.isEmpty()) { // put Y into the reclaimed page slot mapOut.add(keyIn); sizeOut += removedItemSize; break; } //remove identifier of Z from the tail of mapOut keyOut = mapOut.iterator().next(); mapOut.remove(keyOut); valueOut = map.get(keyOut); sizeOut -= safeSizeOf(keyOut, valueOut); } } } return result; } /** * Check for free slot in any container and add if exists * * @param key key * @param sizeOfValue size * @return true if key added */ private boolean add2slot(final K key, final int sizeOfValue) { boolean hasFreeSlot = false; if (!hasFreeSlot && maxSizeIn >= sizeIn + sizeOfValue) { mapIn.add(key); sizeIn += sizeOfValue; hasFreeSlot = true; } if (!hasFreeSlot && maxSizeOut >= sizeOut + sizeOfValue) { mapOut.add(key); sizeOut += sizeOfValue; hasFreeSlot = true; } if (!hasFreeSlot && maxSizeHot >= sizeHot + sizeOfValue) { mapHot.add(key); sizeHot += sizeOfValue; hasFreeSlot = true; } return hasFreeSlot; } /** * Removes the entry for {@code key} if it exists. * * @return the previous value mapped by {@code key}. */ public V remove(K key) { if (key == null) { throw new NullPointerException("key == null"); } V previous; synchronized (this) { previous = map.remove(key); if (previous != null) { if (mapIn.contains(key)) { sizeIn -= safeSizeOf(key, previous); mapIn.remove(key); } if (mapOut.contains(key)) { sizeOut -= safeSizeOf(key, previous); mapOut.remove(key); } if (mapHot.contains(key)) { sizeHot -= safeSizeOf(key, previous); mapHot.remove(key); } } } if (previous != null) { entryRemoved(false, key, previous, null); } return previous; } /** * Called for entries that have been evicted or removed. This method is * invoked when a value is evicted to make space, removed by a call to * {@link #remove}, or replaced by a call to {@link #put}. The default * implementation does nothing. * <p> * <p>The method is called without synchronization: other threads may * access the cache while this method is executing. * * @param evicted true if the entry is being removed to make space, false * if the removal was caused by a {@link #put} or {@link #remove}. * @param newValue the new value for {@code key}, if it exists. If non-null, * this removal was caused by a {@link #put}. Otherwise it was caused by * an eviction or a {@link #remove}. */ protected void entryRemoved(boolean evicted, K key, V oldValue, V newValue) { } /** * Called after a cache miss to compute a value for the corresponding key. * Returns the computed value or null if no value can be computed. The * default implementation returns null. * <p> * <p>The method is called without synchronization: other threads may * access the cache while this method is executing. * <p> * <p>If a value for {@code key} exists in the cache when this method * returns, the created value will be released with {@link #entryRemoved} * and discarded. This can occur when multiple threads request the same key * at the same time (causing multiple values to be created), or when one * thread calls {@link #put} while another is creating a value for the same * key. */ protected V create(K key) { return null; } private int safeSizeOf(K key, V value) { int result = sizeOf(key, value); if (result < 0) { throw new IllegalStateException("Negative size: " + key + "=" + value); } return result; } /** * Returns the size of the entry for {@code key} and {@code value} in * user-defined units. The default implementation returns 1 so that size * is the number of entries and max size is the maximum number of entries. * <p> * <p>An entry's size must not change while it is in the cache. */ protected int sizeOf(K key, V value) { return 1; } /** * Clear the cache, calling {@link #entryRemoved} on each removed entry. */ public synchronized final void evictAll() { Iterator<K> it = map.keySet().iterator(); while (it.hasNext()) { K key = it.next(); it.remove(); remove(key); } mapIn.clear(); mapOut.clear(); mapHot.clear(); sizeIn = 0; sizeOut = 0; sizeHot = 0; } /** * For caches that do not override {@link #sizeOf}, this returns the number * of entries in the cache. For all other caches, this returns the sum of * the sizes of the entries in this cache. */ public synchronized final int size() { return sizeIn + sizeOut + sizeHot; } /** * For caches that do not override {@link #sizeOf}, this returns the maximum * number of entries in the cache. For all other caches, this returns the * maximum sum of the sizes of the entries in this cache. */ public synchronized final int maxSize() { return maxSizeIn + maxSizeOut + maxSizeHot; } /** * Returns the number of times {@link #get} returned a value that was * already present in the cache. */ public synchronized final int hitCount() { return hitCount; } /** * Returns the number of times {@link #get} returned null or required a new * value to be created. */ public synchronized final int missCount() { return missCount; } /** * Returns the number of times {@link #create(Object)} returned a value. */ public synchronized final int createCount() { return createCount; } /** * Returns the number of times {@link #put} was called. */ public synchronized final int putCount() { return putCount; } /** * Returns the number of values that have been evicted. */ public synchronized final int evictionCount() { return evictionCount; } /** * Returns a copy of the current contents of the cache, ordered from least * recently accessed to most recently accessed. */ public synchronized final Map<K, V> snapshot() { return new HashMap<K, V>(map); } @Override public synchronized final String toString() { int accesses = hitCount + missCount; int hitPercent = accesses != 0 ? (100 * hitCount / accesses) : 0; return String.format("Cache[size=%d,maxSize=%d,hits=%d,misses=%d,hitRate=%d%%," + "]", size(), maxSize(), hitCount, missCount, hitPercent) + "\n map:" + map.toString(); } public List<Object> getMapHotSnapshot() { List<Object> result = new ArrayList<Object>(); Iterator<K> it = mapHot.iterator(); while (it.hasNext()) { K key = it.next(); result.add(key); result.add(map.get(key)); } return result; } }
コンテナに注意してください:
/** Primary container */ private final HashMap<K,V> map; /** Sets for 2Q algorithm */ private final LinkedHashSet<K> mapIn, mapOut, mapHot;
ヒープ管理は、超高速で経済的なLinkedHashSetメモリに実装されます。値は重要ではありません。どのヒープが現在どのキーにあるかだけが重要です。 これがスピードの鍵です。
もっと練習。 画像ローダーにねじ込みたいとしましょう-ピカソへのプールリクエスト: github.com/square/picasso/pull/1134
ただし、通常は必要ありません。 通常のライブラリ-任意のキャッシングアルゴリズムを接続できます- クラスをコピーして貼り付け、キャッシュを再定義するだけです(グライドは、あるバージョンからピカソを知っているようです)
私の場合、ヒット率の正確な数値はもう覚えていません。 LRUのヒット率は70%以上80未満だったことを覚えています。2Qのヒット率は80%強でした。 しかし、奇跡が起こりました。 必要なのは、トラフィックの80%を占める情報の20%をキャッシュすることだけだからです。 ちなみに、速度の点では2QがLRUよりも速いという奇跡がありました。
Relap.ioには、たとえばmine-github.com/recoilme/2qcacheなど、いくつかのキャッシュの実装があります (一般的に、私は真珠のプログラマーではありません。これはこの言語で最初であり、できれば唯一のプラスであり、唯一のプラスです)。
したがって、私たちの主任開発者から真珠への2Qの実装を見ることをお勧めします。
パールの実装、bam: github.com/grinya007/2q
だから、あなたが書くものは何でも構いません:サイト、ハイローダーサービス、モバイルアプリケーション、オペレーティングシステム、一度巧妙なキャッシングアルゴリズムを実装すると、どこでも使用でき、ユーザーリソースと神経を節約できます。