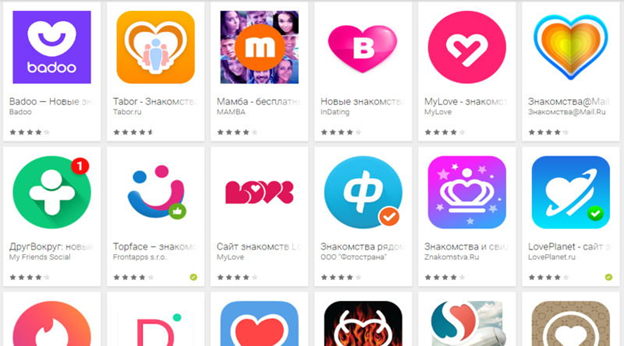
Examples of dating applications
If you do not touch on a large number of fraud, crooks, spammers in this segment, then in fact on sites or in mobile applications people are looking for a couple and it is desirable that their interest in each other be mutual. Everything that does not meet this criterion, in fact, is a waste of time on one side. The most established approach from the point of view of information submission is โleafletsโ, where one profile is displayed and the viewer must decide whether he likes it or not (like, dislike). If she likes the questionnaire, she will be notified and if the interest in each other is mutual, then it becomes possible to start a dialogue. Deviations from this scheme are possible in various applications and on different sites, but the general principle of operation should be clear.
Like, introduced many years ago by Facebook, turned out to be a very powerful motivating and convenient tool. He allows without dialogue to show his interest in someone and, depending on his reaction, not to wait for an answer, but to continue searching. If there is a reaction, a dialogue may begin, but its value is much higher than just scattered "greetings" without taking into account reciprocity.
Yes, likes helped, and the use of mutual sympathy, too, but nevertheless, in the dating market, little has changed over the past 15 years, and this is strange for the IT industry, in which online dating is developing, too.
What, in fact, is the problem?
On average, according to statistics, there are 17 likes per 100 dislikes. That is, a person does a lot more useless work than useful and receives content that is not of interest to him. This is taking into account the current parameters for filtering the results (search by city, age, interests and other characteristics specified in the profile). It turns out that the personโs primary action in relation to the questionnaire is not related to the awareness of the text information that the owner of the questionnaire filled out about himself, but is connected exclusively with the perception of the photograph presented (the whole or the person in particular). Further evaluation of the opponent will be made already at the next step, when mutual sympathy is formed.
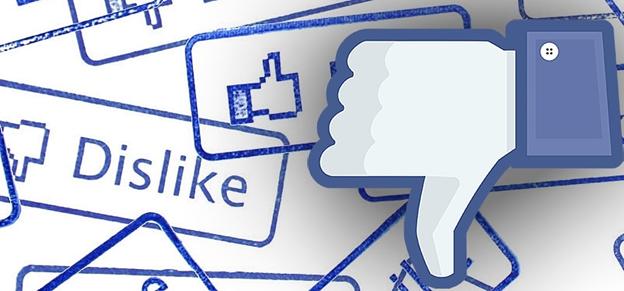
Likes, dislikes - what's the problem?
The ultimate goal is to reduce the number of useless actions of users and make a selection of profiles in a better quality dating than at the current stage. Having a sufficiently large base for building statistics, analyzing and testing hypotheses, as well as for training and using neural networks, we made and tested the following two assumptions:
- But what if all people are similar and by analyzing the results of similar people on the choice of questionnaires, can you improve and influence the quality of this sample?
- Can a neural network from photographs predict our preferences based on previous experience?

Neural networks in dating
Below we will describe in more detail about our experiments using the example of data from one of the mobile applications in the field of dating. Thanks to the colleagues who gave their consent to such experiments, analytics and the introduction of new technologies. Of course, all the changes made and the experiments carried out in no way violate the Federal Law on personal data and GDPR.
Solution 1. What if all people are alike?
The assumption and hypothesis are as follows: if one person likes someone taking into account basic search filters, such as a city, age range and gender, then the results (choice) of another who like the same person will be more likely to fit the first one and take into account repeating hypotheses and increasing the number of people, the quality of the sample will improve.
An extension of this hypothesis is the minimization of incoming filtering parameters by sex and city, as well as taking into account not only outgoing likes of the people you are looking for with similar preferences, but also taking into account the responses to them of the profiles they like.
Hypothesis test. Only the gender and city are specified in the filter. Sampling results with dislikes at the beginning:
- The first four profiles that do not like
- First two likes on suitable profiles
- Another dislike
- And at the fourth iteration, getting a sample of 5 suitable profiles according to specified criteria
Based on the experiment, the process of automatically generating a search query based on the opinions of other people, based on which the possible preferences of the active participant are formed, is demonstrated.
As an extension of this scheme, it is possible to consider taking dislikes together with likes to clarify the portrait of a person, as well as age and other characteristics of profiles that fall into the sample.
Used algorithm settings when testing a hypothesis:

Option 2. And let's learn the neural network to make a decision for us
The assumption and hypothesis are as follows: perhaps the person in the photo plays a more significant role in the choice of people than the general background, location, accessories, shooting quality and other factors. To test the hypothesis, we used a detector on Intel OpenVINO for 1 million photos. The results were obtained faces from the general photos of users with the parallel availability of a database of likes (who liked whom and where these likes are mutual). The result of the detector and the imposition of key points of the face on the original image:
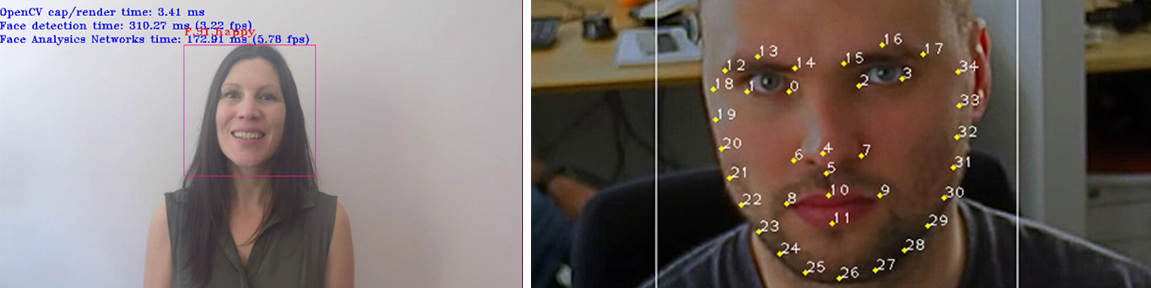
Further, pairs of photographs in various variations were transmitted to neural networks during the training process:
- The first photo (profile) like the second
- The first photo (profile) like the second, and the second first
- The first photo (profile) does not like the second, and the second does not like the first
The training sample when comparing on the basis of likes on the database is shown below. Two inputs in the form of photos that are transmitted to the network only if there is an incoming like from the first to the second, mutual like or when there are no mutual likes (for three different networks with their sequential training).
The result was three neural networks that, with a given degree of probability, can determine the compatibility of people in photographs. The advantage of this option over the first is the high speed of searching for preferences in photographs and the minimum number of queries and dependencies at the level of the SQL query. In other words, the resulting solution is highly productive with high speed and low consumption and resource requirements in the inference process (not to be confused with the learning process, where maximum performance of computing systems and the participation of many GPUs in the process are required).
conclusions
We have implemented both options and at the current stage we are collecting statistics on their effectiveness. It is curious that both schemes turned out to be working and allowed to significantly improve the result of issuance, reducing the number of useless actions on the part of users. The end result for the business is an increase in user engagement, an increase in the total number of mutual likes and, as a result, the number of purchases of paid accounts.
Now the test operation phase of both solutions is underway and the algorithms are provided to users selectively, but if you want to test the first or second hypotheses yourself on a real base of profiles, register in the application and contact by email dushin@combox.io, indicate your username in the application in the letter, give access.