Throughout its history, from Asimov’s first robots to AlphaGo, AI has had its ups and downs. But in fact his story is just beginning.

Artificial intelligence is still very young. However, many significant events have already occurred in this area. Some of them attracted the attention of culture, others generated a blast wave, perceived only by scientists. Here are some key points that have had the greatest impact on AI.
1. Isaac Asimov first mentioned the " Three Laws of Robotics " (1942)
Azimov’s story “ Round dance ” marks the first appearance in the stories of this famous science fiction writer of the “Three Laws of Robotics”:
- A robot cannot harm a person or, through inaction, allow a person to be harmed.
- The robot must obey all orders given by a person, except in cases where these orders are contrary to the First Law.
- The robot must take care of its safety to the extent that it does not contradict the First or Second Laws.
In the story “Round dance,” Speedy's robot is placed in a position in which the third law conflicts with the first two. Azimov’s stories about robots made NF fans think, including scientists, about the possibility of thinking machines. To this day, people engage in intellectual exercises, applying the laws of Asimov to modern AI.
2. Alan Turing proposed his "Game of Imitation" (1950)
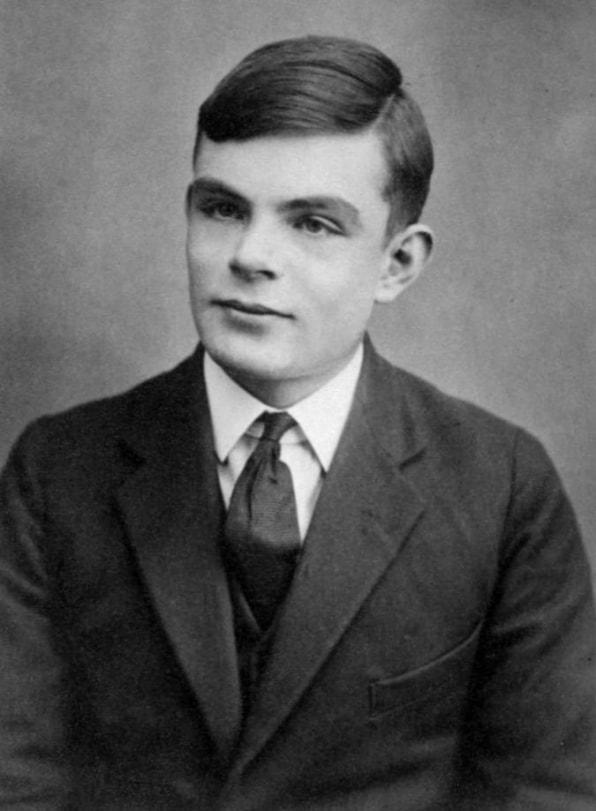
Alan Turing described the first principle of measuring the degree of rationality of a machine in 1950.
I propose to consider the question “Can machines think?” So began the influential Turing research work of 1950, which developed a belief system for reasoning about machine mind. He asked whether a machine can be considered reasonable if it can mimic human behavior.
This theoretical question gave rise to the famous “Simulation Game” [it will later be called the “ Turing Test ” / approx. transl.], an exercise in which a researcher-person must determine with whom he corresponded - with a computer or a person. At the time of Turing, there were no machines capable of passing this test; there are none today. However, his test gave a simple way to determine if the mind was in the car. He also helped shape the philosophy of AI.
3. Dartmouth AI Conference (1956)
By 1955, scientists around the world had already formed concepts such as neural networks and natural language, but there were still no unifying concepts encompassing various types of machine intelligence. John McCarthy, a professor of mathematics at Dartmouth College, coined the term “artificial intelligence” to bring them all together.
McCarthy led the group that applied for a grant to organize an AI conference in 1956. Many leading researchers of the time were invited to Dartmouth Hall in the summer of 1956. Scientists discussed various potential areas of AI study, including learning and searching, eyesight, logical reasoning, language and reason, games (in particular chess), human interactions with intelligent machines such as personal robots.
The general consensus of those discussions was that AI has tremendous potential to benefit people. The general field of research areas, the development of which can be influenced by machine intelligence, was outlined. The conference organized and inspired AI research for many years.
4. Frank Rosenblatt creates the perceptron (1957)
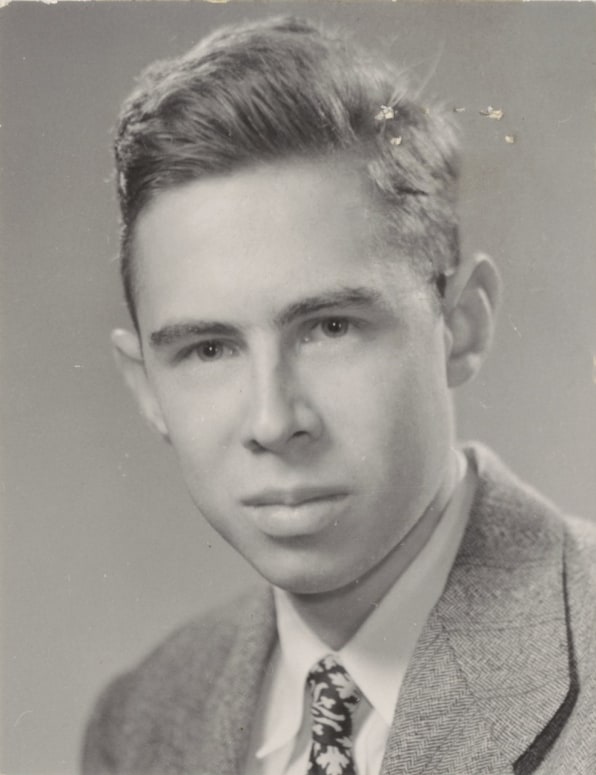
Frank Rosenblatt created a mechanical neural network at the Cornell Aeronautics Laboratory in 1957
The basic component of the neural network is called the “ perceptron ” [this is only the very first and primitive type of artificial neuron / approx. transl.]. A set of input data falls into the node that calculates the output value, and gives a classification and level of confidence. For example, the input data can analyze various aspects of the image based on the input data and “vote” (with a certain level of confidence) for whether there is a face on it. Then the node counts all the “voices” and the level of confidence, and gives out consensus. In the neural networks of today, running on powerful computers, billions of similar structures are working together.
However, perceptrons existed even before the advent of powerful computers. In the late 1950s, a young psychologist researcher Frank Rosenblatt created an electromechanical model of the perceptron called Mark I Perceptron, which is stored today at the Smithsonian Institution. It was an analog neural network, consisting of a grid of photosensitive elements connected by wires to banks of nodes containing electric motors and rotary resistors. Rosenblatt developed the “perceptron algorithm”, which controlled the network, which gradually adjusted the strength of the input signals so that, as a result, the objects were correctly identified - in fact, it was trained.
Scientists argued about the importance of this machine until the 1980s. It played an important role in creating the physical embodiment of the neural network, which until then existed mainly only in the form of a scientific concept.
5. AI faces its first winter (1970s)
Most of its history, AI existed only in research. For much of the 1960s, government agencies, in particular DARPA, poured money into research and practically did not require an investment report. AI researchers often exaggerated the potential of their work to continue to receive funding. Everything changed in the late 1960s and early 1970s. Two reports - one from the ALPAC Advisory Board for the U.S. Government in 1966, and the second from Lighthill for the British Government in 1973 - pragmatically evaluated progress in AI research and gave a very pessimistic forecast about the potential of this technology. Both reports questioned the existence of tangible progress in various areas of AI research. Lighthill in his report argued that AI for speech recognition tasks would be extremely difficult to scale to sizes that could be useful to the government or the military.
As a result, the governments of the United States and Britain began to cut funding for AI research for universities. DARPA, which funded AI research without problems in the 1960s, began to demand clear timelines from projects and a detailed description of the intended results. As a result, it began to seem that AI did not live up to expectations, and could never reach the level of human capabilities. The first “winter” of AI lasted all the 1970s and 80s.
6. The arrival of the second winter of AI (1987)
The 1980s began with the development and first successes of “ expert systems ” that stored large amounts of data and emulated the decision-making process by people. The technology was originally developed at Carnegie Mellon University for Digital Equipment Corporation, and then other corporations began to quickly implement it. However, expert systems required expensive specialized equipment, and this became a problem when similar power and cheaper workstations from Sun Microsystems as well as personal computers from Apple and IBM began to appear. The market for expert computer systems collapsed in 1987 when major equipment manufacturers left it.
The success of expert systems in the early 80s inspired DARPA to increase funding for AI research, but it soon changed again, and the agency cut back on most of this funding, leaving only a few programs. Again, the term “artificial intelligence” in the research community has become almost forbidden. To prevent them from being perceived as impractical dreamers in search of financing, researchers began to use other names for work related to SS - “computer science”, “machine learning” and “analytics”. This second winter of AI continued until the 2000s.
7. IBM Deep Blue defeats Kasparov (1997)
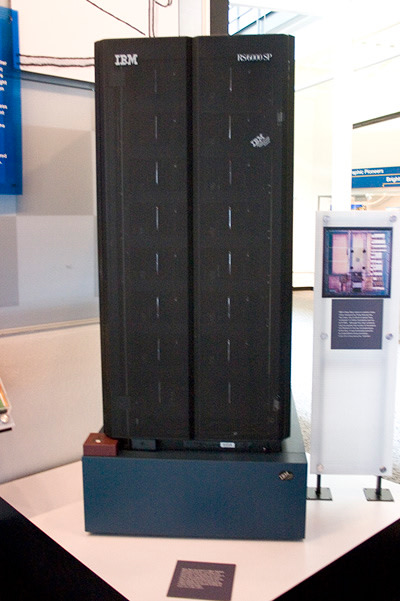
IBM Deep Blue defeated the world's best chess player, Garry Kasparov, in 1997.
Public awareness of AI improved in 1997 when IBM's Deep Blue chess computer defeated then-world champion Garry Kasparov. Of the six games held in the television studio, Deep Blue won in two, Kasparov in one, and three ended in a draw. Earlier that year, Kasparov defeated the previous version of Deep Blue.
The Deep Blue computer had enough computing power, and he used the "brute force method", or exhaustive search, evaluating 200 million possible moves per second and choosing the best one. People’s abilities are limited to evaluating only about 50 moves after each move. The work of Deep Blue was similar to the work of AI, but the computer did not think about strategies and did not learn the game, as the systems that followed it could do.
Nevertheless, the victory of Deep Blue over Kasparov impressively returned AI to the circle of public attention. Some people were fascinated. Others did not like that the machine beat an expert in chess. Investors were impressed: Deep Blue’s victory of $ 10 raised the value of IBM shares, bringing them to the maximum of that time.
8. Neural network sees cats (2011)
By 2011, scientists from universities around the world talked about neural networks and created them. That year, Google programmer Jeff Dean met Stanford computer science professor Andrew Eun . Together, they conceived the creation of a large neural network, provided with the huge computing power of Google servers, which could feed a huge set of images.
The neural network they created worked on 16,000 server processors. They fed her 10 million random and unlabeled frames from YouTube videos. Dean and Eun did not ask the neural network to give out any specific information or to mark these images. When a neural network works this way, learning without a teacher, it naturally tries to find patterns in the data and forms classifications.
The neural network processed the images for three days. Then she produced three blurry images denoting visual images that she met again and again in the training data - the face of a person, the body of a person and a cat. This study was a major breakthrough in the use of neural networks and non-teacher learning in computer vision. It also marked the start of the Google Brain project.
9. Joffrey Hinton unleashed deep neural networks (2012)
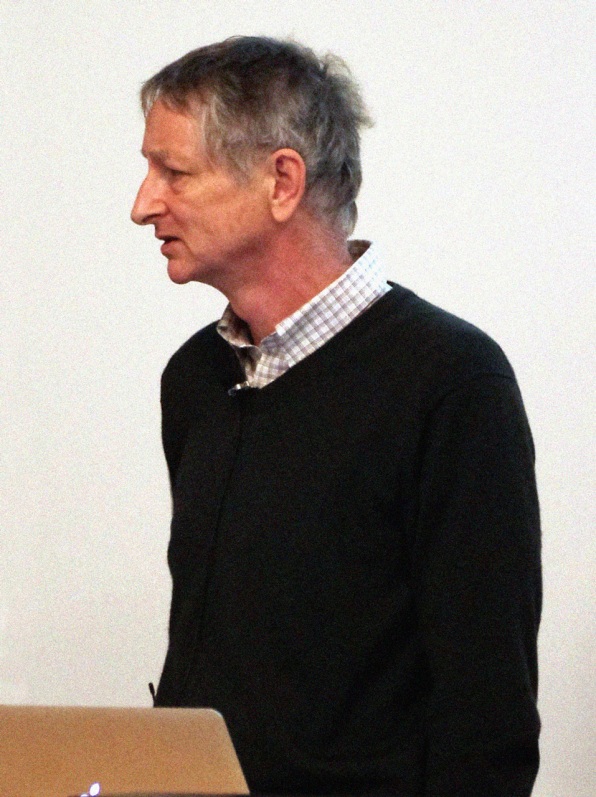
Joffrey Hinton's study helped revive interest in deep learning
A year after the breakthrough, Dean and Un, a professor at the University of Toronto, Joffrey Hinton, and two of his students created a neural network for computer vision, AlexNet, to participate in the ImageNet image recognition competition. Participants had to use their systems to process millions of test images and identify them with the highest possible accuracy. AlexNet won the competition with a percentage of errors two times less than that of the nearest competitor. In five versions of the image caption given by the neural network, only in 15.3% of cases there was no correct option. The previous record was 26% of errors.
This victory convincingly showed that deep neural networks running on GPUs, where better than other systems can accurately determine and classify images. This event, perhaps more than others, influenced the revival of interest in deep neural networks, and earned Hinton the nickname "godfather of deep learning." Together with other AI gurus, Yoshua Benjio and Jan Lekun, Hinton received the long-awaited Turing Award in 2018.
10. AlphaGo beats world go champion (2016)
In 2013, researchers at British startup DeepMind published a paper describing how a neural network learned to play and win in 50 old Atari games. Impressed by this, Google bought the company - as they say, for $ 400 million. However, the main fame of DeepMind was still ahead.
A few years later, scientists from DeepMind, now within the Google framework, switched from Atari games to one of the oldest AI tasks - the Japanese board game go. They developed the AlphaGo neural network, capable of playing go and learning while playing. The program has conducted thousands of games against other versions of AlphaGo, learning from losses and wins.
And it worked. AlphaGo defeated the greatest go player in the world, Lee Sedola , 4-1 in a series of games in March 2016. The process was filmed for a documentary. When viewing it, it is difficult not to notice the sadness with which Sedol perceived the loss. It seemed that all people lost, and not just one person.
Recent advances in the field of deep neural networks have changed the field of AI so much that its real history, perhaps, is only just beginning. We are waiting for a lot of hopes, hype and impatience, but it is now clear that AI will affect all aspects of life of the XXI century - and perhaps even more than the Internet did in its time.