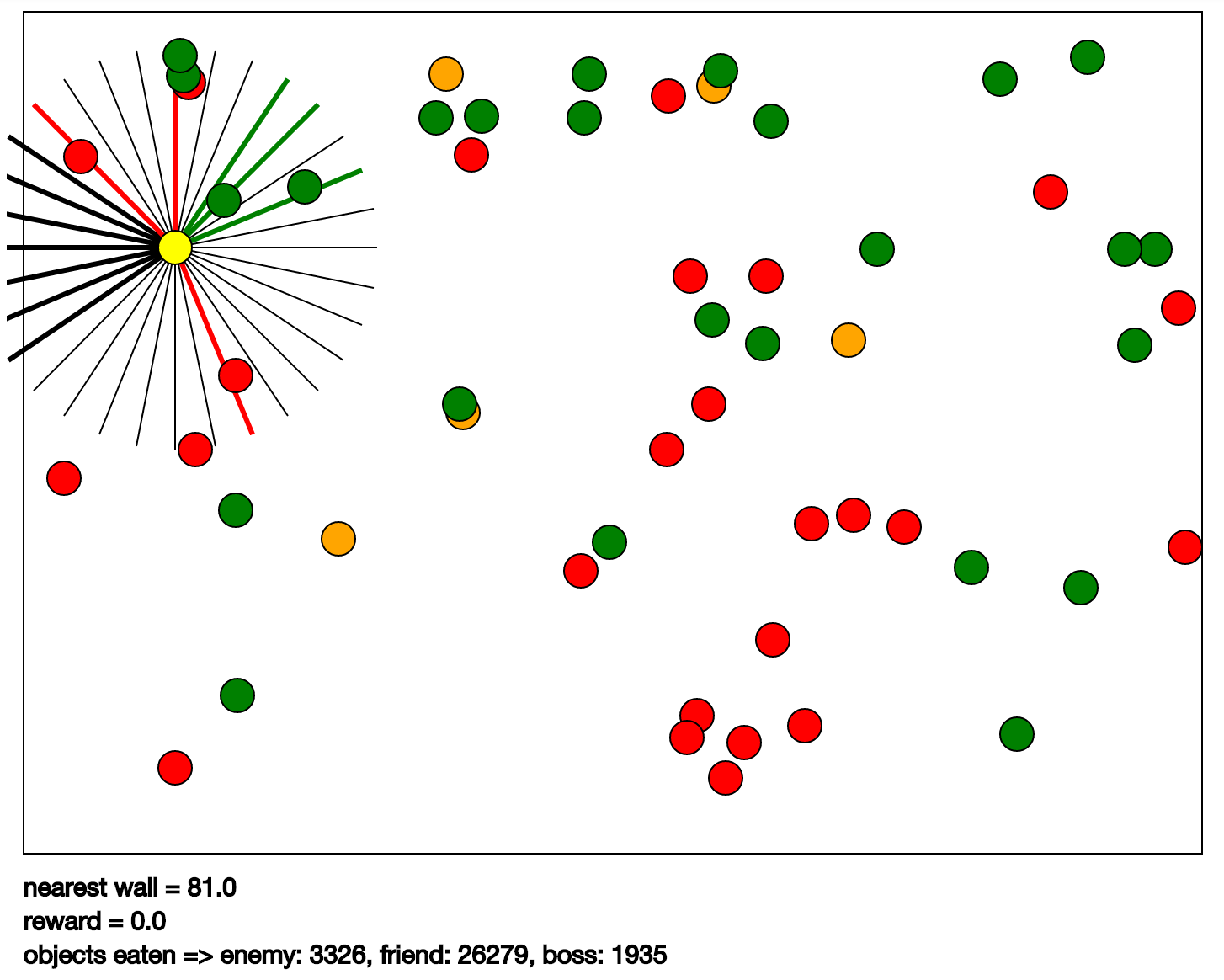
みなさんこんにちは!
多くの人がGoogle DeepMindについて聞いたことがあると思います。 人間よりもアタリゲームを上手にプレイするためのプログラムの教え方について 本日は、同様のことを行う方法に関する記事を紹介したいと思います。 この記事は、強化学習の特殊なケースであるQラーニングアプリケーションの例のアイデアとコードのレビューです。 この例は、Google従業員DeepMindの記事に基づいています 。
ゲーム
この例では、ゲームKarpathyゲームを使用します。 彼女はCPDVに描かれています。 その本質は次のとおりです。緑のボールを「食べ」、赤やオレンジを食べないように黄色のボールを制御する必要があります。 オレンジの場合、赤よりも大きなペナルティが与えられます。 黄色のボールには、視覚に関与する放射状に広がるセグメントがあります(プログラムでは、目と呼ばれます)。 そのようなセグメントの助けを借りて、プログラムは、セグメントの方向に最も近いオブジェクトのタイプ、その速度と距離を感知します。 オブジェクトのタイプは、ボールまたは壁の色です。 入力データのセットは、次のように取得されます。各目からのデータと独自の速度。 出力は黄色のボール制御コマンドです。 実際、これらは上、左、下、右の4つの方向の加速度です。
アイデア
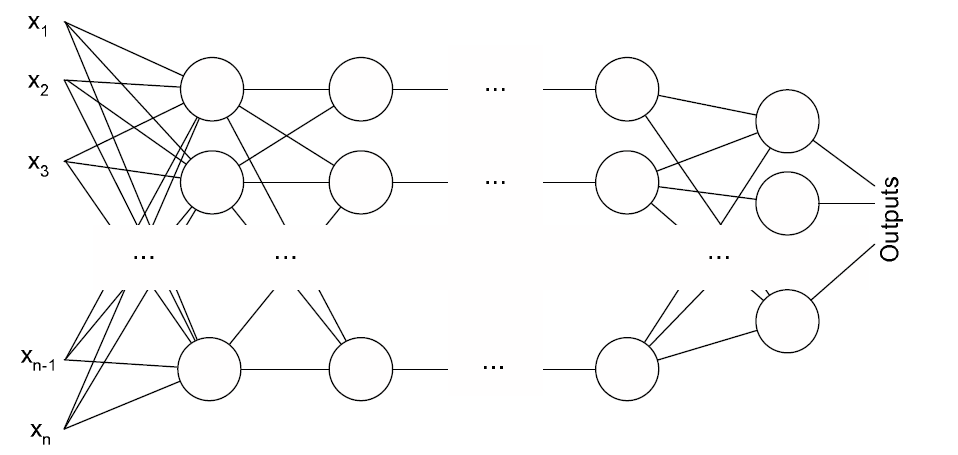
多層パーセプトロンがこのゲームをプレイします。 入力で、彼は上記の入力データを持っています。 出力は、可能なアクションのそれぞれの有用性です。 パーセプトロンは、逆伝播法によって訓練されます。 具体的には、RMSPropメソッドが使用されます。 その特徴は、最適化のためにすぐに多数の例を使用することですが、これが唯一の機能ではありません。 この方法の詳細については、 これらのスライドをご覧ください。 彼らはRMSPropだけではありません。 私はまだ良いものを見つけていません。 ニューラルネットワークの出力誤差は、同じQ学習を使用して計算されます。
テンソルフロー
最近リリースされたTensorFlowライブラリのおかげで、アルゴリズムの独自の実装を書くことなく、これらのほとんどすべてを多少簡単にコーディングできます。 このライブラリを使用したプログラミングは、結果を得るために必要な計算のグラフを記述することになります。 次に、このグラフはTensorFlowセッションに送信され、そこで計算自体が実行されます。 RMSPropは完全にTensorFlowから取得されます。 ニューラルネットワークはそこから行列に実装されます。 Q学習は、従来のTensorFlow操作にも実装されています。
コード
次に、サンプルコードの最も興味深い場所を見てみましょう。
models.py-多層パーセプトロン
import math import tensorflow as tf from .utils import base_name # MLP # class Layer(object): # input_sizes - , MLP # output_size - # scope - , TensorFlow # ( https://www.tensorflow.org/versions/master/how_tos/variable_scope/index.html) def __init__(self, input_sizes, output_size, scope): """Cretes a neural network layer.""" if type(input_sizes) != list: input_sizes = [input_sizes] self.input_sizes = input_sizes self.output_size = output_size self.scope = scope or "Layer" # with tf.variable_scope(self.scope): # self.Ws = [] for input_idx, input_size in enumerate(input_sizes): # W_name = "W_%d" % (input_idx,) # - W_initializer = tf.random_uniform_initializer( -1.0 / math.sqrt(input_size), 1.0 / math.sqrt(input_size)) # - input_size x output_size W_var = tf.get_variable(W_name, (input_size, output_size), initializer=W_initializer) self.Ws.append(W_var) # # self.b = tf.get_variable("b", (output_size,), initializer=tf.constant_initializer(0)) # # xs - # def __call__(self, xs): if type(xs) != list: xs = [xs] assert len(xs) == len(self.Ws), \ "Expected %d input vectors, got %d" % (len(self.Ws), len(xs)) with tf.variable_scope(self.scope): # # - # - # - + return sum([tf.matmul(x, W) for x, W in zip(xs, self.Ws)]) + self.b # # def variables(self): return [self.b] + self.Ws def copy(self, scope=None): scope = scope or self.scope + "_copy" with tf.variable_scope(scope) as sc: for v in self.variables(): tf.get_variable(base_name(v), v.get_shape(), initializer=lambda x,dtype=tf.float32: v.initialized_value()) sc.reuse_variables() return Layer(self.input_sizes, self.output_size, scope=sc) # class MLP(object): # input_sizes - , , # , # , # , # hiddens - , # 2 100 # - 4 , , # , # nonlinearities - , <a href="https://ru.wikipedia.org/wiki/%D0%98%D1%81%D0%BA%D1%83%D1%81%D1%81%D1%82%D0%B2%D0%B5%D0%BD%D0%BD%D1%8B%D0%B9_%D0%BD%D0%B5%D0%B9%D1%80%D0%BE%D0%BD"> </a> # scope - , TensorFlow # # given_layers - def __init__(self, input_sizes, hiddens, nonlinearities, scope=None, given_layers=None): self.input_sizes = input_sizes self.hiddens = hiddens self.input_nonlinearity, self.layer_nonlinearities = nonlinearities[0], nonlinearities[1:] self.scope = scope or "MLP" assert len(hiddens) == len(nonlinearities), \ "Number of hiddens must be equal to number of nonlinearities" with tf.variable_scope(self.scope): if given_layers is not None: # self.input_layer = given_layers[0] self.layers = given_layers[1:] else: # # self.input_layer = Layer(input_sizes, hiddens[0], scope="input_layer") self.layers = [] # for l_idx, (h_from, h_to) in enumerate(zip(hiddens[:-1], hiddens[1:])): self.layers.append(Layer(h_from, h_to, scope="hidden_layer_%d" % (l_idx,))) # # xs - # def __call__(self, xs): if type(xs) != list: xs = [xs] with tf.variable_scope(self.scope): # hidden = self.input_nonlinearity(self.input_layer(xs)) for layer, nonlinearity in zip(self.layers, self.layer_nonlinearities): # hidden = nonlinearity(layer(hidden)) return hidden # def variables(self): res = self.input_layer.variables() for layer in self.layers: res.extend(layer.variables()) return res def copy(self, scope=None): scope = scope or self.scope + "_copy" nonlinearities = [self.input_nonlinearity] + self.layer_nonlinearities given_layers = [self.input_layer.copy()] + [layer.copy() for layer in self.layers] return MLP(self.input_sizes, self.hiddens, nonlinearities, scope=scope, given_layers=given_layers)
distinct_deepq.py-Qラーニングの実装
import numpy as np import random import tensorflow as tf from collections import deque class DiscreteDeepQ(object): # def __init__(self, observation_size, num_actions, observation_to_actions, optimizer, session, random_action_probability=0.05, exploration_period=1000, store_every_nth=5, train_every_nth=5, minibatch_size=32, discount_rate=0.95, max_experience=30000, target_network_update_rate=0.01, summary_writer=None): # """Initialized the Deepq object. Based on: https://www.cs.toronto.edu/~vmnih/docs/dqn.pdf Parameters ------- observation_size : int length of the vector passed as observation num_actions : int number of actions that the model can execute observation_to_actions: dali model model that implements activate function that can take in observation vector or a batch and returns scores (of unbounded values) for each action for each observation. input shape: [batch_size, observation_size] output shape: [batch_size, num_actions] optimizer: tf.solver.* optimizer for prediction error session: tf.Session session on which to execute the computation random_action_probability: float (0 to 1) exploration_period: int probability of choosing a random action (epsilon form paper) annealed linearly from 1 to random_action_probability over exploration_period store_every_nth: int to further decorrelate samples do not all transitions, but rather every nth transition. For example if store_every_nth is 5, then only 20% of all the transitions is stored. train_every_nth: int normally training_step is invoked every time action is executed. Depending on the setup that might be too often. When this variable is set set to n, then only every n-th time training_step is called will the training procedure actually be executed. minibatch_size: int number of state,action,reward,newstate tuples considered during experience reply dicount_rate: float (0 to 1) how much we care about future rewards. max_experience: int maximum size of the reply buffer target_network_update_rate: float how much to update target network after each iteration. Let's call target_network_update_rate alpha, target network T, and network N. Every time N gets updated we execute: T = (1-alpha)*T + alpha*N summary_writer: tf.train.SummaryWriter writer to log metrics """ """ Deepq : https://www.cs.toronto.edu/~vmnih/docs/dqn.pdf ------- observation_size : int ( ) num_actions : int observation_to_actions: dali model ( ), : [batch_size, observation_size] : [batch_size, num_actions] optimizer: tf.solver.* RMSProp session: tf.Session TensorFlow random_action_probability: float (0 to 1) , , exploration_period: int , 1 random_action_probability store_every_nth: int . train_every_nth: int training_step ( ) . , . minibatch_size: int RMSProp. , , dicount_rate: float (0 to 1) Q-learning max_experience: int target_network_update_rate: float , 2 T - target_q_network N - q_network , . T N. N, : alpha = target_network_update_rate T = (1-alpha)*T + alpha*N summary_writer: tf.train.SummaryWriter """ # memorize arguments self.observation_size = observation_size self.num_actions = num_actions self.q_network = observation_to_actions self.optimizer = optimizer self.s = session self.random_action_probability = random_action_probability self.exploration_period = exploration_period self.store_every_nth = store_every_nth self.train_every_nth = train_every_nth self.minibatch_size = minibatch_size self.discount_rate = tf.constant(discount_rate) self.max_experience = max_experience self.target_network_update_rate = \ tf.constant(target_network_update_rate) # deepq state self.actions_executed_so_far = 0 self.experience = deque() self.iteration = 0 self.summary_writer = summary_writer self.number_of_times_store_called = 0 self.number_of_times_train_called = 0 self.create_variables() # # # ( ) def linear_annealing(self, n, total, p_initial, p_final): """Linear annealing between p_initial and p_final over total steps - computes value at step n""" if n >= total: return p_final else: return p_initial - (n * (p_initial - p_final)) / (total) # TensorFlow # # Q-learning def create_variables(self): # T N self.target_q_network = self.q_network.copy(scope="target_network") # # FOR REGULAR ACTION SCORE COMPUTATION with tf.name_scope("taking_action"): # self.observation = tf.placeholder(tf.float32, (None, self.observation_size), name="observation") # self.action_scores = tf.identity(self.q_network(self.observation), name="action_scores") tf.histogram_summary("action_scores", self.action_scores) # self.predicted_actions = tf.argmax(self.action_scores, dimension=1, name="predicted_actions") # with tf.name_scope("estimating_future_rewards"): # FOR PREDICTING TARGET FUTURE REWARDS # - self.next_observation = tf.placeholder(tf.float32, (None, self.observation_size), name="next_observation") # - self.next_observation_mask = tf.placeholder(tf.float32, (None,), name="next_observation_mask") # self.next_action_scores = tf.stop_gradient(self.target_q_network(self.next_observation)) tf.histogram_summary("target_action_scores", self.next_action_scores) # - self.rewards = tf.placeholder(tf.float32, (None,), name="rewards") # target_values = tf.reduce_max(self.next_action_scores, reduction_indices=[1,]) * self.next_observation_mask # r + DF * MAX(Q,s) Q-learning self.future_rewards = self.rewards + self.discount_rate * target_values # N with tf.name_scope("q_value_precition"): # FOR PREDICTION ERROR # self.action_mask = tf.placeholder(tf.float32, (None, self.num_actions), name="action_mask") # self.masked_action_scores = tf.reduce_sum(self.action_scores * self.action_mask, reduction_indices=[1,]) # # - (r + DF * MAX(Q,s) — Q[s',a']) temp_diff = self.masked_action_scores - self.future_rewards # # RMSProp self.prediction_error = tf.reduce_mean(tf.square(temp_diff)) # RMSProp, - gradients = self.optimizer.compute_gradients(self.prediction_error) for i, (grad, var) in enumerate(gradients): if grad is not None: gradients[i] = (tf.clip_by_norm(grad, 5), var) # Add histograms for gradients. for grad, var in gradients: tf.histogram_summary(var.name, var) if grad: tf.histogram_summary(var.name + '/gradients', grad) # - self.train_op = self.optimizer.apply_gradients(gradients) # T # T = (1-alpha)*T + alpha*N # UPDATE TARGET NETWORK with tf.name_scope("target_network_update"): self.target_network_update = [] for v_source, v_target in zip(self.q_network.variables(), self.target_q_network.variables()): # this is equivalent to target = (1-alpha) * target + alpha * source update_op = v_target.assign_sub(self.target_network_update_rate * (v_target - v_source)) self.target_network_update.append(update_op) self.target_network_update = tf.group(*self.target_network_update) # summaries tf.scalar_summary("prediction_error", self.prediction_error) self.summarize = tf.merge_all_summaries() self.no_op1 = tf.no_op() # def action(self, observation): """Given observation returns the action that should be chosen using DeepQ learning strategy. Does not backprop.""" assert len(observation.shape) == 1, \ "Action is performed based on single observation." self.actions_executed_so_far += 1 # exploration_p = self.linear_annealing(self.actions_executed_so_far, self.exploration_period, 1.0, self.random_action_probability) if random.random() < exploration_p: # return random.randint(0, self.num_actions - 1) else: # return self.s.run(self.predicted_actions, {self.observation: observation[np.newaxis,:]})[0] # # # def store(self, observation, action, reward, newobservation): """Store experience, where starting with observation and execution action, we arrived at the newobservation and got thetarget_network_update reward reward If newstate is None, the state/action pair is assumed to be terminal """ if self.number_of_times_store_called % self.store_every_nth == 0: self.experience.append((observation, action, reward, newobservation)) if len(self.experience) > self.max_experience: self.experience.popleft() self.number_of_times_store_called += 1 # def training_step(self): """Pick a self.minibatch_size exeperiences from reply buffer and backpropage the value function. """ if self.number_of_times_train_called % self.train_every_nth == 0: if len(self.experience) < self.minibatch_size: return # # minibatch_size # sample experience. samples = random.sample(range(len(self.experience)), self.minibatch_size) samples = [self.experience[i] for i in samples] # # # bach states states = np.empty((len(samples), self.observation_size)) newstates = np.empty((len(samples), self.observation_size)) action_mask = np.zeros((len(samples), self.num_actions)) newstates_mask = np.empty((len(samples),)) rewards = np.empty((len(samples),)) for i, (state, action, reward, newstate) in enumerate(samples): states[i] = state action_mask[i] = 0 action_mask[i][action] = 1 rewards[i] = reward if newstate is not None: newstates[i] = newstate newstates_mask[i] = 1 else: newstates[i] = 0 newstates_mask[i] = 0 calculate_summaries = self.iteration % 100 == 0 and \ self.summary_writer is not None # # # # ( # ) cost, _, summary_str = self.s.run([ self.prediction_error, self.train_op, self.summarize if calculate_summaries else self.no_op1, ], { self.observation: states, self.next_observation: newstates, self.next_observation_mask: newstates_mask, self.action_mask: action_mask, self.rewards: rewards, }) # self.s.run(self.target_network_update) if calculate_summaries: self.summary_writer.add_summary(summary_str, self.iteration) self.iteration += 1 self.number_of_times_train_called += 1
karpathy_game.py-ニューラルネットワークでプレイされるゲーム
import math import matplotlib.pyplot as plt import numpy as np import random import time from collections import defaultdict from euclid import Circle, Point2, Vector2, LineSegment2 import tf_rl.utils.svg as svg # # # # class GameObject(object): def __init__(self, position, speed, obj_type, settings): """Esentially represents circles of different kinds, which have position and speed.""" self.settings = settings self.radius = self.settings["object_radius"] self.obj_type = obj_type self.position = position self.speed = speed self.bounciness = 1.0 def wall_collisions(self): """Update speed upon collision with the wall.""" world_size = self.settings["world_size"] for dim in range(2): if self.position[dim] - self.radius <= 0 and self.speed[dim] < 0: self.speed[dim] = - self.speed[dim] * self.bounciness elif self.position[dim] + self.radius + 1 >= world_size[dim] and self.speed[dim] > 0: self.speed[dim] = - self.speed[dim] * self.bounciness def move(self, dt): """Move as if dt seconds passed""" self.position += dt * self.speed self.position = Point2(*self.position) def step(self, dt): """Move and bounce of walls.""" self.wall_collisions() self.move(dt) def as_circle(self): return Circle(self.position, float(self.radius)) def draw(self): """Return svg object for this item.""" color = self.settings["colors"][self.obj_type] return svg.Circle(self.position + Point2(10, 10), self.radius, color=color) # . # , , # , # . # , ^ , , # , # observe, # class KarpathyGame(object): def __init__(self, settings): """Initiallize game simulator with settings""" self.settings = settings self.size = self.settings["world_size"] self.walls = [LineSegment2(Point2(0,0), Point2(0,self.size[1])), LineSegment2(Point2(0,self.size[1]), Point2(self.size[0], self.size[1])), LineSegment2(Point2(self.size[0], self.size[1]), Point2(self.size[0], 0)), LineSegment2(Point2(self.size[0], 0), Point2(0,0))] self.hero = GameObject(Point2(*self.settings["hero_initial_position"]), Vector2(*self.settings["hero_initial_speed"]), "hero", self.settings) if not self.settings["hero_bounces_off_walls"]: self.hero.bounciness = 0.0 self.objects = [] for obj_type, number in settings["num_objects"].items(): for _ in range(number): self.spawn_object(obj_type) self.observation_lines = self.generate_observation_lines() self.object_reward = 0 self.collected_rewards = [] # # # every observation_line sees one of objects or wall and # two numbers representing speed of the object (if applicable) self.eye_observation_size = len(self.settings["objects"]) + 3 # , , # - # additionally there are two numbers representing agents own speed. self.observation_size = self.eye_observation_size * len(self.observation_lines) + 2 self.last_observation = np.zeros(self.observation_size) self.directions = [Vector2(*d) for d in [[1,0], [0,1], [-1,0],[0,-1]]] self.num_actions = len(self.directions) self.objects_eaten = defaultdict(lambda: 0) def perform_action(self, action_id): """Change speed to one of hero vectors""" assert 0 <= action_id < self.num_actions self.hero.speed *= 0.8 self.hero.speed += self.directions[action_id] * self.settings["delta_v"] def spawn_object(self, obj_type): """Spawn object of a given type and add it to the objects array""" radius = self.settings["object_radius"] position = np.random.uniform([radius, radius], np.array(self.size) - radius) position = Point2(float(position[0]), float(position[1])) max_speed = np.array(self.settings["maximum_speed"]) speed = np.random.uniform(-max_speed, max_speed).astype(float) speed = Vector2(float(speed[0]), float(speed[1])) self.objects.append(GameObject(position, speed, obj_type, self.settings)) def step(self, dt): """Simulate all the objects for a given ammount of time. Also resolve collisions with the hero""" for obj in self.objects + [self.hero] : obj.step(dt) self.resolve_collisions() def squared_distance(self, p1, p2): return (p1[0] - p2[0]) ** 2 + (p1[1] - p2[1]) ** 2 def resolve_collisions(self): """If hero touches, hero eats. Also reward gets updated.""" collision_distance = 2 * self.settings["object_radius"] collision_distance2 = collision_distance ** 2 to_remove = [] for obj in self.objects: if self.squared_distance(self.hero.position, obj.position) < collision_distance2: to_remove.append(obj) for obj in to_remove: self.objects.remove(obj) self.objects_eaten[obj.obj_type] += 1 self.object_reward += self.settings["object_reward"][obj.obj_type] self.spawn_object(obj.obj_type) def inside_walls(self, point): """Check if the point is inside the walls""" EPS = 1e-4 return (EPS <= point[0] < self.size[0] - EPS and EPS <= point[1] < self.size[1] - EPS) # def observe(self): """Return observation vector. For all the observation directions it returns representation of the closest object to the hero - might be nothing, another object or a wall. Representation of observation for all the directions will be concatenated. """ num_obj_types = len(self.settings["objects"]) + 1 # and wall max_speed_x, max_speed_y = self.settings["maximum_speed"] # observable_distance = self.settings["observation_line_length"] # relevant_objects = [obj for obj in self.objects if obj.position.distance(self.hero.position) < observable_distance] # # # objects sorted from closest to furthest relevant_objects.sort(key=lambda x: x.position.distance(self.hero.position)) observation = np.zeros(self.observation_size) observation_offset = 0 # for i, observation_line in enumerate(self.observation_lines): # shift to hero position observation_line = LineSegment2(self.hero.position + Vector2(*observation_line.p1), self.hero.position + Vector2(*observation_line.p2)) observed_object = None # # if end of observation line is outside of walls, we see the wall. if not self.inside_walls(observation_line.p2): observed_object = "**wall**" # for obj in relevant_objects: if observation_line.distance(obj.position) < self.settings["object_radius"]: # observed_object = obj break # # , object_type_id = None speed_x, speed_y = 0, 0 proximity = 0 if observed_object == "**wall**": # wall seen # object_type_id = num_obj_types - 1 # # , , # , , # # # # a wall has fairly low speed... # speed_x, speed_y = 0, 0 # I think relative speed is better than absolute speed_x, speed_y = tuple (-self.hero.speed) # best candidate is intersection between # observation_line and a wall, that's # closest to the hero best_candidate = None for wall in self.walls: candidate = observation_line.intersect(wall) if candidate is not None: if (best_candidate is None or best_candidate.distance(self.hero.position) > candidate.distance(self.hero.position)): best_candidate = candidate if best_candidate is None: # assume it is due to rounding errors # and wall is barely touching observation line proximity = observable_distance else: proximity = best_candidate.distance(self.hero.position) elif observed_object is not None: # agent seen # # object_type_id = self.settings["objects"].index(observed_object.obj_type) # # speed_x, speed_y = tuple(observed_object.speed - self.hero.speed) intersection_segment = obj.as_circle().intersect(observation_line) assert intersection_segment is not None # try: proximity = min(intersection_segment.p1.distance(self.hero.position), intersection_segment.p2.distance(self.hero.position)) except AttributeError: proximity = observable_distance for object_type_idx_loop in range(num_obj_types): # 1.0 # observation[observation_offset + object_type_idx_loop] = 1.0 if object_type_id is not None: # # 0.0 1.0 # observation[observation_offset + object_type_id] = proximity / observable_distance # observation[observation_offset + num_obj_types] = speed_x / max_speed_x observation[observation_offset + num_obj_types + 1] = speed_y / max_speed_y assert num_obj_types + 2 == self.eye_observation_size observation_offset += self.eye_observation_size # # observation[observation_offset] = self.hero.speed[0] / max_speed_x observation[observation_offset + 1] = self.hero.speed[1] / max_speed_y assert observation_offset + 2 == self.observation_size self.last_observation = observation return observation def distance_to_walls(self): """Returns distance of a hero to walls""" res = float('inf') for wall in self.walls: res = min(res, self.hero.position.distance(wall)) return res - self.settings["object_radius"] def collect_reward(self): """Return accumulated object eating score + current distance to walls score""" wall_reward = self.settings["wall_distance_penalty"] * \ np.exp(-self.distance_to_walls() / self.settings["tolerable_distance_to_wall"]) assert wall_reward < 1e-3, "You are rewarding hero for being close to the wall!" total_reward = wall_reward + self.object_reward self.object_reward = 0 self.collected_rewards.append(total_reward) return total_reward def plot_reward(self, smoothing = 30): """Plot evolution of reward over time.""" plottable = self.collected_rewards[:] while len(plottable) > 1000: for i in range(0, len(plottable) - 1, 2): plottable[i//2] = (plottable[i] + plottable[i+1]) / 2 plottable = plottable[:(len(plottable) // 2)] x = [] for i in range(smoothing, len(plottable)): chunk = plottable[i-smoothing:i] x.append(sum(chunk) / len(chunk)) plt.plot(list(range(len(x))), x) def generate_observation_lines(self): """Generate observation segments in settings["num_observation_lines"] directions""" result = [] start = Point2(0.0, 0.0) end = Point2(self.settings["observation_line_length"], self.settings["observation_line_length"]) for angle in np.linspace(0, 2*np.pi, self.settings["num_observation_lines"], endpoint=False): rotation = Point2(math.cos(angle), math.sin(angle)) current_start = Point2(start[0] * rotation[0], start[1] * rotation[1]) current_end = Point2(end[0] * rotation[0], end[1] * rotation[1]) result.append( LineSegment2(current_start, current_end)) return result def _repr_html_(self): return self.to_html() def to_html(self, stats=[]): """Return svg representation of the simulator""" stats = stats[:] recent_reward = self.collected_rewards[-100:] + [0] objects_eaten_str = ', '.join(["%s: %s" % (o,c) for o,c in self.objects_eaten.items()]) stats.extend([ "nearest wall = %.1f" % (self.distance_to_walls(),), "reward = %.1f" % (sum(recent_reward)/len(recent_reward),), "objects eaten => %s" % (objects_eaten_str,), ]) scene = svg.Scene((self.size[0] + 20, self.size[1] + 20 + 20 * len(stats))) scene.add(svg.Rectangle((10, 10), self.size)) num_obj_types = len(self.settings["objects"]) + 1 # and wall observation_offset = 0; for line in self.observation_lines: # getting color of the line linecolor = 'black'; linewidth = '1px'; for object_type_idx_loop in range(num_obj_types): if self.last_observation[observation_offset + object_type_idx_loop] < 1.0: if object_type_idx_loop < num_obj_types - 1: linecolor = self.settings["colors"][self.settings["objects"][object_type_idx_loop]]; linewidth = '3px'; observation_offset += self.eye_observation_size scene.add(svg.Line(line.p1 + self.hero.position + Point2(10,10), line.p2 + self.hero.position + Point2(10,10), color = linecolor, stroke = linecolor, stroke_width = linewidth)) for obj in self.objects + [self.hero] : scene.add(obj.draw()) offset = self.size[1] + 15 for txt in stats: scene.add(svg.Text((10, offset + 20), txt, 15)) offset += 20 return scene
すべての動作を確認するには、 IPython Notebookが必要です。 これらはすべて、彼のために台本にまとめられるので。 スクリプトは、notebooks / karpathy_game.ipynbにあります。
結果
記事を書いている間に、私は数時間トレーニングを始めました。 以下にビデオを示します。最終的に、私はかなり短時間でグリッドを学習しました。
次に行く場所
さらに、このメソッドを仮想quadrocopterに実装しようとする予定です 。 まず、安定化を試みたいです。 それから、もし成功したら、それを飛ばそうとしますが、おそらく多層パーセプトロンの代わりに畳み込みネットワークが必要になるでしょう。
この例は、ユーザーnivwusquorumによってGitHubに慎重にレイアウトされています。これについては、彼に多大な感謝を表したいと思います。