
ãæ³šæ
ãã®åéã®å€ãã®è±èªã®ååã«ã€ããŠã¯ã翻蚳ãå©çšã§ããªããã翻蚳è
ã«ãã£ãŠç°ãªããããŸãšããªç€ŸäŒã§ããã䜿çšããããšã¯ãŸã£ããäžå¯èœã§ãããã®ããããã§ãã ãããã£ãŠãè±èªçã§ã¯å€ãã®ååãä»ããããŸãã èå³ã®ããæ¹ã®ããã«ãSSMã®ã¢ããªã³ã°ã«é¢ããæé«ã®æ¬ã® 1ã€ã ãŸãããã·ã¢èªã®ç¿»èš³ã®ãããã¯ã«é¢ããè¯ãæ¬ã¯ã»ãšãã©ãªãããšã倿ããŸããã ãªãã·ã§ã³ãšããŠ-ã¢ã¬ã¯ãµã³ããŒã»ãã£ãã©ã³ãã®äœå㯠ãå¥ã®èšäºãšããŠåºçãããŠããŸãããå®éã«ã¯éåžžã«ç°¡ç¥åãããããŒãžã§ã³ã®æ¬ã®ã³ããŒã§ãã
ããã§ã¯å§ããŸãããã
1.åæããŒã¿åæ
ãã®éšåã¯ãããã»ã¹ã®æãéèŠãªéšåã®1ã€ã§ãããªããªããä»ééããå Žåãæ®ãã®äœæ¥ã¯ãã¹ãŠæéã®ç¡é§ã«ãªãå¯èœæ§ãããããã§ãã åã®èšäºã§äœ¿çšããã¢ã¹ã¯ã¯å°åã®å庫矀ã®1ã€ã§ãååã®åºè·ã«é¢ããããŒã¿ãéããŸãã ããããããŸãããïŒ
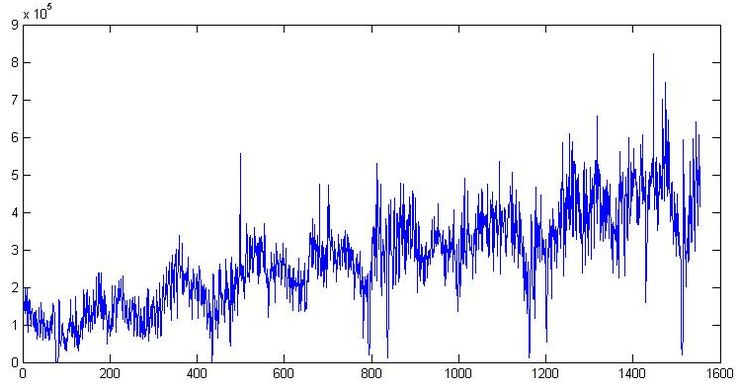
第äžã«ãçŽ300æ¥ã®ãªãŒããŒã®ãã¬ã³ããšãµã€ã¯ã«ãæããã«ãããŸãã ãã£ãŒããéããŸãã ç ãæã£ãŠè¡ããŸãããã æ¥ãŠãããäžåºŠéããŠãæ°åãã®ãã®ãèŠãŠã¿ãŸãããã åºè·æ¥ã¯2009幎9æ1æ¥ã®åœ¢åŒã§ç€ºãããŠãããããExcelã§éããææ¥ã衚瀺ããããã«ããŒã¿ã[$ -F800] ddddãmmmm ddãyyyyã®åœ¢åŒã«è»¢éãããšãéåžžãåææ¥ã«åºè·ã®å€ãã¯ããã«å°ãªããªããŸãé±ã®æ®ãããã ããšãã°ããã£ãŒã1ã«2é±éã瀺ãããŠããŸããäžè¬çã«ãããŒããŒåç¶ã®Vasyaã¯åææ¥ã®æ©ãæéã«å®¶ãåºãŠãµãã«ãŒã芳æŠããŠããŸãããã®ããã7æ¥éã®å£ç¯æ§ãæã€ãã€ã¯ããµã€ã¯ã«ã®ååšãããã«èæ ®ããå¿ èŠããããŸãã ãšããã§ãåã®èšäºã®ããã«é±ééã«ããŒã¿ã転éããã®ã§ã¯ãªããæ¯æ¥ã®ããŒã¿ãåŒãç¶ã䜿çšããŸãã
å³è¡š1
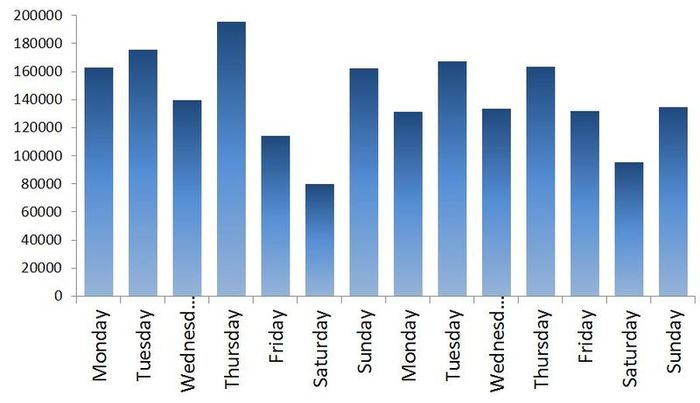
2.ç¶æ 空éã¢ãŒã
ããã§ãæå°éã®çè«ããã詳现ã«ããã¹ãŠã®åŒãç±æ¥ããçç±ãšå Žæãæ¬ã§èªãããšãã§ããããã³ã¡ã³ãã§çããŸãã
ããæç³»åããããšããŸããã


ã©ãã§





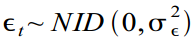
ãã¬ã³ãã¯ãããŒã«ã«ã®ç·åœ¢ãã¬ã³ãã®ã¢ãã«ãšããŠæ§ç¯ããããšã«ãã倿§åã§ããŸãã

ïŒ2ïŒã¯ãã¬ã³ãèªäœã§ãããïŒ3ïŒã¯ãã¬ã³ãã¹ããŒãã§ãããããããã«ãšã©ãŒããããŸãã ãã®ãããªã¢ãã«ã¯ãããªããã©ã³ãã ãŠã©ãŒã¯ããçµ±åã©ã³ãã ãŠã©ãŒã¯ã¢ãã«ãŸã§ããã¬ã³ãã¢ããªã³ã°ã«å€ãã®ãªãã·ã§ã³ãæäŸããŸãã å€ãã®èšéçµæžåŠè ã¯ãïŒ2ïŒã®ãšã©ãŒãé€å»ããŠãæ»ããã§æ»ãããªãã¬ã³ããååŸãããã¹ãŠã®ã©ã³ãã ãšã©ãŒããã¬ã³ãã¹ããŒãã«å²ãåœãŠãŸãã
確ççãµã€ã¯ã«ã¯ãäžè§é¢æ°ãšãããã®å°é¢æ°ã®åèšãšããŠã¢ãã«åãããŸãïŒ

ã©ãã§

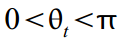


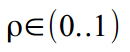
ãããŠæåŸã«ãåšæs = 7ã®é±æ¬¡ãã€ã¯ããµã€ã¯ã«ããäžè§é¢æ°ã®åèšã«åºã¥ããŠæ§ç¯ãããŸãã

çŸåšãäžèšã®ãã¹ãŠã®åŒããã«ã«ãã³ãã£ã«ã¿ãŒã«é©ããããããæ§é 圢åŒã«æŽçããå¿ èŠããããŸãã
枬å®åŒã¯ã芳å¯ããããŒã¿ã瀺ããŠããŸãã

ããã³é·ç§»æ¹çšåŒ-æ§æãã倿°ã®ãã€ããã¯ã¹ã説æããŸã


ç§ãã¡ã®å Žåãæ¹çšåŒïŒ2ïŒ-ïŒ6ïŒã§å®çŸ©ããã10ã®æœåšå€æ°ããããŸãã

次ã«ãäžèšã®ãã¹ãŠã®åŒãè¡å圢åŒã«å€æããŸãã
ïŒ7ïŒã®é·ç§»è¡åïŒ

ïŒ8ïŒã®æœåšå€æ°ã®ãã€ããã¯ã¹ã®ãã®ãããªåŒ±ããããªãã¯ã¹ïŒ
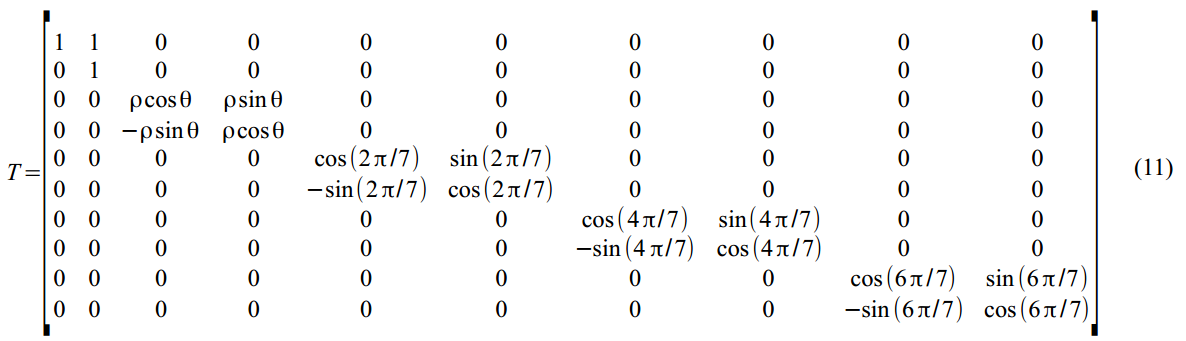
ãã¹ãŠã®ç¶æ ã®ãã¹ãŠã®ãšã©ãŒã®ãã¯ãã«ïŒ2ïŒ-ïŒ6ïŒïŒ

è¡åã䜿çšããŠãã€ããã¯ã¹ã®æ¹çšåŒïŒ8ïŒã«çµã¿èŸŒãŸããŠããŸã
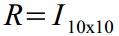
ãããŠæåŸã«ãïŒ8ïŒã®ãã¹ãŠã®ãšã©ãŒãšæåã®ããªãšãŒã·ã§ã³ã®ãããªãã¯ã¹ïŒ
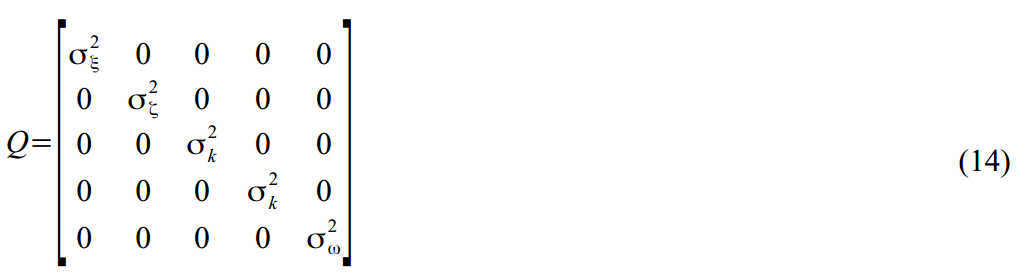
3.ãã£ã«ã¿ãŒãšã«ã«ãã³ã¹ã ãŒã¶ãŒ
ããŠãããã§ã«ã«ãã³ãåžãæºåãã§ããŸããã 倿°æå®ã®





åæå€ãä»®å®ãã

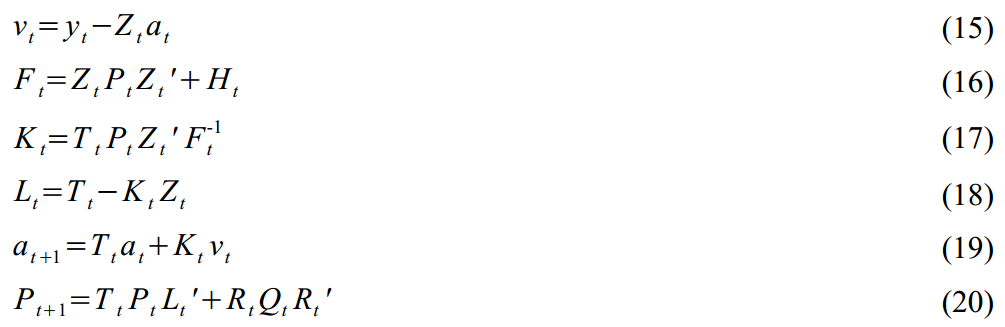
ã©ãã§



ç¶æ ãã¯ãã«ã®èšç®ã«å ããŠããšã©ãŒã®å€åããµã€ã¯ã«åšæ³¢æ°ããµã€ã¯ã«æžè¡°ãã©ã¡ãŒã¿ãŒãªã©ã®åã ã®ã¢ãã«ãã©ã¡ãŒã¿ãŒãèŠã€ããããšã«ãé¢å¿ããããŸãã ç®çã®ãã©ã¡ãŒã¿ãŒã®ãã¯ãã«ã次ã®ããã«åŒã³åºããŸã




以æ¥



ãã®é¢æ°ãæå€§åããããšã«ãããå¿ èŠãªãã©ã¡ãŒã¿ãŒã®æšå®å€ãèŠã€ããããšãã§ããŸã

ããŠãããäžã€ã®wunderwaffeã«ã€ããŠã®ããã€ãã®èšè-Kalman smootherïŒKalman smootherïŒã[DurbinãJ. and Koopmanã2003]ãããã¯ãŸã Habréã§èšåãããŠããªãããã§ãã äžè¬ã«ãèãæ¹ã¯äŒŒãŠãããã«ã«ãã³ãã£ã«ã¿ãŒã®ã¿ãåŸç¶ã®åå€ãèšç®ããŸã



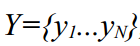
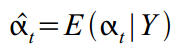
ã«ã«ãã³å¹³æ»åã¯éååž°ã§ãã
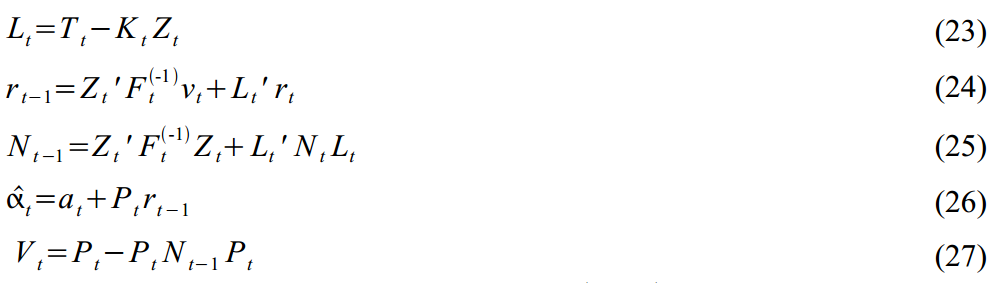
ã¯ãå¹³æ»åãããç¶æ å€ã®ãã¯ãã«ã§ã
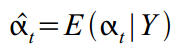
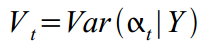





äžè¬çã«ãçè«ã«ã€ããŠã¯é·ãéæžãããšãã§ããŸããããã¹ãããã«ã¯æãããããªããŸãã ããã§ã¯ãå®èšŒçãªå¯ç©è«ã«ç§»ããŸãããã
4.ååž°
ç§ã¯Matlabaã®ä»¥äžã®ããã°ã©ã ã®æé©æ§ãšé床ã®ãµããããŸãããç§ã¯æ¬åœã®æº¶æ¥å·¥ã§ã¯ãããŸããããã¹ãŠã®ã³ãŒãã¯èªåã®ããã«æžãããŸããã 審çŸçã§ã¯ãªãããå®äŸ¡ã§ä¿¡é Œæ§ãé«ãå®çšçã ãŸãããã£ã«ã¿ãŒããã³ã¹ã ãŒãžã³ã°ã®ã³ãŒãã¯ãããŸããŸãªåæåãªãã·ã§ã³ãªã©ã®ããã«èšè¿°ãããŠãããããããã¶ãåé·ã§ãã ã³ãŒãã®äžéšãæšãŠãããšãããšããããªãã¯ã¹ãšã«ãŒãã§Matlabãã¯ã©ãã·ã¥ããããšãé¿ããããªãã£ãããããã¹ãŠããã®ãŸãŸã«ããŠããããšã«ããŸããã
ãã¹ãŠã次ã®ããã«æ©èœããŸãã
- ã¡ã€ã³ããã°ã©ã otgr_ssm.mã¯ããŒã¿ãããŠã³ããŒããã ssmoptæ§é ãæºåããŸãã ãã®æ§é ã«ã¯ãã³ãŒãå ã®ããŸããŸãªå Žæã«éä¿¡ãããå€ãã®è²Žéãªã¡ã¢ãšéèŠãªå€æ°ãæžã蟌ãŸããŸãã åºè·ã®æåŸã®70ã®å€ïŒ10é±éïŒã¯ãäºæž¬ãšã®æ¯èŒã®ããã«ç¢ºä¿ãããŸããäºæž¬ã¯ãæåŸã«å¿ ãäœæãããŸãã
- ããŒã¿ã¯å¯Ÿæ°å°€åºŠé¢æ°æå€§åã«ãŒãã³ã«ã¢ããããŒããããŸãã åŸè ã®æé ã¯èšéçµæžåŠã§ãã䜿çšããããããã¡ã€ã³ã³ãŒããæ£ããããªãããã«ãå¥ã®é¢æ°mle_my.mã«ãã£ãŠäžè¬çãªåœ¢åŒã§ãã°ããèã®äžã«æžãããŸããã
- æ±ãããããã©ã¡ãŒã¿ãŒã«ã¯ç¶æ
ã®åæ£ãå«ãŸããããããããã¯å³å¯ã«æ£ã§ãªããã°ãªãããæ°å€æé©åäžã«åžžã«ååŸããããšã¯éããŸããã ãããã£ãŠãããŒã³ãã¡ã¬ãã«å€æŽãããšããã¹ãŠã®å
¥å忣å€ã¯æ¬¡ã®ããã«å€æãããŸã
ãããã³æžè¡°ä¿æ°ã¯ç¯å²ã§æ£èŠåãããŸã
ã©ããã£ãŠ
ã ããŠãåºåã§ã¯ããããã¯é©åãªå€ã«å€æãããŸã-
ããªãšãŒã·ã§ã³ãš
æžè¡°ä¿æ°çšã å€ssmopt.transã¯ã倿ããå¿ èŠããããïŒ1ïŒããªããïŒ0ïŒãã©ã®æ¹åïŒãinããŸãã¯ãoutãïŒã瀺ããã瀺ããŸãã ããã¯ãã¹ãŠãå¥ã®transform.m颿°ã§çºçããŸã
- mle_my.mã¯ã kfmy2.mã䜿çšããŠã«ã«ãã³ãã£ã«ã¿ãŒïŒ15ïŒ-ïŒ22ïŒãå®è¡ãããªããžã§ã¯ã颿°f_obj.mãåŒã³åºãã察æ°å°€åºŠé¢æ°ïŒ22ïŒã®å€ãèšç®ãã2åèµ·ããªãããã«ã«ã«ãã³ã¹ã ãŒã¶ãŒïŒ23 ïŒ-ïŒ27ïŒ ksmy2.mã䜿çšããŸã ã ããã¯ãã¹ãŠmle_my.mã«æ»ã ã颿°ã®æå€§å€ïŒ22ïŒã«éãããã©ããããã§ãã¯ããŸãã ããå Žåã¯ããã¹ãŠæé 5ã«é²ã¿ãŸãããªãå Žåã¯ãæé 2ã4ãç¹°ãè¿ããŸãã
æåã®ãã£ã«ã¿ãŒã®å®è¡ã¯ã次ã®ä»®å®ããå§ãŸããŸããã å€ãã®å€æ°ãããã®ã§ãããã§è©ŠããŠã¿ãŠãã ããã6次å 颿°ã®ã°ããŒãã«ãªæå€§å€ãæ¢ããŠãããããå€ãã®å±æçãªæ¥µå€ãå¯èœã§ãã è¯ãæ¹æ³ã§ã¯ã察æ°é¢æ°ã®å¯èœãªå€ã®ã°ã©ããäœæããå¯èœãªæ¥µå€ãèŠãããšãã§ããŸãã
- çµæ-èŠã€ãã£ããã©ã¡ãŒã¿ãŒãšã°ã©ãã衚瀺ããŸãã åæã«ã70æ¥éã®äºæž¬ãèšç®ãã frcst.mã䜿çšããŠå®éã®ããŒã¿ãšæ¯èŒããŸã
å®éã«ã¯ãã³ãŒãïŒ
otgr_ssm.m
clear all; clc; close all; format long; %------------------- 1. Load and prepare data ------------------------------ load otgruzka.mat; % Structure ssmopt contains several important records used throughout the code ssmopt.frcst=70; % forecast length yend=y(end-ssmopt.frcst+1:end); % saved last observation for the forecast comparison y=y(1:end-ssmopt.frcst); ssmopt.N=length(y); ssmopt.trans=1; % transform estimated parameters to preserve positiveness of variances ssmopt.sv=[5e+8;500;5e+8;5e+8;0.05;0.9]; % starting values ssmopt.mle='f_obj'; % name of the objective function for the maximization ssmopt.sv=transform(ssmopt.sv,'in',ssmopt); ssmopt.filter='kfmy2'; % name of the function computing Kalman Filter ssmopt.smooth='ksmy2'; % name of the function computing Kalman Smoother %------------------- 2. Estimate the model ------------------------------ result=mle_my(y,ssmopt); % call Maximum Likelihood maximization function b=transform(result.b,'out',ssmopt); % transform parameters back % recompute data based on the correct non-transformed parameters ssmopt.trans=0; ssmopt.sv=b; [LH,KF_out,Ksm_out] = feval(ssmopt.mle,b,y, ssmopt); % Recover filtered states series - trend, cycle, and seasonal a_trend=KF_out.Afilt(1,:); a_cycle=KF_out.Afilt(3,:); a_seas=KF_out.Afilt(5,:)+KF_out.Afilt(7,:)+KF_out.Afilt(9,:); y_filt=a_trend+a_cycle+a_seas; % build the estimated filtered series Y % Recover smoothed states series - trend, cycle, and seasonal a_trendsm=Ksm_out.Asm(1,:); a_cyclesm=Ksm_out.Asm(3,:); a_seassm=Ksm_out.Asm(5,:)+Ksm_out.Asm(7,:)+Ksm_out.Asm(9,:); y_smooth=a_trendsm+a_cyclesm+a_seassm; % build the estimated smoothed series Y result=mle_my(y,ssmopt); % find correct Hessian for non-transformed parameters %------------------- 3. Compute estimation statistics ------------------------------ %Find standard errors, and p-values se=sqrt(abs(diag(inv(result.hessian)/ssmopt.N))); % se(b) tstat=b./se; % t-statistics pval=2*(1-tcdf(abs(tstat),ssmopt.N-length(ssmopt.sv))); % p-value % Display output fprintf('Estimated parameters and p-values:\n'); out=[b pval] period=2*pi/b(end-1) % Compute R-squared resid=y-y_filt; % estimation errors SSE=resid*resid'; % Sum of Squared Errors SST=(y-mean(y))*(y-mean(y))'; % Sum of Squares Total R2=1-SSE/SST % R-squared' %------------------- 4. Make Forecast ------------------------------ af0=KF_out.Afilt(:,end-ssmopt.frcst); [yf,af]=frcst(b,y,ssmopt, af0); %------------------- 5. Plot results ------------------------------ %p=ssmopt.N; p=600; t=[1:1:p]; figure(1) plot(t,y(1:p),'k',t,y_filt(1:p),'b',t,y_smooth(1:p),'r--') title('Original, Filtered, and Smoothed data') legend('y(t)','y filtered','y smoothed'); figure(2) plot(t,y(1:p),'k',t,a_trend(1:p),'b',t,a_trendsm(1:p),'r--') title('Original data, Filtered and Smoothed trend') legend('y(t)','Filtered trend','Smoothed trend'); figure(3) plot(t,a_cycle(1:p),'b',t,a_cyclesm(1:p),'r--') title('Filtered and Smoothed cycle') legend('Filtered cycle','Smoothed cycle'); figure(4) % Filtered + smoothed seasonal plot(t,a_seas(1:p),'b',t,a_seassm(1:p),'r--') title('Filtered and Smoothed weekly seasonal') legend('Filtered seasonal','Smoothed seasonal'); t=[1:1:ssmopt.frcst]; figure(5) plot(t,yend,'b',t,yf,'r--') title('Original data and Forecast') legend('Original data','Forecast'); RMSE=sqrt(sum((yend - yf).^2)/ssmopt.frcst) % Root Mean Squared Error
mle_my.m
function result_mle=mle_my(y,mleopt); warning off; %---------------- 1. Set-up minimization options ---------------- options=optimset('fminunc'); options=optimset('LargeScale', 'off' , ... 'HessUpdate', 'bfgs' , ... 'LineSearchType', 'quadcubic' , ... 'GradObj' , 'off' , ... 'Display','off' , ... 'MaxIter' , 1000 , ... 'TolX', 1e-12 , ... 'TolFun' , 1e-12, ... 'DerivativeCheck' , 'off' , ... 'Diagnostics' , 'off' , ... 'MaxFunEvals', 1000); %---------------- 2. Run minimization ---------------- [b,fval,exitflag,output,grad,hessian]=fminunc(mleopt.mle,mleopt.sv,options,y,mleopt); warning on; result_mle.b=b; result_mle.fval=fval; result_mle.output=output; result_mle.hessian=hessian;
f_obj.m
function [obj,KF_out,Ksm_out]=f_obj_tr(b,y,ssmopt); %---------------- 1. Recover parameters ------------------------------------ b=transform(b,'out',ssmopt); s2_irr=b(1); s2_tr=b(2); s2_cyc=b(3); s2_seas=b(4); freq=b(5); rho=b(6); %---------------- 2. Build the model ------------------------------------ ssmopt.ssmodel.states=10; ssmopt.ssmodel.Z=[1 0 1 0 1 0 1 0 1 0]; T1 = [1 1 0 0; 0 1 0 0; 0 0 rho*cos(freq) rho*sin(freq); 0 0 -rho*sin(freq) rho*cos(freq)]; T2=[cos(2*pi/7) sin(2*pi/7) 0 0 0 0;... -sin(2*pi/7) cos(2*pi/7) 0 0 0 0;... 0 0 cos(4*pi/7) sin(4*pi/7) 0 0;... 0 0 -sin(4*pi/7) cos(4*pi/7) 0 0;... 0 0 0 0 cos(6*pi/7) sin(6*pi/7);... 0 0 0 0 -sin(6*pi/7) cos(6*pi/7)]; ssmopt.ssmodel.T = [T1 zeros(rows(T1),cols(T2));zeros(rows(T2),cols(T1)) T2]; ssmopt.ssmodel.R=eye(10); ssmopt.ssmodel.R(1,1)=0; H=s2_irr; Q=zeros(10,10); Q(2,2)=s2_tr; Q(3,3)=s2_cyc; Q(4,4)=s2_cyc; Q(5,5)=s2_seas; Q(6,6)=s2_seas; Q(7,7)=s2_seas; Q(8,8)=s2_seas; Q(9,9)=s2_seas; Q(10,10)=s2_seas; %---------------- 3. Suggest starting conditions for the states ------------------------ a0=[y(1);0;0;0;0;0;0;0;0;0]; P0=eye(ssmopt.ssmodel.states)*1e+10; %---------------- 4. Run Kalman filter ------------------------ KF_out = feval(ssmopt.filter,y, ssmopt, Q, H, a0, P0); obj=KF_out.LH; %---------------- 5. Run Kalman smoother ------------------------ if nargout>2 ssmopt.ssmodel.num_etas=3; % number of the state variances (required for Kalman smoother) Ksm_out = feval(ssmopt.smooth,KF_out, ssmopt); end
kfmy2.m
% Kalman filter % y[t] = Z*alpha[t] + eps, eps ~ N(0,H). % alpha[t] = T*alpha[t-1] + R*eta, eta ~ N(0,Q). % v[t]=y[t]-E(y[t]) = y[t]-Z*a[t] % F[t]=var(v[t]) function KF_out = kfmy_koop(y, ssmopt, Q, H, a, P); N=ssmopt.N; m=ssmopt.ssmodel.states; %---------------- 1. Recover parameters and prepare matrices ---------------- T=ssmopt.ssmodel.T; Z=ssmopt.ssmodel.Z; R=ssmopt.ssmodel.R; KF_out.Vmat=zeros(1,N); KF_out.Fmat=zeros(1,N); KF_out.Afilt=zeros(m,N); KF_out.Pfilt=zeros(m,m,N); KF_out.Kmat=zeros(m,N); KF_out.Lmat=zeros(m,m,N); LHmat=zeros(1,N); if ~isfield(ssmopt,'exactcheck'); ssmopt.exactcheck=1; % set exact filter initialization by default end; %---------------- 2. Set default starting values for a and P , if none provided ---------------- Pinf=eye(m); if nargin < 6 if ssmopt.exactcheck==1 P=zeros(m,m); else P=eye(m)*1000000000; end end if nargin < 5 a=[y(1); zeros(m-1,1)]; end KF_out.Afilt(:,1)= a; KF_out.Pfilt(:,:,1) = P; d=0; %---------------- 3. Exact Filtering ---------------- if ssmopt.exactcheck==1 evals=10; % number of time steps to evaluate until Pinf converges to zero KF_out.exact.F1=zeros(1,evals); KF_out.exact.F2=zeros(1,evals); KF_out.exact.L1=zeros(m,m,evals); KF_out.exact.Pinf=zeros(m,m,evals); KF_out.exact.Pinf(:,:,1)=Pinf; for i=1:evals if sum(sum(Pinf))<1e-20; d=i-1; % time point after which Pinf-->0, and after which we may start regular Kalman filter break; else if sum(Pinf*Z')>0 % Pinf is not singular F1=inv(Z*Pinf*Z'); F2=-F1*(Z*P*Z'+H)*F1; K=T*Pinf*Z'*F1; K1=T*(P*Z'*F1+Pinf*Z'*F2); L=TK*Z; L1=-K1*Z; P=T*Pinf*L1' + T*P*L' + R*Q*R'; Pinf=T*Pinf*L'; else F1=Z*P*Z'+H; F2=F1; K=T*P*Z'/F1; L=TK*Z; L1=L; P=T*P*L' + R*Q*R'; Pinf=T*Pinf*T'; end v=y(i) - Z*a; a=T*a+K*v; %save filtered estimates KF_out.Afilt(:,i+1)=a; KF_out.Pfilt(:,:,i+1)=P; KF_out.Vmat(i)=v; KF_out.Fmat(i)=F1; KF_out.Kmat(:,i)=K; KF_out.Lmat(:,:,i)=L; LHmat(i) = -0.5*(log(2*pi*F1) + v^2/F1); %save exact values for smoother KF_out.exact.F1(i)=F1; KF_out.exact.F2(i)=F2; KF_out.exact.L1(:,:,i)=L1; KF_out.exact.Pinf(:,:,i+1)=Pinf; end end end %---------------- 4. Regular Filtering ---------------- for i=d+1:N v=y(i) - Z*a; f=Z*P*Z' + H; K=T*P*Z'/f; L=TK*Z; a=T*a+K*v; P=T*P*L'+R*Q*R'; if i<N KF_out.Afilt(:,i+1)=a; KF_out.Pfilt(:,:,i+1)=P; end KF_out.Vmat(i)=v; KF_out.Fmat(i)=f; KF_out.Kmat(:,i)=K; KF_out.Lmat(:,:,i)=L; LHmat(i) = -0.5*(log(2*pi*f) + v^2/f); end %---------------- 5. Prepare output ---------------- KF_out.LH=-sum(LHmat); KF_out.Q=Q; KF_out.H=H; KF_out.exact.d=d; end
ksmy2.m
function [Ksm_out, Kdism_out] = ksmy2(KF_out, ssmopt); [m,N]=size(KF_out.Afilt); meta=ssmopt.ssmodel.num_etas; %---------------- 1. Recover filtered matrices ---------------- Fmat=KF_out.Fmat; Vmat=KF_out.Vmat; Pfilt=KF_out.Pfilt; Afilt=KF_out.Afilt; Q=KF_out.Q; H=KF_out.H; %---------------- 2. Recover Model structure ---------------- Z=ssmopt.ssmodel.Z; T=ssmopt.ssmodel.T; R=ssmopt.ssmodel.R; Asm=zeros(m,N); Psm=zeros(m,m,N); rmat=zeros(m,N); Nmat=zeros(m,m,N); Eps=zeros(1,N); Eta=zeros(meta,N); Kmat=KF_out.Kmat; Lmat=KF_out.Lmat; if ~isfield(KF_out,'exact'); KF_out.exact.d=0; end d=KF_out.exact.d; if KF_out.exact.d>0 L1=KF_out.exact.L1; F1=KF_out.exact.F1; F2=KF_out.exact.F2; Pinf=KF_out.exact.Pinf; end %---------------- 3. Regular Smoothing for t=N..d+1 observations ---------------- for i=N:-1:d+1 r=Z'/Fmat(i)*Vmat(i) + Lmat(:,:,i)'*rmat(:,i); N=Z'/Fmat(i)*Z + Lmat(:,:,i)'*Nmat(:,:,i)*Lmat(:,:,i); Asm(:,i)=Afilt(:,i) + Pfilt(:,:,i)*r; Psm(:,:,i)=Pfilt(:,:,i)-Pfilt(:,:,i)*N*Pfilt(:,:,i); if i>1 rmat(:,i-1)=r; Nmat(:,:,i-1)=N; end if nargout>1 Eps(i)=H*(1/(Fmat(i))*Vmat(i)-Kmat(:,i)'*rmat(:,i)); Eta(:,i)=Q*R'*rmat(:,i); end end %---------------- 4. Exact Smoothing for t=d..1 observations ---------------- if KF_out.exact.d>0 r1=zeros(m,1); N1=zeros(m,m); N2=zeros(m,m); for i=d:-1:1 if sum(Pinf(:,:,i)*Z')>0 %cond(Pinf)<1e+12 % Pinf is not singular r1=Z'*F1(i)*Vmat(i) + Lmat(:,:,i)'*r1 + L1(:,:,i)'*rmat(:,i); N2=Z'*F2(i)*Z + Lmat(:,:,i)'*N2*Lmat(:,:,i) + Lmat(:,:,i)'*N1*L1(:,:,i) + L1(:,:,i)'*N1*Lmat(:,:,i) + L1(:,:,i)'*Nmat(:,:,i)*L1(:,:,i); N1=Z'*F1(i)*Z + Lmat(:,:,i)'*N1*Lmat(:,:,i) + L1(:,:,i)'*Nmat(:,:,i)*Lmat(:,:,i); r=Lmat(:,:,i)'*r1; N=Lmat(:,:,i)'*Nmat(:,:,i)*Lmat(:,:,i); if nargout>1 Eps(i)=-H*Kmat(:,i)'*rmat(:,i); Eta(:,i)=Q*R'*rmat(:,i); end else % Pinf is singular r1=T'*rmat(:,i); N2=T'*N2*T; N1=T'*N1*Lmat(:,:,i); r=Z'/(Fmat(i))*Vmat(i) + Lmat(:,:,i)'*rmat(:,i); N=Z'/(Fmat(i))*Z + Lmat(:,:,i)'*Nmat(:,:,i)*Lmat(:,:,i); if nargout>1 Eps(i)=H*(1/Fmat(i)*Vmat(i) - Kmat(:,i)'*rmat(:,i)); Eta(:,i)=Q*R'*rmat(:,i); end end if i>1 rmat(:,i-1)=r; Nmat(:,:,i-1)=N; end Asm(:,i)=Afilt(:,i) + Pfilt(:,:,i)*r + Pinf(:,:,i)*r1; Psm(:,:,i)=Pfilt(:,:,i)-Pfilt(:,:,i)*N*Pfilt(:,:,i) - (Pinf(:,:,i)*N1*Pfilt(:,:,i))' - Pinf(:,:,i)*N1*Pfilt(:,:,i) - Pinf(:,:,i)*N2*Pinf(:,:,i); end end %---------------- 5. Prepare output ---------------- Ksm_out.Asm=Asm; Ksm_out.Psm=Psm; Ksm_out.Kmat=Kmat; Ksm_out.Lmat=Lmat; Ksm_out.Nmat=Nmat; Ksm_out.rmat=rmat; Kdism_out.Eps=Eps; Kdism_out.Eta=Eta;
transform.m
function b=transform(b,howto,ssmopt); k=length(b); if strcmp(howto,'in') % in-transformation if ssmopt.trans==0 % no transformation b=b; end; if ssmopt.trans==1 % transformation to preserve the positiveness of variances b(1:k-1,:)=log(b(1:k-1,:)); b(k)=log(1/b(k)-1); end; else % out-transformation if ssmopt.trans==0 % no transformation b=b; end; if ssmopt.trans==1 b(1:k-1,:)=exp(b(1:k-1,:)); b(k)=1/(1+exp(b(k))); end; end
5.çµæ
ïŒèŠã€ãã£ãè©äŸ¡ã®på€ã¯æ¬åŒ§å ã«ç€ºãããŠããŸãïŒ
芳枬ãããç³»åã®èª€å·®ã®åæ£ ![]() | 1.77E + 009ïŒ0.00ïŒ |
ãã¬ã³ããšã©ãŒåæ£ ![]() | 348.73ïŒ0.00ïŒ |
ãµã€ã¯ã«åæ£ ![]() | 6.07E + 008ïŒ0.00ïŒ |
å£ç¯æå誀差ã®åæ£ ![]() | 3.91E + 006ïŒ0.00ïŒ |
ãµã€ã¯ã«é »åºŠ ![]() | 3.91E + 006ïŒ0.00ïŒ |
ãµã€ã¯ã«æéïŒæ¥æ°ïŒ | 362.6ïŒ0.00ïŒ |
ã«ãŒãæžè¡°ä¿æ° ![]() | 0.891ïŒ0.00ïŒ |
Räºä¹ååž° | 0.78 |
6.ãã£ãŒã
ãããããããããããã°ã©ãã¯æåã®600æ¥éã®ã¿è¡šç€ºãããã·ãªãŒãºå šäœã§ã¯ãã¿ãã¬ã®äžã«é ããŠããŸãã
aã çããã£ã«ã¿ãŒãããã³å¹³æ»åãããããŒã¿
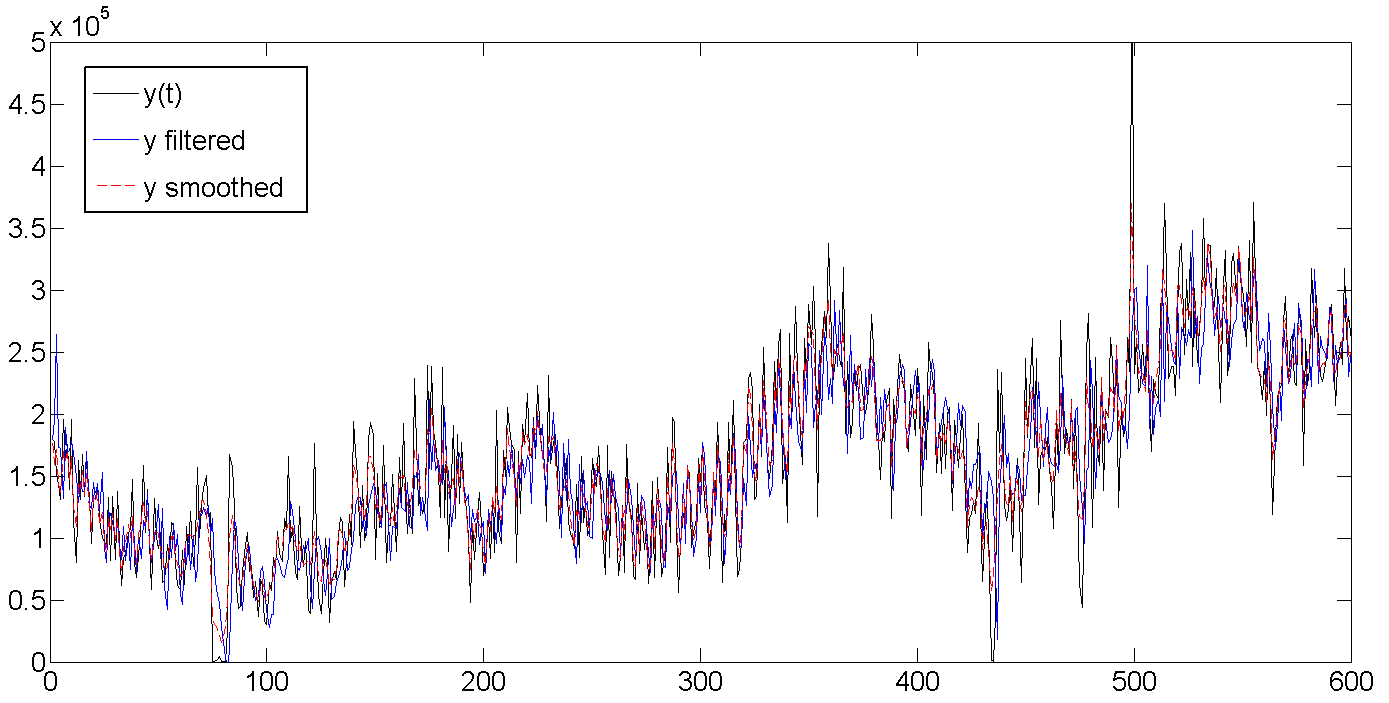
è¡å
šäœ
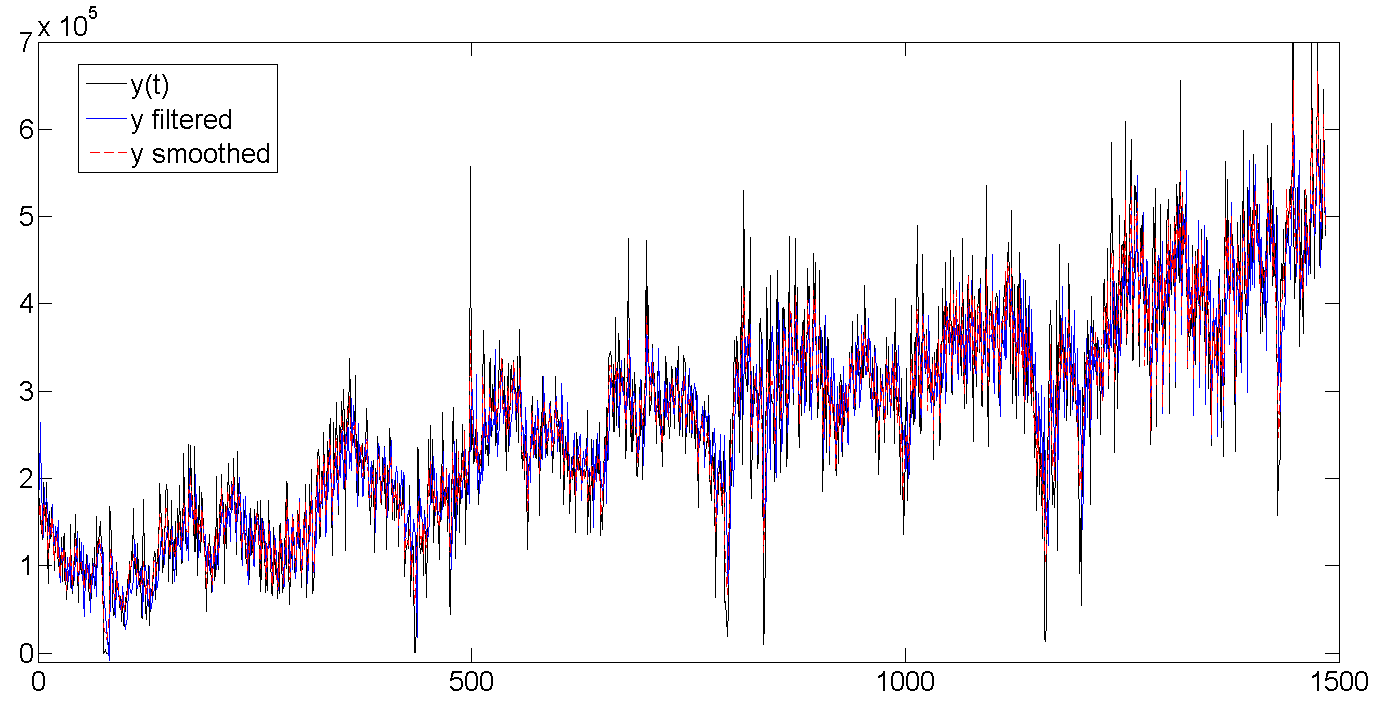
bã ãœãŒã¹ããŒã¿ããã£ã«ã¿ãŒåŠçãããåŸåãå¹³æ»åãããåŸå
ã芧ã®ããã«ãã«ã«ãã³ãã£ã«ã¿ãŒã¯ä»¥åã®å€ã«åºã¥ããŠåŸåãæšæž¬ããããšããŠãããããé¢ä¿è ã®ã©ã€ã³ã«åãããŠå€åããŸãããããŒã¿ãããã«é²ãå Žæãæšæž¬ããããšããŠå°ãé ããŸãã Kalmanã¯ã·ãªãŒãºå šäœãããã¹ã ãŒãºã«ãèŠããããããã¬ã³ãã¯ããæ»ããã§èœã¡çããããã«èŠããŸãã

è¡å
šäœ
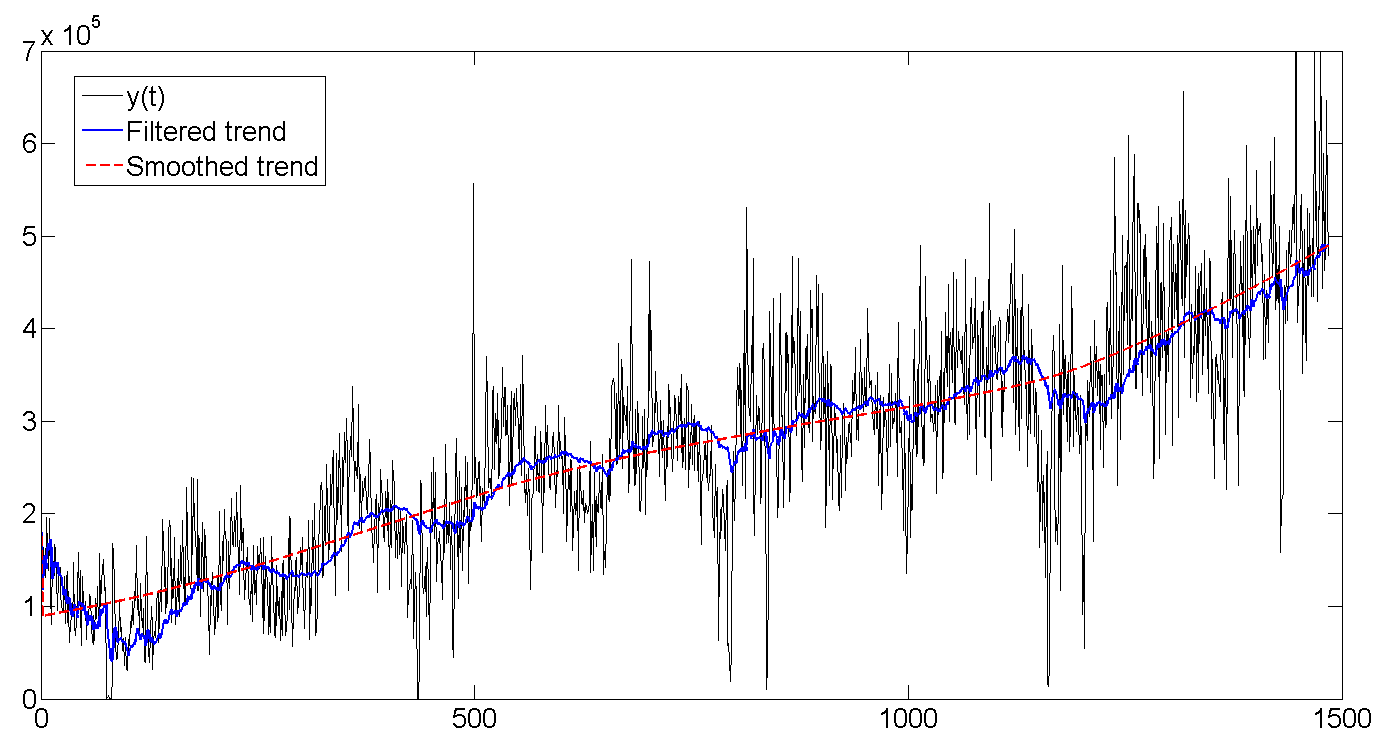
cã ãã£ã«ã¿ãŒåŠçããã³å¹³æ»åãããã«ãŒã
çµæã®è¡šãããããããã«ãå¹³åãµã€ã¯ã«ã®é·ãã¯çŽ362æ¥ããŸãã¯ã»ãŒ1幎ã§ãïŒã ããé©ãã§ãããïŒã ãŸããæœåšå€æ°ã®åæå€ããŒãã«èšå®ãã1e + 10ã®ãªãŒããŒã®éåžžã«å€§ããªåæ£ãèšå®ããããããã£ã«ã¿ãŒãæåã«ã©ã®ããã«èª¿æŽãéå§ããããŒã¿ãå®å šã«å€±ãããèŠãããšãã§ããŸãã ãã ããéåžžããã£ã«ã¿ãŒããªãºã ã«åãããã«ã¯ãæåã®æ°åã®è©Šè¡ã§ååã§ãã ãšããã§ããã®äœæ¥ã§ã¯ããã£ã«ã¿ãŒã®æ£ç¢ºãªåæåïŒæ£ç¢ºãªåæåïŒã䜿çšããŸãããããã«ããããã£ã«ã¿ãŒåŠçãããå€ãããŒã¿ããã°ããååŸã§ããããã«ãªããŸãã

è¡å
šäœ
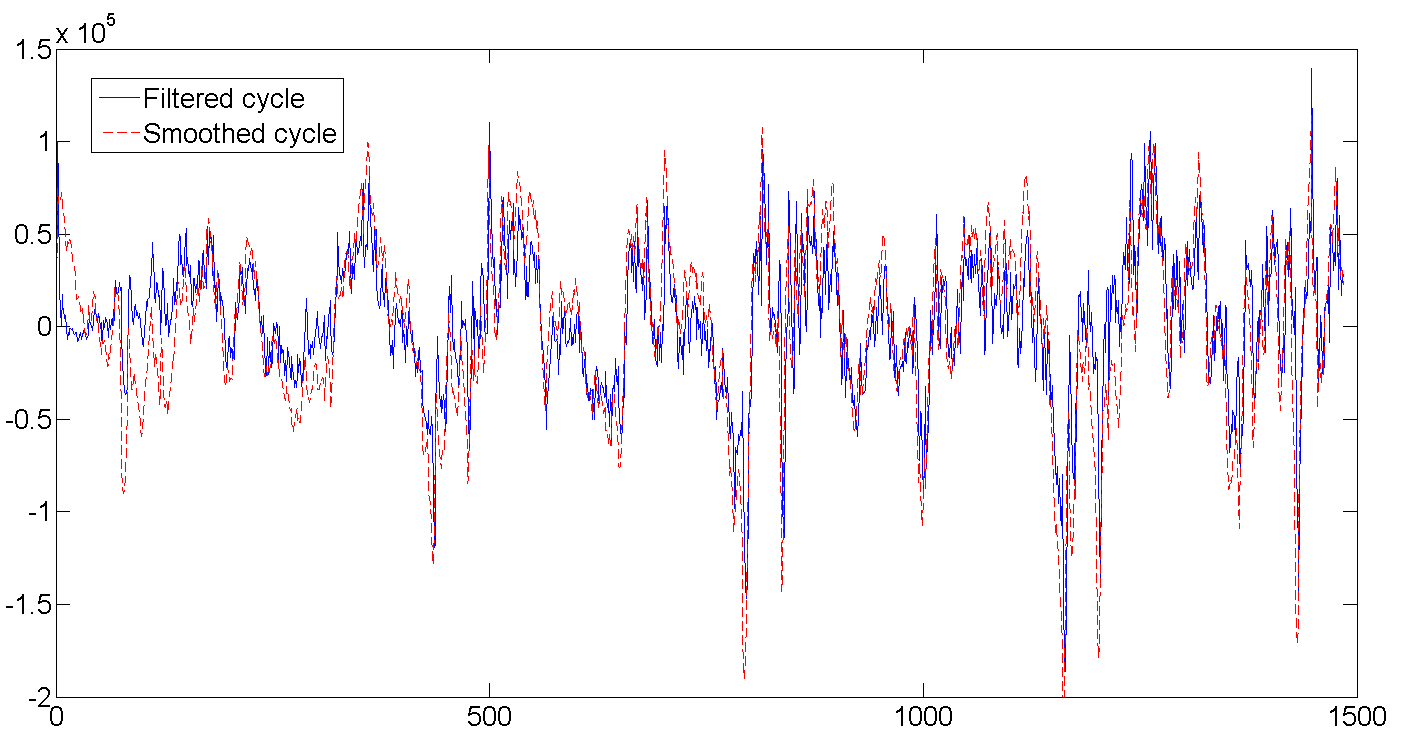
dã ãã£ã«ã¿ãŒåŠçããã³å¹³æ»åãããæ¯é±ã®å£ç¯èŠå
åºè·æ°ã¯åŸã ã«å¢å ããŠãããæ¯æ¥ã®ãã©ãã£ãªãã£ã¯å¢å ããŠããŸãã
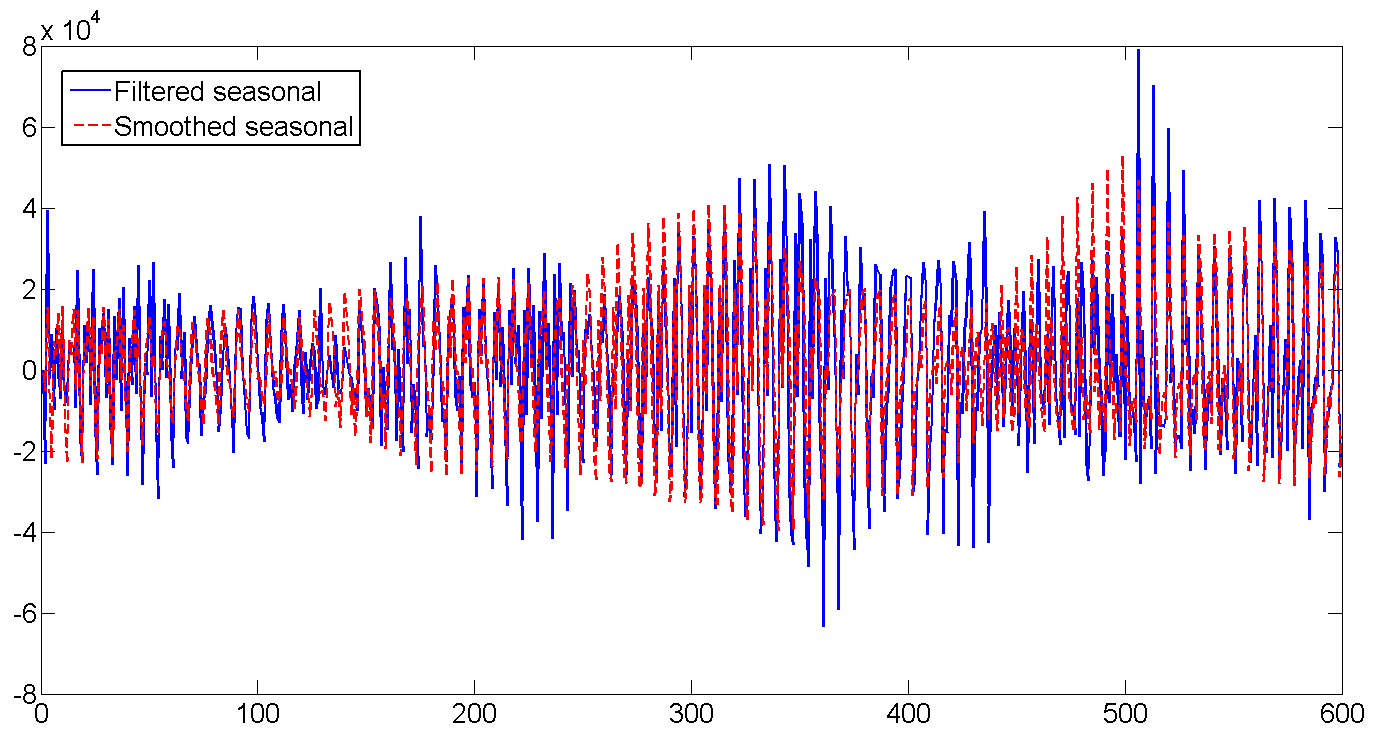
è¡å
šäœ
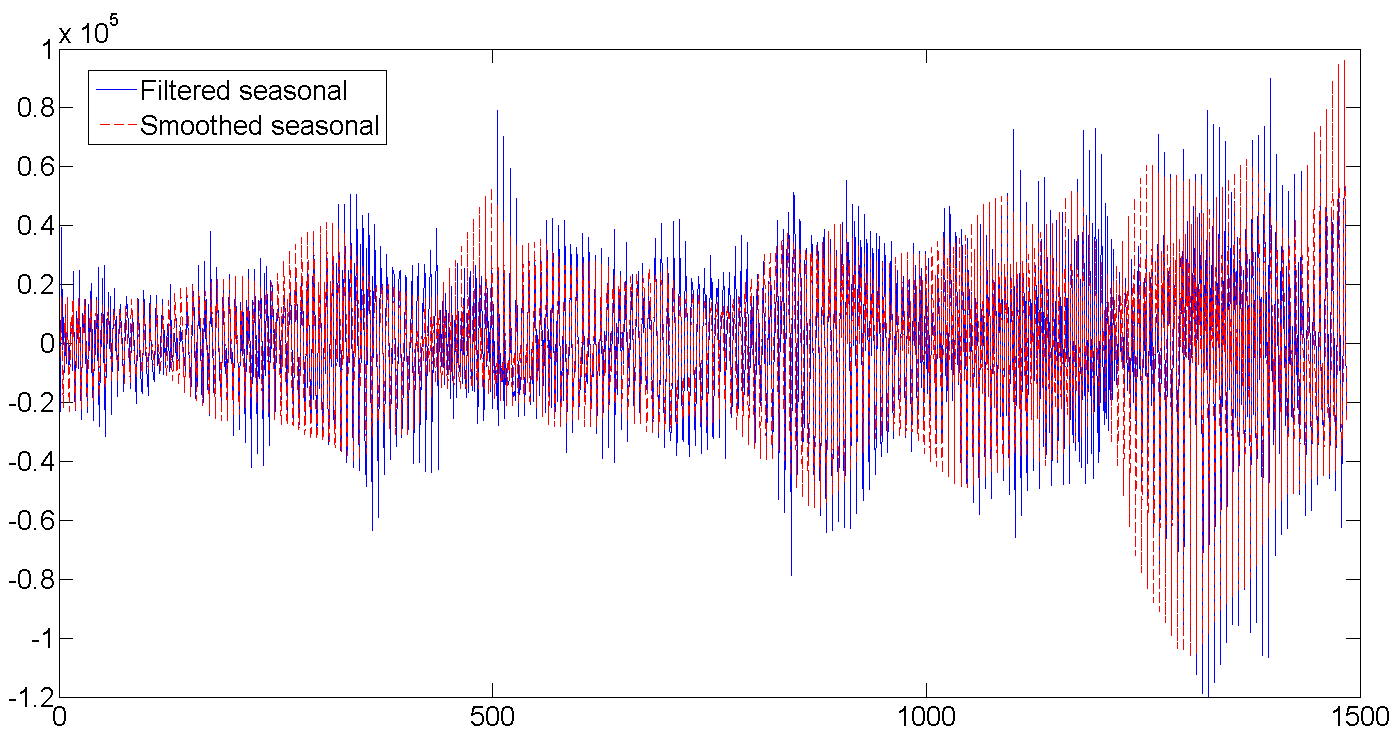
6.äºæž¬
ååŸãããã©ã¡ãŒã¿ãŒã«åºã¥ããŠããã£ã«ã¿ãŒåŠçãããç¶æ ã®ææ°ã®å€ã䜿çšããŠã70æ¥éïŒ10é±éïŒã®äºæž¬ãäœæããæ¢åã®ããŒã¿ãšæ¯èŒããŸãã äžè¬çã«ãäºæž¬ã¯èŠæ ããããããããçåœãäžãããã£ã«ã¿ãŒã®åœ¹å²ã§ãã ææ¥ããšã®ãã©ãã£ãªãã£ã®æšæž¬ã¯ç¹ã«åã°ããããšã§ãã æ§ç¯ãããäºæž¬ã®å šäœã®é·ããããèŠãŠãæ³ååãæå¹ã«ãããšã幎éåºè·ãµã€ã¯ã«ã®äžã§äºæž¬ãã©ã®ããã«æ²ããããèŠãããšãã§ããŸãã äºæž¬ãæ©èœããªãã£ãå¯äžã®ç¬éã¯26ã32æ¥ã§ãã ããããæããã«åºè·ãã»ãŒæ¯é±äœäžãããã®åŸããã«æ¥æ¿ãªãžã£ã³ããçºçããŸããããã®ãããªã±ãŒã¹ã¯ã·ãªãŒãºã®æåã«äžåºŠããçºçããªãã£ããããäºæž¬ããããšã¯ã»ãšãã©äžå¯èœã§ããã äžè¬çã«ãèœã¡ãã®ã¯æãããã§ãã
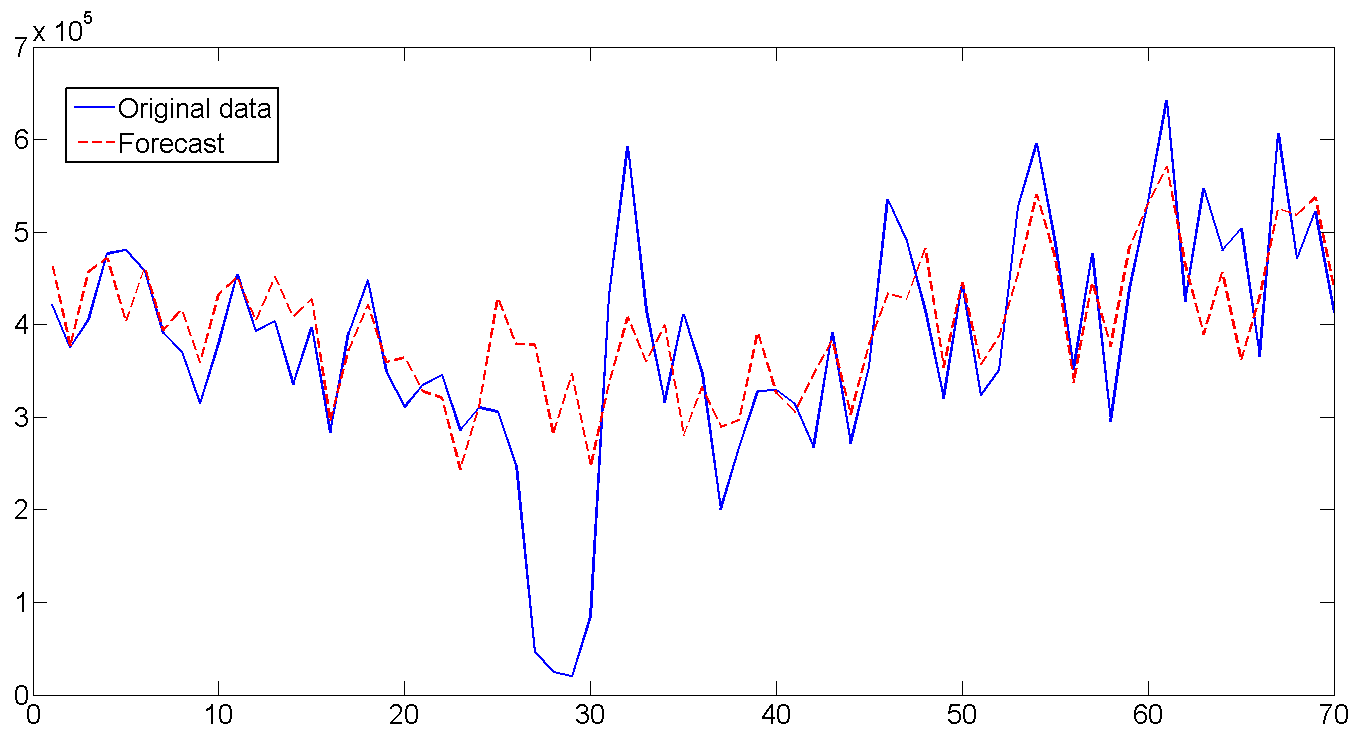
ã¢ãã«ãæ¯èŒããå Žåãäºæž¬RMSEã¯1.112e + 005ã§ãã
ãŸããããã ãã§ãã
ãæ³šæ
ç¶æ
空éã¢ãã«ã¯ãèšéçµæžåŠã«ãããŠäŸ¡å€ã®ãããã®ãšèŠãªãããã¹ãã§ã¯ãããŸããã ããã©ãããããããã¯ããå€ãã®ç¹å®ã®ã¢ãã«ã®äžè¬åãããããŒãžã§ã³ã衚ããŸãã ããšãã°ãMAïŒ1ïŒããã»ã¹
SSM圢åŒã§ç°¡åã«æ³åã§ããŸãã
ãŸãã¯ARMAïŒ2,1ïŒããã»ã¹ïŒ
SSM圢åŒã§ããã¯ïŒ

SSM圢åŒã§ç°¡åã«æ³åã§ããŸãã

ãŸãã¯ARMAïŒ2,1ïŒããã»ã¹ïŒ

SSM圢åŒã§ããã¯ïŒ

é¢é£æç®
- DurbinãJããããã³Koopmanãç¶æ ç©ºéæ³ã«ããSJæç³»ååæã ãªãã¯ã¹ãã©ãŒãïŒãªãã¯ã¹ãã©ãŒã倧åŠåºçå±ã2001ã
- DurbinãJããããã³KoopmanãSJãç¶æ 空éæç³»ååæã®ããã®ããã·ã³ãã«ã§å¹ççãªã·ãã¥ã¬ãŒã·ã§ã³ãBiometrika volã 89ã第3å·ã2002幎ã
- HarveyãACãããã³JaegerãAãããã¬ã³ãé€å»ãå®ååãããäºå®ããã³ããžãã¹ãµã€ã¯ã«ããJournal of Applied EconometricsïŒ8ïŒã1993ã
- HarveyãACãäºæž¬ãæ§é æç³»åã¢ãã«ãããã³ã«ã«ãã³ãã£ã«ã¿ãŒã ã±ã³ããªããžïŒã±ã³ããªããžå€§åŠåºçå±ã1989幎ã