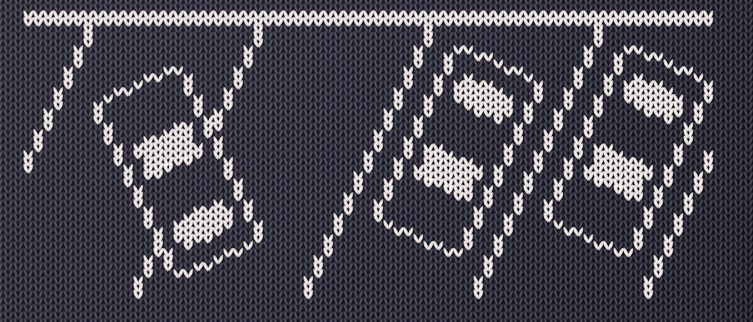
Evaluating whether selfish or altruistic drivers are around is important.
Imagine trying to turn left onto a busy road. Machine after machine pass by, keeping you trapped, your tension rises. Finally, the generous driver slows down enough to miss you. Checking oncoming traffic, a little acceleration, and you successfully merge into the traffic.
Such scenes are played around the world countless times a day. And this is a situation in which it is difficult to understand the physics of movement and the motives of other drivers, as evidenced by the fact that in the United States 1.4 million accidents occur during bends every year. Now add autonomous cars to this situation. Usually they are limited only to the assessment of physical parameters and make more careful decisions in situations with ambiguous situations.
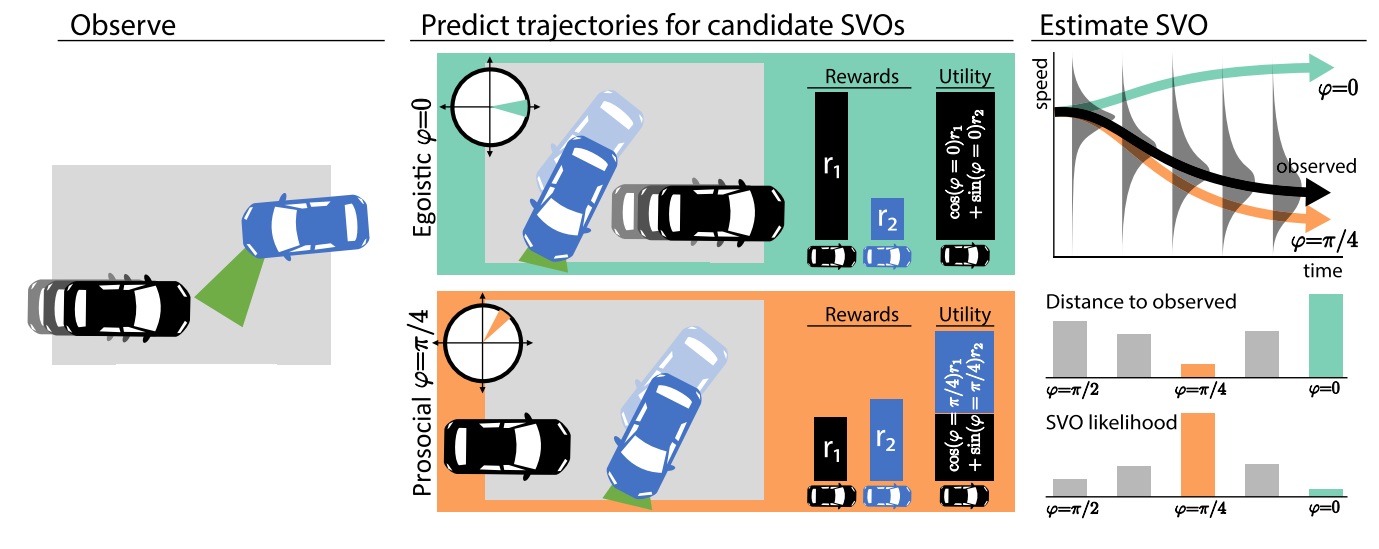
Knowing the "social preferences" of the driver, we can predict his behavior. An autonomous car (blue) observes the trajectory of another driver (black). We can predict the future movement of a black vehicle based on a decision model that maximizes utility (“Driving as a game in a mixed human-robot system”).
Recently, a group of computer scientists have figured out how to improve the performance of an autonomous vehicle under these conditions. Scientists, in fact, partially taught their autonomous cars the theory of mind , which allows vehicles to better interpret the behavior of living drivers who are nearby.
Mind theory
The theory of reason is so close to us that it is sometimes difficult to realize how rarely it is found outside our species. We can easily understand what other people think the same way we do, and we use this tool to evaluate their knowledge and possible motives. We use this tool in most social interactions, including driving. While a friendly wave of the hand from another driver may mean that he is giving way to you, you can often draw conclusions simply based on how he drives the car.
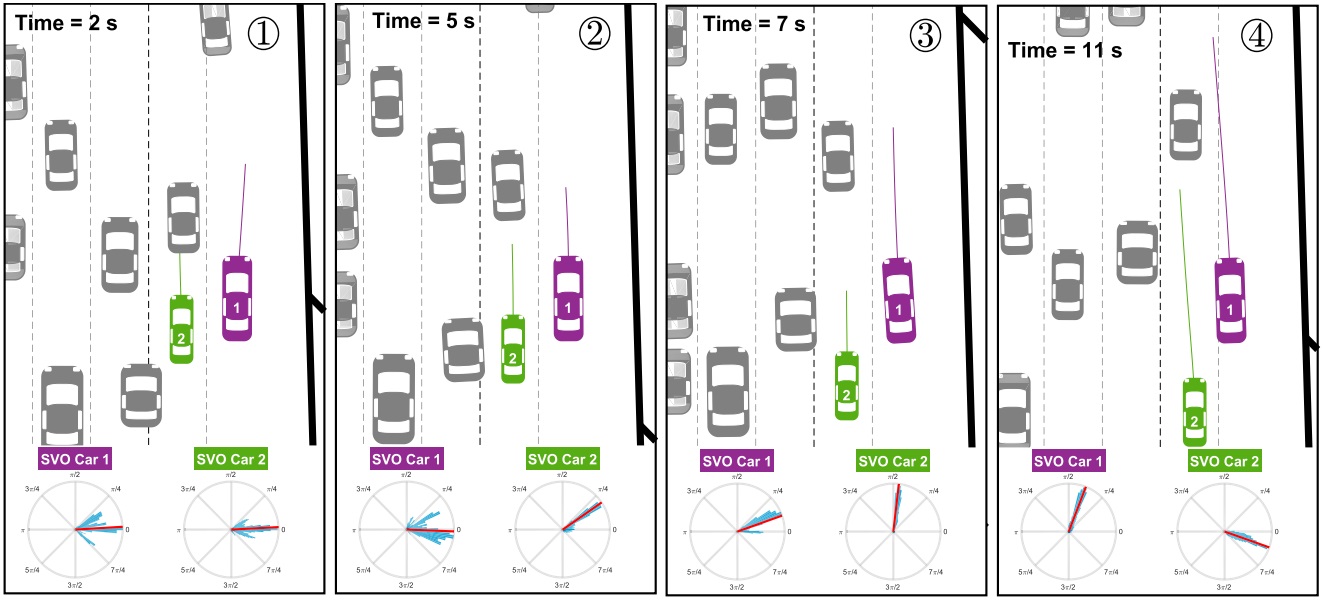
NGSIM dataset with n = 2 active cars (purple and green) and n = 50 “obstacles” (gray). The purple machine is trying to integrate into the stream and should interact with the green machine. The bold line shows the predicted trajectory of the algorithm. Blue indicates distribution, red indicates evaluation.
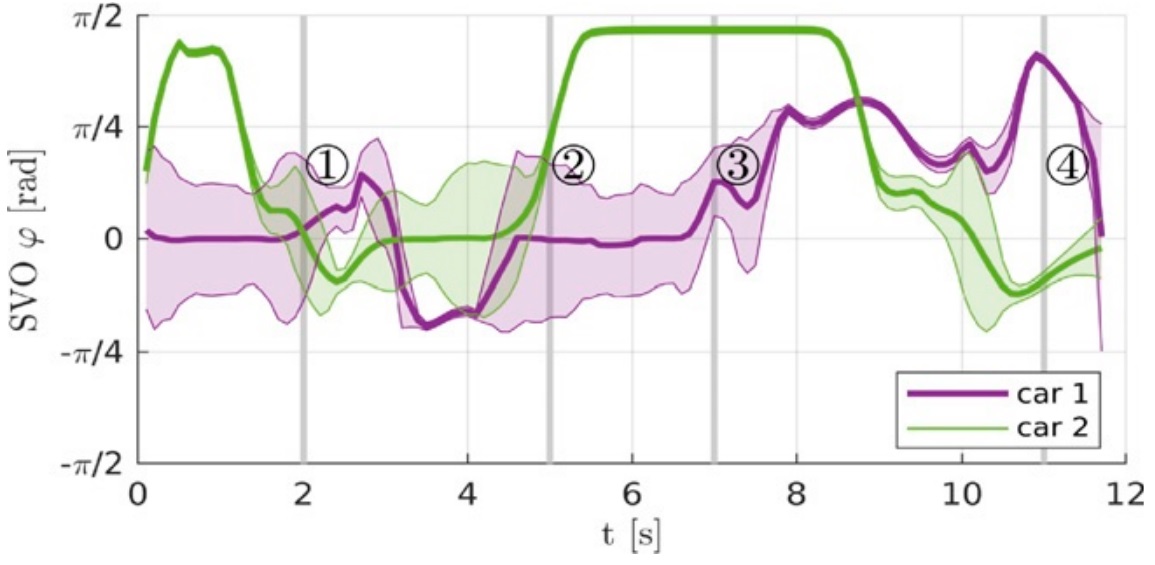
The solid line shows the assessment of "social preferences" in time, and the shaded area shows the confidence limits. Initially, car 2 does not interact with car 1 and does not allow it to integrate into the stream. After a few seconds, car 2 becomes more prosocial, which means “slow down (dropping it back)” and allows the first car to integrate into the stream.
Unfortunately, autonomous cars are not very good at this. In many cases, their behavior does not tell other drivers anything. A study of autonomous car accidents in California showed that in more than half of accidents, autonomous cars crashed into the rear, as human drivers could not understand the actions of cars in automatic control (in particular, Volvo is working to change this )
It is impossible to think that we will be able to fully train autonomous machines of the theory of the mind in the near future. Artificial intelligence is not so developed, and it will be unnecessary for machines that interact only with a limited number of types of human behavior. However, a team of researchers from MIT and Delft University of Technology decided that using a very limited theory of mind is possible to make some decisions on the road, including turns and situations where it is necessary to join the traffic flow.
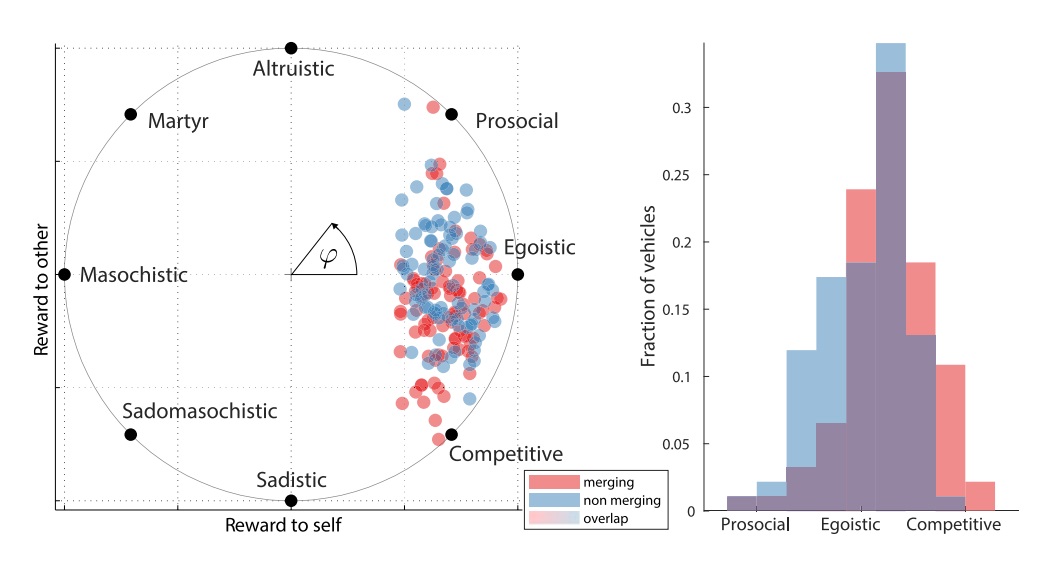
“Social preferences” are presented as an angular preference ϕ, depending on how people distribute the weight / value of the reward / win in the game about the social dilemma.
The idea that underlies the work of researchers is described in a new article in PNAS and includes the concept of orientation to social values , which allows you to evaluate how selfish or altruistic human actions are. And although, of course, there are detailed polls that can give a detailed description of orientation to the social values of a person, autonomous cars usually have no time to conduct polls among drivers around.
Thus, the researchers divided the orientation on social values into 4 categories: altruists who try to be as positive as possible towards other drivers; prosocial drivers who try to act as profitably as possible for everyone else (sometimes it can be selfish acceleration); individualists for whom their own driving experience comes first; and drivers who compete with everyone else in driving skills.
Value Orientation (SVO)
Researchers have derived a formula by which the expected trajectory of movement for each of the categories of drivers can be calculated, taking into account the starting positions of other cars.
Autonomous cars were programmed to compare the trajectories of real drivers with the calculated ones, and use this comparison to determine which of the four categories these drivers most likely belong to. Given this classification, an autonomous machine will be able to design its next steps. As the researchers wrote, “We are expanding the power of reasoning of autonomous cars by combining personality assessments and driving styles of other drivers using social signals.”
This differs significantly from some work in this area related to game theory. These works implied that each driver tries to maximize his profit, altruism is only an accident in this increase. The new work, on the contrary, includes altruistic behavior in its calculations and recognizes that the behavior of drivers is difficult to evaluate, and that they can change their intentions as the situation develops. In fact, previous studies have shown that in situations other than driving, about half of the people studied showed prosocial behavior, while the other 40% were selfish.

(Left) the calculated distribution of SVO preferences of the blue machine is shown as polar histograms in SVO circles during the preparation and implementation of embedding into the stream. (On right)
the average score is shown in red, and reality (80 °, altruist) in black. SVO estimates with 1-σ uncertainty limits are shown on the right. The interaction zone corresponds to the gray area.
After the implementation of the system, researchers obtained data on the location and trajectories of vehicles when drivers poured into the stream of cars on the highway, which often requires the generosity of other drivers. With a system of orientation to social values, the artificial driver was able to make more accurate forecasts of the trajectories of the movement of people-drivers - errors decreased by 25%. The system also worked with lane changes on busy freeways, as well as when cornering into a traffic stream.
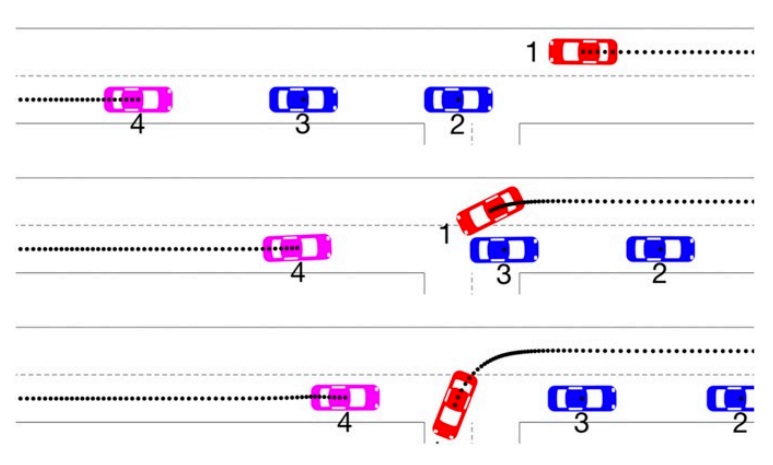
Unsafe left turn of an autonomous car (red; i = 1) with oncoming traffic. When an autonomous car approaches an intersection, two selfish cars (blue; i = 2, 3) continue to move and are not inferior. The third altruistic car (purple; i = 4) yields, slows down, allowing the autonomous car to be embedded.
Using these estimates, researchers could also draw some conclusions from the available traffic patterns. For example, they found that a driver on a highway can begin to selfishly follow a car in front of him, then start acting altruistically, slowing down so that another driver can join the traffic flow, and then return to a selfish approach. Similarly, drivers who encounter a motorway exit usually compete with others - as in situations where you see a car that drives off and slows down everyone who is stuck behind it.
While we are still far from giving autonomous machines full-fledged artificial intelligence or a complete theory of the mind, but studies show that you can get significant benefits from using their limited versions. And this is a great example of the fact that if we want to integrate autonomous systems into social interactions, it can be very important to pay attention to what sociologists have already found out.

About ITELMA
We are a large automotive component company. The company employs about 2500 employees, including 650 engineers.
We are perhaps the most powerful competence center in Russia for the development of automotive electronics in Russia. Now we are actively growing and we have opened many vacancies (about 30, including in the regions), such as a software engineer, design engineer, lead development engineer (DSP programmer), etc.
We have many interesting challenges from automakers and concerns driving the industry. If you want to grow as a specialist and learn from the best, we will be glad to see you in our team. We are also ready to share expertise, the most important thing that happens in automotive. Ask us any questions, we will answer, we will discuss.
We are perhaps the most powerful competence center in Russia for the development of automotive electronics in Russia. Now we are actively growing and we have opened many vacancies (about 30, including in the regions), such as a software engineer, design engineer, lead development engineer (DSP programmer), etc.
We have many interesting challenges from automakers and concerns driving the industry. If you want to grow as a specialist and learn from the best, we will be glad to see you in our team. We are also ready to share expertise, the most important thing that happens in automotive. Ask us any questions, we will answer, we will discuss.
Read more useful articles: