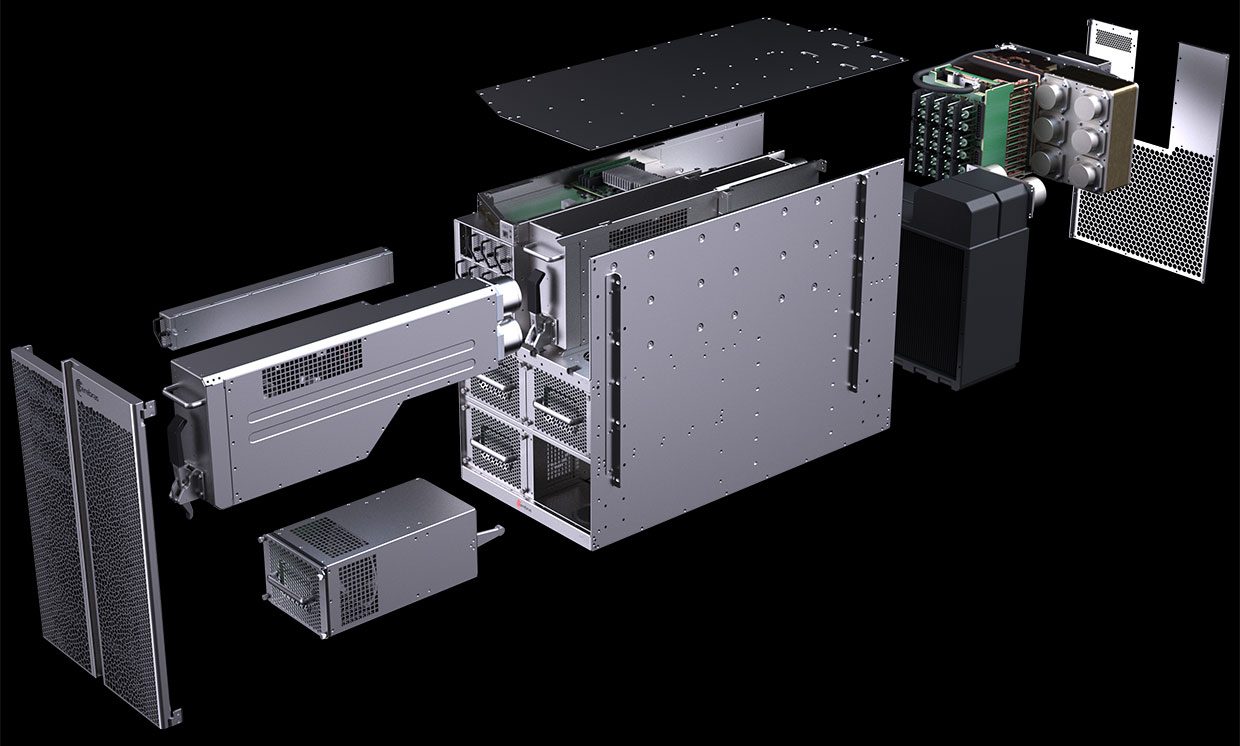
The CS-1 circuit diagram shows that most is dedicated to powering and cooling the giant “processor-on-plate” Wafer Scale Engine (WSE). Photo: Cerebras Systems
In August 2019, Cerebras Systems and its manufacturing partner TSMC announced the largest chip in the history of computer technology . With an area of 46,225 mm² and 1.2 trillion transistors, the Wafer Scale Engine (WSE) chip is approximately 56.7 times larger than the largest GPU (21.1 billion transistors, 815 mm²).
Skeptics said that developing a processor is not the most difficult task. But here is how it will work in a real computer? What is the percentage of defective work? What food and cooling will be required? How much will such a machine cost?
It seems that the engineers at Cerebras Systems and TSMC were able to solve these problems. On November 18, 2019, at the Supercomputing 2019 conference, they officially unveiled the CS-1 , "the world's fastest computer for computing in the field of machine learning and artificial intelligence."
The first copies of CS-1 have already been sent to customers. One of them is installed in the Argonne National Laboratory of the US Department of Energy, the one in which the assembly of the most powerful supercomputer in the USA from Aurora modules on the new Intel GPU architecture will begin. Another customer was the Livermore National Laboratory.
The processor with 400,000 cores is designed for data centers for processing computing in the field of machine learning and artificial intelligence. Cerebras claims that the computer trains AI systems by orders of magnitude more efficiently than existing equipment. Performance CS-1 is equivalent to “hundreds of GPU-based servers” consuming hundreds of kilowatts. At the same time, it occupies only 15 units in the server rack and consumes about 17 kW.

WSE processor. Photo: Cerebras Systems
Andrew Feldman, CEO and co-founder of Cerebras Systems, says the CS-1 is "the world's fastest AI computer." He compared it to Google’s TPU clusters and noted that each of them “takes 10 racks and consumes more than 100 kilowatts to provide a third of the performance of a single CS-1 installation.”
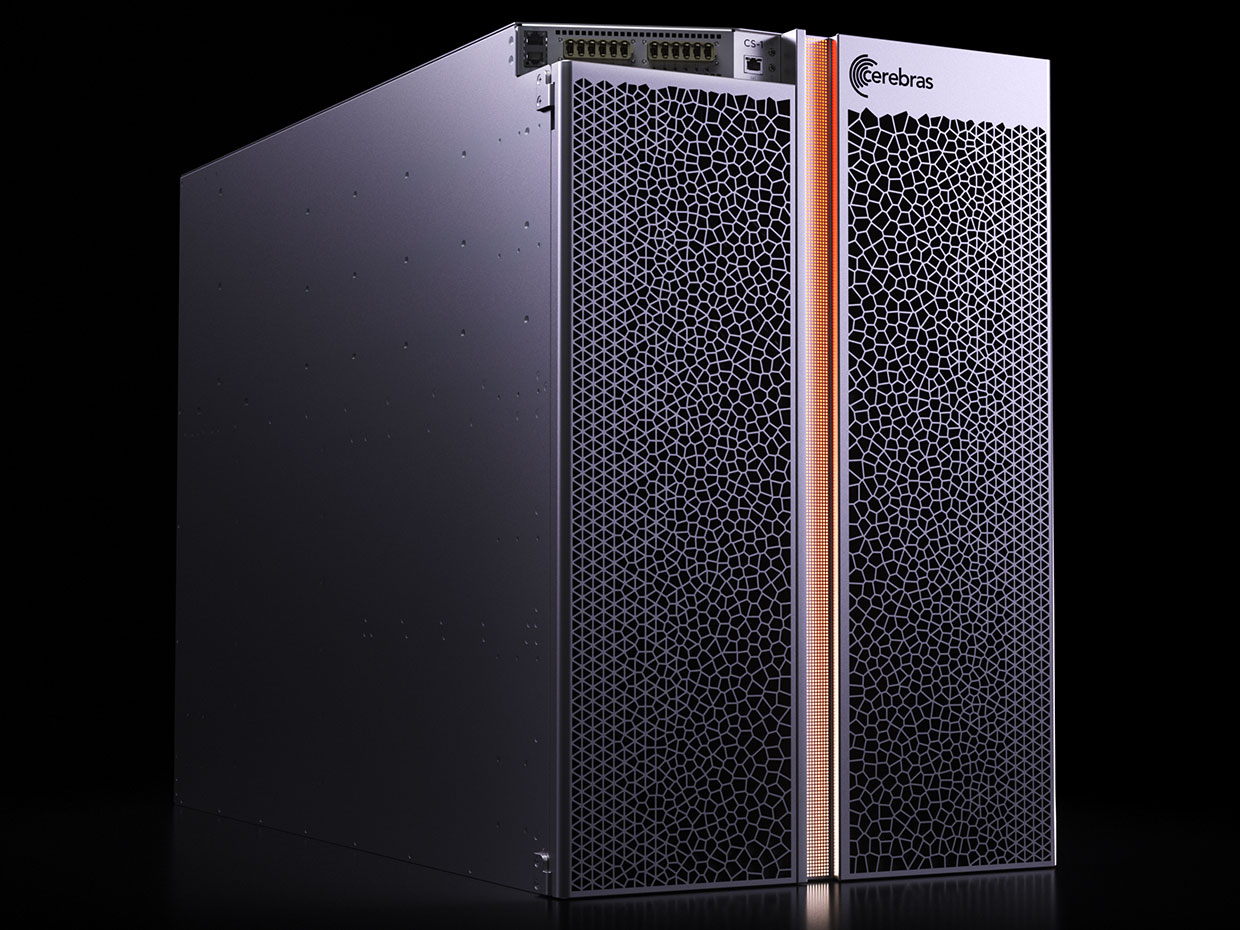
Computer CS-1. Photo: Cerebras Systems
Learning large neural networks can take weeks on a standard computer. Installing a CS-1 with a processor chip of 400,000 cores and 1.2 trillion transistors performs this task in minutes or even seconds, writes IEEE Spectrum. However, Cerebras did not provide real test results to verify high performance statements such as MLPerf tests . Instead, the company directly established contacts with potential customers - and allowed to train its own models of neural networks on CS-1.
This approach is not unusual, analysts say: “Everyone manages their own models that they have developed for their own business,” said Karl Freund , an artificial intelligence analyst at Moor Insights & Strategies. “This is the only thing that matters to customers.”
Many companies are developing specialized chips for AI, including traditional industry representatives such as Intel, Qualcomm, as well as various start-ups in the US, UK and China. Google has developed a chip specifically for neural networks - a tensor processor, or TPU. Several other manufacturers followed suit. AI systems operate in multi-threaded mode, and data transfer between the chips becomes a bottleneck: “Connecting the chips actually slows them down and requires a lot of energy,” explains Subramanian Iyer, a professor at the University of California at Los Angeles who specializes in developing chips for artificial intelligence. Equipment manufacturers are exploring many different options. Some are trying to expand interprocess connections.
Founded three years ago, Cerebras startup, which received more than $ 200 million in venture capital financing, has proposed a new approach. The idea is to save all the data on a giant chip - and thereby speed up the calculations.

The entire microcircuit plate is divided into 400,000 smaller sections (cores), given that some of them will not work. The chip is designed to route around defective areas. The programmable SLAC kernels (Sparse Linear Algebra Cores) are optimized for linear algebra, that is, for calculations in vector space. The company also developed “sparsity harvesting” technology to improve computing performance under sparse workloads (containing zeros), such as deep learning. Vectors and matrices in vector space usually contain a lot of zero elements (from 50% to 98%), so on traditional GPUs, most of the calculations go to waste. In contrast, SLAC cores pre-filter null data.
Communications between the cores is provided by the Swarm system with a throughput of 100 petabits per second. Hardware routing, latency measured in nanoseconds.
The cost of a computer is not called. Independent experts believe that the real price depends on the percentage of marriage. Also, the performance of the chip and how many cores are workable in real samples are not reliably known.
Software
Cerebras has announced some details about the software part of the CS-1 system. The software enables users to create their own machine learning models using standard frameworks such as PyTorch and TensorFlow . Then the system distributes 400,000 cores and 18 gigabytes of SRAM memory on the chip into the layers of the neural network so that all layers complete their work at about the same time as their neighbors (optimization task). As a result, information is processed by all layers without delay. With a 12-port 100-gigabit Ethernet I / O subsystem, the CS-1 can process 1.2 terabits of data per second.
The conversion of the source neural network to an optimized executable representation (Cerebras Linear Algebra Intermediate Representation, CLAIR) is done by the Cerebras Graph Compiler (CGC). The compiler allocates computing resources and memory for each part of the graph, and then compares them with the computing array. Then, the communication path is calculated according to the internal structure of the plate, unique to each network.
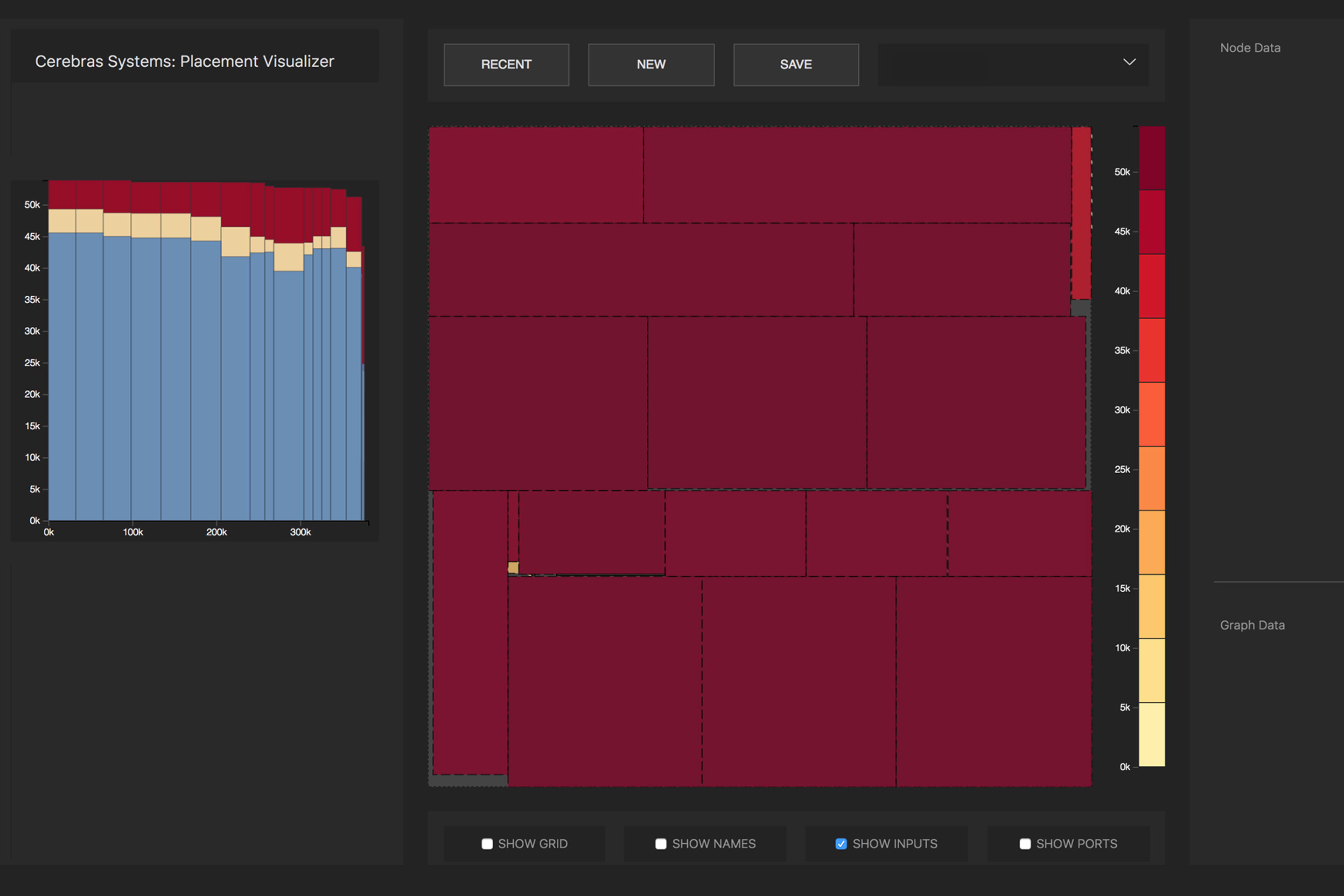
Distribution of mathematical operations of a neural network by processor cores. Photo : Cerebras
Due to the huge size of WSE, all layers in a neural network are simultaneously located on it and work in parallel. This approach is unique to WSE - no other device has enough internal memory to fit all layers on one chip at once, says Cerebras. Such an architecture with the placement of the entire neural network on a chip provides huge advantages due to its high throughput and low latency.
The software can perform the optimization task for multiple computers, allowing the cluster of computers to act as one large machine. A cluster of 32 CS-1 computers shows an approximately 32-fold increase in performance, which indicates very good scalability. Feldman says that this is different from the behavior of clusters based on GPUs: “Today, when you make a cluster of GPUs, it does not behave like one big machine. You get a lot of small cars. ”
The press release said that the Argonne National Laboratory has been working with Cerebras for two years: "By deploying CS-1, we dramatically increased the speed of training of neural networks, which allowed us to increase the productivity of our research and achieve significant success."
One of the first loads for CS-1 will be a neural network simulation of a collision of black holes and gravitational waves, which are created as a result of this collision. The previous version of this task worked on 1024 of 4392 nodes of the Theta supercomputer.