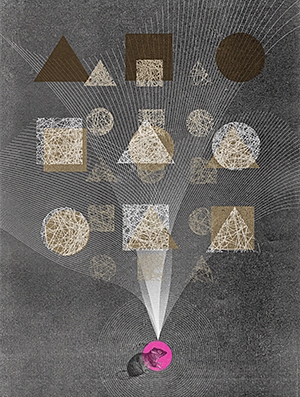
Today's systems with artificial intelligence can crush people champions in such difficult games as chess, go and
Texas Hold'em . In flight simulators, they can shoot down the best pilots. They excel human doctors in creating accurate surgical stitches and making cancer diagnoses. But in some cases, a three-year-old child will easily furnish the best AI in the world: when the competition is associated with training, so routine that people do not even know about it.
David Cox [David Cox], a neurobiologist from Harvard, an AI expert, the proud father of a three-year-old daughter, came up with such a thought when she, seeing a long-legged skeleton in a museum of national history, pointed a finger at him and said: "Camel!" a camel happened several months before when her father showed her a painted camel in a picture book.
AI researchers call this ability to identify an object by a single example of “learning at a time,” and they terribly envy such abilities of karapuz. Today's AI systems are trained in a completely different way. According to the autonomous learning system called “deep learning”, the program is given an array of data from which to draw conclusions. To train the AI that recognizes camels, the system must digest thousands of images of camels — drawings, anatomical diagrams, photos of single-humped and two-humped camels — all images marked “camel.” AI will also require thousands of other pictures, marked “not a camel”. And when he has lived through all this data and determined the distinctive features of the animal, he will become an excellent determinant of camels. But Cox's daughter by then would have time to move on to giraffes and platypuses.
Cox mentioned his daughter, explaining the US state program called Machine Intelligence on Cortical Networks [Machine Intelligence from Cortical Networks, Microns]. His ambitious goal: to carry out reverse engineering of human intelligence so that programmers can create improved AI. First, neuroscientists need to find out what computational strategies are in the gray matter of the brain; then the data team will translate them into algorithms. One of the main tasks of the final AI will be learning at once. “People have an amazing opportunity to draw conclusions and summarize,” says Cox, “and this is what we are trying to grasp.”
The five-year program, which received funding in the amount of $ 100 million from the
Agency for Advanced Intelligence Research (IARPA), focuses on the visual cortex, a part of the brain that processes visual information. Working with mice and rats, the three Microns teams plan to mark the layout of the neurons in a cubic millimeter of brain tissue. This may not sound so impressive, but this cube contains about 50,000 neurons connected to each other through 500 million connections, synapses. The researchers hope that a clear understanding of all connections will allow them to determine the neural “contours” activated during the work of the visual cortex. The project requires a special neuroimaging system showing individual neurons with a resolution at the level of nanometers, which has not yet been achieved for a brain area of this size.
Although representatives of several institutes work in each Microns team, most of the members of the team working under the direction of Cox, assistant professor of molecular and cellular biology and computer science at Harvard, work in the same building on Harvard territory. During a walk through the laboratory, it is possible to observe rodents engaged in performing tasks in the "game club" for rats; brain slicing machine, like the best automatic sausage cutter; one of the fastest and most powerful microscopes on the planet. With such equipment, working to the fullest, and with huge investments of human forces, Cox believes that they have every chance to break the code of this unfortunate cubic millimeter.
Try to imagine this enormous power of the human brain. To process information about the world and maintain the functioning of the body, electrical impulses travel through 86 billion neurons wedged into spongy tissues inside your skull. Each neuron has a long
axon , curling through this tissue and allowing it to connect with thousands of other neurons, resulting in trillions of connections. The patterns of electrical impulses correlate with all the feelings and sensations of a person: with the movement of a finger, digesting a meal, falling in love or recognizing a camel.
Two-photon laser microscope. An infrared laser scans the brain tissue of a living animal that performs a specific task. When two photons simultaneously strike a neuron, a fluorescent label emits a photon with a different wavelength. A microscope records video with these flashes (above). “You can see how the rat thinks,” says David Cox.
Programmers have been trying to emulate the brain since the 1940s, when they first came up with software structures called artificial neural networks. Most of the best modern AIs use some modern form of this architecture: deep neural networks, convolutional neural networks, neural networks with feedback, etc. These networks, based on the structure of the brain, consist of a set of computational nodes, artificial neurons that perform small specific tasks and are connected to each other so that the whole system can perform impressive things.
Neural networks could not copy the anatomical brain more accurately, since science still does not have basic information about the layout of the nervous system. Jacob Vogelnstein, Microns project manager at IARPA, says that the researchers usually worked on either a microscale or a macroscale. “We used tools that either monitored individual neurons or collected signals from large areas of the brain,” he says. “There is a big gap in understanding operations at the circuit level — how thousands of neurons work together to process information.”
The situation has changed thanks to recent technological breakthroughs that have allowed neuroscientists to construct "
connectom " maps, revealing many connections between neurons. But Microns needs more than just a static link diagram. The team must demonstrate how these bonds are activated when the rodent sees, learns, and recalls. “It’s very similar to how a person tries to understand the operation of an electronic circuit,” said Vogelstein. “You can look at the chip in detail, but you won’t understand what it should do until you see how it works.”
For IARPA, the real result will be obtained if researchers can trace the pattern of neurons involved in recognition and translate it into a more brain-like architecture of artificial neural networks. “I hope that the computational strategies of the brain can be reproduced in terms of mathematics and algorithms,” says Vogelstein. The government puts on the fact that systems with AI, working in a manner similar to the brain, will be able to cope better with real tasks than their predecessors. Of course, understanding how the brain works is a noble task, but intelligence agencies want the AI to quickly learn to recognize not only the camel, but also the half-hidden face in the granular frame of the surveillance camera.
The Game Club for Cox Rats is a small room in which four microwave-sized black boxes are stacked on top of each other. In each box there is a rat facing the screen, and there are two faucets in front of its nose.
At the Argonne National Laboratory, the APS synchrotron accelerates electrons and they cut into the metal filament, producing extremely bright X-rays that focus on a small piece of brain tissue. X-ray images made from many angles are combined to create a three-dimensional image that demonstrates each neuron inside the piece.
Neurons in the brain tissue
In the current experiment, rats are trying to cope with the challenge. The screen shows three-dimensional images created by a computer. These are not some objects from the outside world, just lumpy abstract forms. When a rat sees object A, it must lick the left tap to get a drop of sweet juice. When she sees object B, the juice will be in the right tap. But objects are shown from different angles, so the rat will need to rotate each object in mind and decide whether it belongs to A or B.
Training sessions are diluted in obtaining photographs for which rats are carried along the corridor to another laboratory, where a large microscope is covered with a black cloth and looks like old-fashioned photographic equipment. The team uses a two-photon laser microscope to study the animal's visual cortex when it looks at the screen, where two familiar objects A and B are shown from different angles. The microscope records flashes and luminescence occurring when the laser hits the active neurons, and a three-dimensional video shows patterns resembling green fireflies flashing on a summer night. Cox wants to know how these patterns change when an animal becomes an expert in a given task.
The resolution of the microscope is not good enough to see the axons connecting the neurons to each other. Without this information, scientists do not determine how one neuron activates the next one to create an information processing loop. To do this, the animal needs to be killed, and the brain must be examined more closely.
Researchers cut a tiny cube out of the visual cortex, which FedEx delivers to the Argonne National Laboratory. There, a particle accelerator uses powerful X-rays to build a three-dimensional map showing individual neurons, other types of brain cells and vessels. This map also does not show the associated axions in the cube, but it helps later when researchers compare images from a two-photon microscope with images obtained from electron microscopes. “The x-ray for us is the
Rosetta Stone ,” says Cox.
Then a piece of the brain returns to the Harvard laboratory of Jeff Lichtman, a professor of molecular and cellular biology, a leading expert in brain connectivity. The team of Likhtman takes this cubic millimeter of the brain and cuts it with the help of a machine into 33,000 pieces 30 nm thick. These thinnest sheets are collected on strips of film and placed on a silicon substrate. The researchers then use one of the world's fastest electron microscopes, sending 61 electron beams to each tissue sample, and measuring electron scattering. A machine the size of a refrigerator works around the clock, and produces images of each slice with a resolution of 4 nm.
Each image resembles a section of a cube of tightly packed spaghetti. The software image processing system collects the slices in order and tracks each spaghetti thread from one slice to another, sketching the full axon length of each neuron along with its thousands of connections to other neurons. But the software sometimes loses the thread or confuses one with the other. People are better than computers doing this task, Cox says. “Unfortunately, there are not enough people all over the Earth to process this amount of data.” Harvard and MIT programmers are working on the tracking task that they need to solve to build an accurate diagram of the brain structure.
Putting this diagram on a map of brain activity obtained on a two-photon microscope, it will be possible to detect the computational structures of the brain. For example, such a combination should show which of the neurons in the circuit, lit when the rat sees a strange object, mentally turns it upside down and decides that it looks more like object A.
Another difficult problem facing the Cox team is speed. In the first phase of the project, which ended in May, each team needed to show the results of a study of a piece of brain tissue measuring 100 cubic micrometers. With such a reduced piece, the Cox team completed the stage with electron microscopy and reconstruction in two weeks. In the second phase, teams need to learn how to process pieces of the same size in a few hours. Scaling from 100 μm
3 to 1 mm
3 increases the volume a thousand times. Therefore, Cox is obsessed with automating every step of the process, from training rats with video to tracking a connectom. “These IARPA projects make research look like the work of engineers,” he says. “We need to turn the crank handle very quickly.”
Accelerating experiments allows the Cox team to test more theories about brain structure that will help AI researchers as well. In machine learning, the programmer sets the overall architecture of the neural network, and the program decides how to link the calculations into a sequence. Therefore, researchers plan to train the rats and neural networks on the same visual task and compare the communication schemes and results. “If we notice some patterns in the brain connections, and we don’t notice them in the models, this could be a hint that we are doing something wrong,” says Cox.
One area of research includes brain training rules. It is believed that the recognition of objects occurs through hierarchical processing, in which the first set of neurons receives the primary colors and shape, the next set finds edges to separate the object from the background, and so on. When an animal learns to better cope with the task of recognition, researchers may ask: which of the sets of neurons in the hierarchy changes its activity the most? And when the AI begins to better cope with the same task, does its neural network change in the same way as the rat neural network?
IARPA hopes that the discoveries will apply not only to computer vision, but also to machine learning as a whole. “We all act at random here, but our luck is supported by evidence,” says Cox. He notes that the cerebral cortex, the outer layer of the nervous tissue, in which high-level recognition occurs, has a “suspiciously identical” structure throughout the volume. Such homogeneity makes neuroscientists and experts on AI consider that one fundamental communication scheme can be used in information processing in the brain, which they plan to detect. The definition of such a proto circuit can be a step forward to general-purpose AI.
In the meantime, the Cox team is turning the crank, trying to get the tried and tested procedures to work faster, another researcher from Microns is engaged in a radical idea. If it works, as George Church says, a professor at the Harvard Institute is inspired by the biology of technology. Wissa, she can revolutionize the science of the brain.
Church leads the Microns team with Tai Sing Lee from Carnegie Malone University in Pittsburgh. Church is responsible for the connection layout, and his approach is very different from other commands. He does not use an electron microscope to track axonal connections. He believes that this technology is too slow and produces too many errors. He says that when trying to track axons in a cubic millimeter of tissue, errors will accumulate and contaminate the connective data.
The method of Church does not depend on the length of the axon or the size of the investigated piece of brain. It uses genetically modified mice and a technology called
DNA barcoding , which marks each neuron with a unique genetic identifier that can be read both from the fringe of its dendrites and from the end of its long axon. “It doesn't matter how huge your axon is,” he says. “With barcoding, you find two ends, and how everything is messed up in the middle does not matter.” His team uses slices of brain tissue thicker than the Cox team - 20 µm instead of 30 nm - because they do not need to worry about losing the exact path of the axon between the slices. DNA sequencing machines record all barcodes present in a given slice, and then the program processes lists of genetic information and creates a map showing which neurons are associated with which.
Church and his colleague Anthony Zador, a professor of neuroscience at Cold Spring Harbor Laboratory in New York, have proven in previous experiments that barcoding and sequencing technologies are working, but have not yet collected the data into a solid map of the connection that is needed to work on the Microns project. . If the team manages to do this, Church says that Microns will only be the beginning of his attempts to map the brain: then he wants to build a diagram of all the connections in the whole mouse brain, in which you can find 70 million neurons and 70 billion connections. “Working with a cubic millimeter means being extremely short-sighted,” Church says. “My plans don't end there.”
Brain plot map based on RNA barcoding
Sequencing machine
Such large-scale maps can contribute to the emergence of new ideas for the development of AI, thoroughly emulating the biological brain. , , : , , . « , », – . , , , .
, Microns - . , , , – . « , – , – . – , , ».