泚é
ãã®èšäºã§ã¯ããã¬ãŒãã³ã°ã®ã¢ããªã³ã°ã«åºã¥ããç§åŠæ å ±åŠã®åæéçºã®æ¹æ³è«ã玹ä»ããŸãã 調æ»äžã®ãªããžã§ã¯ãã®åºç€ãšããŠããã«ããã§ãŒãºãã¥ãŒã€ã³ã°ã·ã¹ãã ãæäŸããŠããŸãã PythonãšäžŠåã³ã³ãã¥ãŒãã£ã³ã°ã䜿çšããŠã¢ãã«ãå®è£ ããããã°ã©ã ã³ãŒããšç¢ºççã¢ããªã³ã°ã®çµæãæäŸããŸãã
1.ã¯ããã«ãšèæ¯
ç§ãã¡ã®ç ç©¶ã§ã¯ãç§åŠçããã³å·¥åŠçåé¡ãåæããã³è§£æ±ºããããã®ã³ã³ãã¥ãŒã¿ãŒã®äœ¿çšãšããŠããç§åŠæ å ±åŠããšããçšèªã®æå³ãçè§£ããŠããŸãã åçŽãªæ°å€èšç®ãšåºå¥ããŸãã æè²ã«ãããç§åŠæ å ±åŠã®äœ¿çšã¯ãçåŸãšæåž«ã®äž¡æ¹ã«ãšã£ãŠåžžã«é£ãã課é¡ã§ãã ãã®ãããªåŠç¿ããã»ã¹ã¯ãå€ãã®æè¡çããã³åŠéçãªåé¡ãæ±ããæ°åŠçç¥èãšã³ã³ãã¥ãŒã¿ãŒãµã€ãšã³ã¹ã®åæãå¿ èŠãšããŸãã ãããã®å°é£ãå æããããã«ãæå°ã«å¯Ÿããæ§æäž»çŸ©çã¢ãããŒãã«åºã¥ããŠãããæåž«ã«é©åãªæ§é çåºç€ãæäŸããäžé£ã®æå°ååãšæ¹æ³è«ãæäŸããŸãã ããã«ãããåŠçã¯ã³ã³ãã¥ãŒã¿ã¢ãã«ã䜿çšããŠäžé£ã®èšç®å®éšãè¡ãããšãã§ããŸãã ãã®ã¢ãããŒãã¯æ°åŠãšããã°ã©ãã³ã°ã®ç¥èã«é¢é£ããŠããããããã¯ã¡ã€ã³ãã¬ãŒãã³ã°ã³ãŒã¹ã®éçšã§æãããã坿¥ã«é¢é£ããŠããŸãã èšç®çµ±èšã®ã»ã¯ã·ã§ã³ã¯ãç§åŠæ å ±åŠã®å ¥éã»ã¯ã·ã§ã³ã§ããããã®ç ç©¶ã®å¿çšåéãšããŠèããããŸãã ãã®æ¹æ³è«ã®èæ¯ã以äžã«ç€ºããŸãã
1.1ã ç§åŠæ å ±åŠ
KarniadaxãšKirby IIã¯ããã³ã³ãã¥ãŒã¿ãŒã€ã³ãã©ããã£ã¯ã¹ãæš¡å£ç ç©¶ã®æ žå¿ããšå®çŸ©ããŸããã èè ã¯ããæ°å€ã¢ã«ãŽãªãºã ãææ°ã®ããã°ã©ãã³ã°ææ³ã䞊åèšç®ãžã®å šäœçãªã¢ãããŒããææ¡ããŸããå€ãã®å ŽåãããããæŠå¿µãé¡äŒŒã®ããŒã«ã¯ããŸããŸãªé¢é£ãããã¯ãã³ãŒã¹ãæç§æžã§å®æçã«ç ç©¶ããããããã®é¢ä¿ã¯ããã«æããã«ãªããŸãã ã³ã³ã»ãããšããŒã«ãçµ±åããå¿ èŠæ§ã¯ãéåžžãã³ãŒã¹ãä¿®äºããåŸãããšãã°æåã®å€§åŠé¢ã§ã®äœæ¥äžããŸãã¯è«æã®èŠçŽãæžããšãã«æããã«ãªããããã«ãããåŠçã¯3ã€ã®ç¬ç«ããåéã®çè§£ã1ã€ã«ãŸãšããŠãæãŸãã解決çãåŸãããšãã§ããŸãã ãã®ããã»ã¹ã¯ééããªãéåžžã«äŸ¡å€ããããŸãããå€ãã®æéãèŠããå€ãã®å ŽåãæŠå¿µãšããŒã«ã®å¹æçãªçµã¿åãããæäŸããªãå ŽåããããŸãã æè²åŠã®èгç¹ãããç§åŠæ å ±åŠã®ãããã¯ã®çè§£ãæ·±ããããã«ãç·åçãªçµ±åã¢ãããŒãã¯ãåŠçãäžåºŠã«ããã€ãã®åéã«åºæ¿ããããšãã§ããŸãã å³1ã¯ãæ°å€æ°åŠãæ å ±åŠãã¢ããªã³ã°ã®å ±ééšåãšããŠã®ç§åŠæ å ±åŠã®å®çŸ©ã瀺ããŠããŸã[16]ã

å³ 1.ç§åŠæ å ±åŠã
1.2ã åŠç¿ã«ãããæ§æäž»çŸ©
ã±ã€ã³ãšã±ã€ã³ã®åºç€ç ç©¶[6]ã§ã¯ãåŠç¿ã«ãããæ§æäž»çŸ©ã®åºæ¬ååãææ¡ããŸããã ç§ãã¡ã«ãšã£ãŠæãéèŠãªããšã®1ã€ã¯ããè³ãéšåãšå šäœãåæã«åŠçããããšãã§ãã
ãã®ããã«ãããçµç¹ãããåŠç¿ããã»ã¹ã¯ãåºç€ãšãªã詳现ãšã¢ã€ãã¢ãå®èšŒããŸãã ã¢ããªã³ã°ããŒã¹ã®ã¢ãããŒãã䜿çšãããšãã·ãã¥ã¬ãŒã·ã§ã³ã¢ãã«ãäœæããåŸã調æ»ã®ç®çãæããã«ãªããŸãã ããã«ãããçµæã芳å¯ããé¢é£ããçµè«ãç«ãŠãããšãã§ããŸãã
1.3ã ã¢ããªã³ã°ããŒã¹ã®åŠç¿ïŒãªãã¢ãã«ãªã®ãïŒ
ã®ãã³ãºã¯ã2001幎ã«ã·ãã¥ã¬ãŒã·ã§ã³ããŒã¹ã®ãã¬ãŒãã³ã°ããã°ã©ã ãå°å ¥ããŠããŸã[9]ã æ¬¡ã®åºæ¬ååã匷調ããŸãã
- çåŸã¯ã¢ãã«ãšã®ããåããéããŠçµéšãç©ãã
- åŠçã¯ã¢ãã«ãå®éšããããšã§ç§åŠçããã³å·¥åŠçãªåé¡ã解決ããŸãã
- åé¡ã®æ€èšãšå£°æ;
- ç¹å®ã®æè²ç®æšã®å®çŸ©;
- 決å®ã®æèã«ããããã¹ãŠã®å¿ èŠãªæ å ±ã®æç€ºã
Millard et alã[30]ã¯ããã€ã³ã¿ã©ã¯ãã£ãã¢ããªã³ã°ãã䜿çšããåŠç¿ä¿é²ã¢ãã«ãææ¡ããŠããŸãã èè ã¯ããã·ã¹ãã ãã€ããã¯ã¹ãã«åºã¥ããææãªæ¹æ³è«ãã«åºã¥ãçŸä»£ã®ã³ã³ãã¥ãŒã¿ãŒãã¯ãããžãŒã玹ä»ããŸãã ãå®éã®çµéšã«ã¯ãã€ã³ã¿ã©ã¯ãã£ããªã¢ãã«ã®æ§ç¯ãããã³ãããã䜿çšãã仮説ãšå®éšã®ãã¹ããå«ãŸããŸããã
Lehrer and Schauble [25]ã¯ãã¢ãã«ã®ããŸããŸãªè¡šçŸã䜿çšããå®éšã«çŠç¹ãåœãŠãŠããŸãããåŠçãã¢ãã«ã®ããã€ãã®ããŒãžã§ã³ãäœæããã³ä¿®æ£ãããããã®ããŸããŸãªã¢ãã«ã®èšè¿°ã®åŠ¥åœæ§ãæ¯èŒããæ©äŒããããšãåŠçã®åŠç¿ã匷åãããŸããã
1.4ã æè²ã®äžå¿ã«ããç§åŠæ å ±åŠïŒã¢ãã«ã䜿ã£ãå®éš
Xue [40]ã¯ããç§åŠæ å ±åŠãã«åºã¥ããæè²ã®æ¹é©ããã¢ããªã³ã°ãšæš¡å£ãéããŠææ¡ããŠããŸãã 圌ã¯ã...ã¢ããªã³ã°ãšã·ãã¥ã¬ãŒã·ã§ã³ã䜿çšããŠãããã°ã©ãã³ã°ãã¢ããªã³ã°ãããŒã¿åæã®çŸåšã®åé¡ã解決ãã...ããšã¢ããã€ã¹ããŠããŸãã ã¢ããªã³ã°ããŒã¹ã®åŠç¿ã¯æ°åŠæè²ã§äœ¿çšãããŸãã GeogebraãœãããŠã§ã¢ã䜿çšããŠå€ãã®ã¢ãã«ãæ§ç¯ãããŸãã[33]ã ã¢ãã«ã¯ç§åŠæè²ã§äž»èŠãªåœ¹å²ãæãããŸã[7,18]ã
1.5ã ãã¥ãŒã€ã³ã°ã·ã¹ãã ã®ç¢ºççã¢ããªã³ã°
åæå®çŸ©ã®åçŽããšãã¢ããªã³ã°ãšã·ãã¥ã¬ãŒã·ã§ã³ã®å¹ åºãå¯èœæ§ã®ããã«ããã¥ãŒã€ã³ã°ã·ã¹ãã ã®äœ¿çšãææ¡ããŸãã ãã¹ãµãŒãã¹çè«ã¯ããç¥ãããŠããããã¹ãµãŒãã¹ã·ã¹ãã ïŒQSïŒã®ã·ãã¥ã¬ãŒã·ã§ã³ã¯ãç§åŠ[4.19]ããã³æè²[13.36]ã§åºã䜿çšãããŠããŸãã ãã«ããã§ãŒãºãã¥ãŒã€ã³ã°ã·ã¹ãã ã¯ã䞊åã³ã³ãã¥ãŒãã£ã³ã°ã®äœ¿çšãšåæ§ã«ãåŠçã®å®éšã«é©ãããã©ãããã©ãŒã ã§ãã ãŸããç ç©¶ãšç ç©¶ã«ã¯å€ãã®è峿·±ãçè«ççµæããããŸã[12]ã
1.6ã ç§åŠæ å ±åŠæè²ã«ãããPython
Pythonã¯ãç§åŠè ãæè²è ã«ãšã£ãŠæã人æ°ã®ããããã°ã©ãã³ã°èšèªã®1ã€ã§ã[21â23]ã Pythonã¯ç£æ¥ç§åŠèšç®ã§åºã䜿çšãããŠããŸã[14]ã Langtangenã¯ããªã¹ã倧åŠã§ç§åŠæ å ±åŠãæããããã®äž»èŠèšèªãšããŠPythonã䜿çšãã圌ã®é·æçãªçµéšã«ã€ããŠå ±åããŠããŸã[24]ã Pythonã¯ããã°ã©ãã³ã°ãç ç©¶ããæåã®èšèª[38]ãšããŠããŸãèšç®ææ³ã®è©³çްãªç ç©¶[3,20,34]ãšããŠé²æ©ããŠããŸãã
2.åºæ¬
ã¢ããªã³ã°ãéå§ããåã«ãããã»ã¹ã§äœ¿çšããäž»èŠãªã¢ãããŒããå®çŸ©ããŸãã ãã®ç« ã§ã¯ãä¹±æ°çæãšç¢ºçååžã確ççã¢ããªã³ã°ã®åé¡ã«åãçµã¿ãŸãã åç確çè«ãèããŠã¿ãŸãããã ãããã®å®éšã®äž»ãªç®çã¯ãäžå¿æ¥µéå®çã®å®éšç蚌æã§ãã ã¢ãã«ãšãããã®ã¢ãã«ã䜿çšããå®éšã«ãããæ¬äŒŒä¹±æ°ãžã§ãã¬ãŒã¿ãŒãšæºä¹±æ°ãžã§ãã¬ãŒã¿ãŒã®åçãæç¢ºã«ãªããææ°ååžãçè§£ãããŸãã ããã¯ãQSã¢ãã«ã䜿çšãããã詳现ãªå®éšã®åºç€ãæäŸããŸãã
2.1ã ã©ã³ãã 倿°ãšååž
確çè«ã®ãã¹ãŠã®èŠçŽ ã¯äŒçµ±çã«çè§£ããã®ãé£ãããšèããããŠãããåžžã«åœéæè²æ©é¢ã®å©çã®ç¯å²å ã«ãããŸã[15]ã åæã«ããããã®åé¡ã¯ç§åŠç ç©¶ã«ãããŠéèŠãªåœ¹å²ãå ããŠããŸã[10]ã ã¢ããªã³ã°ããŒã¹ã®ã¢ãããŒãã«ããããã®è³æã®çè§£ã容æã«ãªããŸãã ãã®èšäºã§åãäžããã¢ãã«ã¯ã1ã€ããè€æ°ã®ãµã€ã³ããæããããã®åçŽãªã¢ãã«ã§ãã
ãããã®å°å ¥å®éšã®ã¿ã¹ã¯ã¯éåžžã«è€éã§ãã 確çååžã ãã§ãªããã¢ããªã³ã°ãšäžŠåèšç®ãåãäžããŸãã ãŸããç§åŠç ç©¶ã®äžæ©ãèžã¿åºããŸããäžå€®æ¥µéå®çãå®éšçã«èšŒæããŸãã
ïŒååžã«åœ±é¿ãäžããããšãªãïŒä¹±æ°ãçæããããšããå§ããŸãã æ¬¡ã«ãäžæ§ååžã®ã©ã³ãã 倿°ã«ã€ããŠèª¬æããŸãã çã®ã©ã³ãã æ§ãšæºã©ã³ãã æ§ã«é¢ããè°è«ã¯ãèè ã«ãã£ãŠæç€ºãããŠããŸã[26ã35]ã äžçŽè åãã«ãPythonç䌌ã©ã³ãã 倿°ãžã§ãã¬ãŒã¿ãŒã䜿çšããäžé£ã®å®éšã玹ä»ãããŸãã åææ®µéã§ã¯ãç ç©¶ãæç¢ºã«ããããã«ãã·ãã¥ã¬ãŒã·ã§ã³çµæã芳å¯ããªãããã¹ãã®æ°ãå¢ãããŸãã æ¬¡ã®æ®µéã§ã¯ãããè€éãªå®éšãšäžŠåèšç®ã«é²ã¿ãŸãã ã¢ããªã³ã°ã«ã¯Pythonã®ã©ã³ãã 倿°ã¢ãžã¥ãŒã«ã䜿çšãã䞊åèšç®ã«ã¯mpi4pyã©ã€ãã©ãªã䜿çšããŸãã Pythonã©ã³ãã 倿°ã¢ãžã¥ãŒã«ã¯ãããŸããŸãªååžã®æ¬äŒŒä¹±æ°ãžã§ãã¬ãŒã¿ãŒã«åºã¥ããŠããŸãã äŸïŒ random.randintïŒaãbïŒã¯ãã©ã³ãã ãªæŽæ°Nãè¿ããŸããããã§ã aâ€Nâ€bããã³random.expovariateïŒlambdïŒã¯ããã©ã¡ãŒã¿ãŒ 'lambd'ã䜿çšããŠææ°ååžããã©ã³ãã 倿°ãè¿ããŸãã 詳现ã«ã€ããŠã¯ãPythonã®ããã¥ã¡ã³ããåç §ããŠãã ããã ç«æ¹äœæãã¢ãã«ã®ããã°ã©ãã³ã°ãå³2ã«ç€ºããŸãã
import pylab import random number_of_trials =100 ## Here we simulate the repeated throwing of a single six-sided die list_of_values = [] for i in range(number_of_trials): list_of_values.append(random.randint( 1,6)) print "Trials =", number_of_trials, "times." print "Mean =", pylab.mean(list_of_values) print "Standard deviation =", pylab.std(list_of_values) pylab.hist(list_of_values, bins=[0.5,1.5,2.5,3-5,4.5,5.5,6.5]) pylab.xlabel('Value') pylab.ylabel('Number of times') pylab.show()
å³ 2. Pythonã§åäžã®ãã€ã¹ãæããã¢ãã«ã®ããã°ã©ãã³ã°
1ã€ã®ãã€ããã¹ããã·ãã¥ã¬ãŒã·ã§ã³ã®çµæãå³3ã«ç€ºããŸãã

å³ 3. 1ã€ã®ãã€ã¹ã®ãã¹ãã¢ããªã³ã°ããçµæ
次ã«ã2ã€ã®ãµã€ã³ããæããå ŽåãèããŸãã ãã®æ®µéã§ã®äž»ãªã¢ã€ãã¢ã¯ãç°ãªãæ°ã®ãã¥ãŒãã䜿çšããå®éšã䜿çšããäžå€®åŠçã»ã³ã¿ãŒã®èª¬æã§ãã å³4ã¯ãã®èãæ¹ã瀺ããŠããŸãã

å³ 4.確çå¯åºŠé¢æ°ã®æ¯èŒ
調æ»ããã»ã¹ã¯ãã³ãŒããä¿®æ£ããŠãè€æ°ã®ãµã€ã³ãã®ã±ãŒã¹ãæ€èšãå§ãããããªæ¹æ³ã§2ã€ã®ãµã€ã³ããæããããšãã·ãã¥ã¬ãŒãããããšã«ãã£ãŠç¶è¡ãããŸãã ã³ãŒãã¯ã以äžã«ç€ºã2è¡ã®ã³ãŒããé€ããŠã1ã€ã®ãã¥ãŒããæã€ã³ãŒãã«äŒŒãŠããŸãã
... list_of_values.append(random.randint(1, 6) + random.randint(1, 6)) ... pylab.hist(list_of_values, pylab.arange(1.5, 13.5, 1.0)) ...
2ã€ã®ãã¥ãŒãã®å Žåã®èšç®çµæãå³5ã«ç€ºããŸãã

å³ 5. 2ã€ã®ãã¥ãŒãã®å Žå
ããã§ãæ£èŠååžãæ€èšã§ããŸãã ãã®æ®µéã§ã®ã¿ã¹ã¯ã¯ãããã€ãã®ãã¥ãŒããæã€åã®ã±ãŒã¹ãã©ã®ããã«æ£èŠååžãšçžé¢ãããã瀺ãããšã§ãã æ¬¡ã®ã¿ã¹ã¯ã§ã¯ãå¹³åãšæšæºåå·®ã玹ä»ããŸãã ã³ãŒãã¯ã以äžã®æé ãé€ããåäžã®ãã¥ãŒãã®å ŽåãšåããŸãŸã§ãã
... list_of_values.append(random.normalvariate(7, 2.4)) ...
æ£èŠååžã®ã·ãã¥ã¬ãŒã·ã§ã³çµæãå³6ã«ç€ºããŸãã
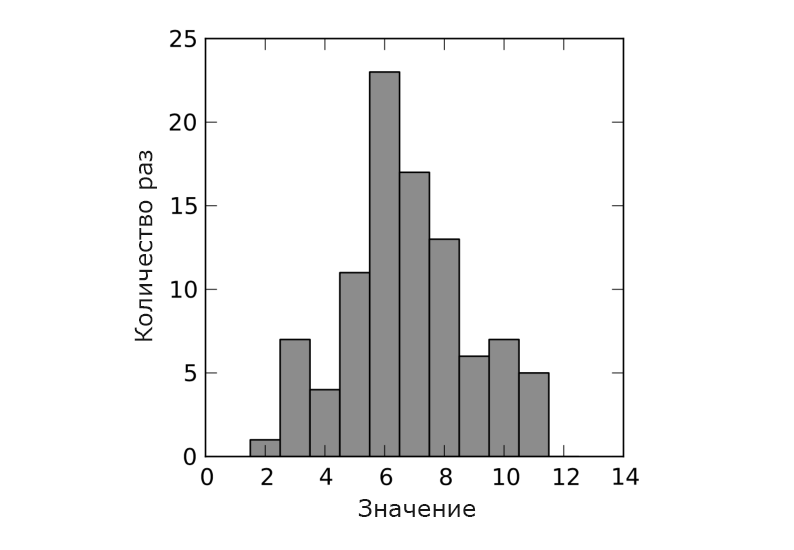
å³ 6.æ£èŠååžã®ã·ãã¥ã¬ãŒã·ã§ã³çµæ
æåŸã®ã¹ãããã¯ãææ°ååžã瀺ãããšã§ãã ææ°ååžã¯ãããŸããŸãªã¿ã€ãã®ã·ã¹ãã ã§èŠä»¶ãåä¿¡ããç¬éã®ééã®ååžïŒæéïŒãã¢ãã«åããããã«äœ¿çšãããŸãã ãããã®ã¢ããªã³ã°ã®çµæã¯ãå³7ããã³8ã«ç€ºãããŠããŸãã
import pylab import random number_of_trials = 1000 number_of_customer_per_hour = 10 ## Here we simulate the interarrival time of the customers list_of_values = [] for i in range(number of trials): list_of_values.append(random.expovariate(number_of_customer_per_hour)) mean=pylab.mean(list_of_values) std=pylab.std(list_of_values) print "Trials =", number_of_trials, "times" print "Mean =", mean print "Standard deviation =", std pylab.hist(list_of_values,20) pylab.xlabel('Value') pylab.ylabel('Number of times') pylab.show()
å³ 7.ææ°ååžã®Pythonã¢ãã«
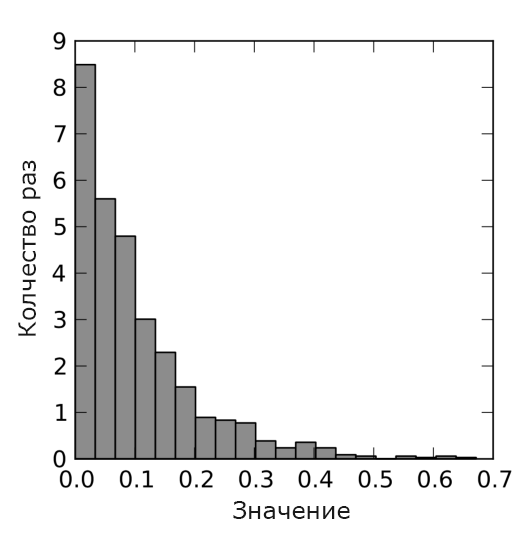
å³ 8.ææ°ååžã®ã·ãã¥ã¬ãŒã·ã§ã³çµæ
2.2ã 確ççã¢ããªã³ã°
確ççã¢ããªã³ã°ã¯ç§åŠæ å ±åŠã®éèŠãªèŠçŽ ã§ãã ã¢ã³ãã«ã«ãæ³ã«çŠç¹ãåœãŠãŸã[10,11,27]ã ã¢ãã«ãæ§ç¯ãããåŸãã©ã³ãã 倿°ãçæããã·ã¹ãã ã®ããŸããŸãªãã©ã¡ãŒã¿ãŒãå®éšã§ããŸãã ãã®èšäºã§ã¯ãã¢ã³ãã«ã«ãå®éšã®éèŠãªãã€ã³ãã¯ãçµæãèç©ããŠçµ±åããããã«ãã¹ããäœåºŠãç¹°ãè¿ãããšã§ãã æãç°¡åãªã¢ããªã±ãŒã·ã§ã³ã«ã€ããŠã¯ãåã®ã»ã¯ã·ã§ã³ã§èª¬æããŸããã ãã¹ãã®æ°ãå¢ããããšã«ãããã·ãã¥ã¬ãŒã·ã§ã³çµæã®ç²ŸåºŠãåäžããŸãã
ããã§ãåŠçã¯ãã¹ãã®æ°ãå¢ããããšã«ããããã®åçŽãªã¢ãã«ã䜿çšããŠç¹å®ã®æ°ã®å®éšãè¡ãå¿ èŠããããŸãã ãã¥ãŒãã®æ°ãšãã¹ãã®æ°ãå¢ãããããçåŸã¯æ¯èŒçé·ãèšç®æéã«çŽé¢ããŸãã ããã¯ã䞊åã³ã³ãã¥ãŒãã£ã³ã°ã䜿çšãã倧ããªçç±ã§ãã ããã€ãã®ãµã€ã³ãã®Pythonã¢ãã«ãå³9ã«ç€ºããŸããã·ãã¥ã¬ãŒã·ã§ã³çµæãå³10ã«ç€ºããŸããæ¬¡ã®ã¹ãããã§ã¯ãããŸããŸãªãã¥ãŒã·ã¹ãã ã«é¢é£ããããäžè¬çãªåé¡ãæ€èšããŸãã QSåé¡ã®æŠèŠã«ã€ããŠã¯ããã®èšäºã®æ¬¡ã®ããŒãã§èª¬æããŸãã M / M / 1ã·ã¹ãã ãšããè€éãªãã¥ãŒã€ã³ã°ã·ã¹ãã ã§èª¿æ»ãéå§ããŸãã 確ççããã»ã¹ã®åºæ¬æŠå¿µã«ã€ããŠã¯ããã®èšäºã®ãã®éšåã§è©³ãã説æããŸãã å¯èœãªäŸãšããŠãåºåã¹ããªãŒã ã調ã¹ãåé¡ãææ¡ã§ããŸãã M / M / 1ã·ã¹ãã ã®å°åºããã¢ãœã³ãããŒã§ããããšã蚌æããŸãããã ãããã£ãŠãåéãããããŒã¿ã¯ãæ§ç¯ãããçµéšçãã¹ãã°ã©ã ã®åœ¢åŒã§è¡šç€ºãããŸãã
import pylab import random number_of_trials = 150000 number_of_dice = 200 ## Here we simulate the repeated throwing ## of a number of single six-sided dice list_of_values = [] for i in range(number_of_trials): sum=0 for j in range(number_of_dice): sum+=random.randint(1,6) list_of_values.append(sum) mean=pylab.mean(list_of_values) std=pylab.std(list_of_values) print "Trials =", number_of_trials, "times" print "Mean =", mean print "Standard deviation =", std pylab.hist(list_of_values,20) pylab.xlabel('Value') pylab.ylabel('Number of times') pylab.show()
å³ 9.æ¡åŒµæ£èŠååžã®Pythonã¢ããªã³ã°ã¢ãã«

å³ 10.æ¡åŒµæ£èŠååžã®ã·ãã¥ã¬ãŒã·ã§ã³çµæ
3.å€çžåŸ ã¡è¡åã·ã¹ãã ãšç¢ºççã¢ããªã³ã°
以äžã¯ãã¢ããªã³ã°ãšç¢ºçè«ã®ãã¥ã¢ã³ã¹ãèæ ®ã«å ¥ãããQSã®å ¥é説æã§ãã
3.1ã ãã«ã¯ãµãŒãã¹ã·ã¹ãã
åçŽãªãã¥ãŒã€ã³ã°ã·ã¹ãã ã¯ãçä¿¡èŠæ±ãåŠçãã1ã€ã®ãµãŒãã³ã°ããã€ã¹ã§æ§æãããŸãã åçŽãªãã¥ãŒã€ã³ã°ã·ã¹ãã ã®äžè¬çãªã¹ããŒã ãå³11ã«ç€ºããŸããäžè¬ã«ãQSã¯çä¿¡èŠæ±ãåŠçãã1ã€ä»¥äžã®ãµãŒãã¹æäŸããã€ã¹ã§æ§æãããŸãã åãã§ãŒãºã§1ã€ä»¥äžã®ãµãŒãã¹ããã€ã¹ã䜿çšãã1ã€ä»¥äžã®ãµãŒãã¹ã¹ããŒãžãå¯èœã§ãã ãã¹ãŠã®ãµãŒããŒãããžãŒã§ãããšãããçä¿¡ã¯ã©ã€ã¢ã³ãã¯ããµãŒãã¹ãæäŸããããã€ã¹ã®åã«ãã1ã€ä»¥äžã®ãã¥ãŒã«åå ããŠããŸãã å®åã·ã¹ãã ãéä¿¡ã·ã¹ãã ãä¿å®ã·ã¹ãã ãªã©ãQSãã·ãã¥ã¬ãŒãã§ããå€ãã®ã¢ããªã±ãŒã·ã§ã³ããããŸãã äžè¬çãªQSã¯ãã¢ããªã±ãŒã·ã§ã³ã®ãããŒããµãŒãã¹ããã»ã¹ããã¥ãŒãµãŒãã¹ã¡ãœããã®3ã€ã®äž»èŠãªã³ã³ããŒãã³ãã§ç¹åŸŽä»ããããŸãã ã¢ããªã±ãŒã·ã§ã³ã¯ãããã€ãã®éå®ããããœãŒã¹ãŸãã¯ç¡å¶éã®ãœãŒã¹ããæäŸãããŸãã

å³ 11.ã·ã³ãã«QSã
ã¢ããªã±ãŒã·ã§ã³ããã»ã¹ã§ã¯ãã¢ããªã±ãŒã·ã§ã³ãã·ã¹ãã ã«å ¥ãæ¹æ³ã説æããŸãã å®çŸ©ãã
alphai ã¢ããªã±ãŒã·ã§ã³ã®å°çéã®æéééãšã㊠ïŒiâ1ïŒ ãã㊠i ã¢ããªã±ãŒã·ã§ã³ãã¢ããªã±ãŒã·ã§ã³ã®åä¿¡éã®äºæ³ïŒå¹³åïŒæé EïŒ\ã¢ã«ãã¡ïŒ ããã³ã¢ããªã±ãŒã·ã§ã³ã®åä¿¡é »åºŠ lambda= frac1EïŒ alphaïŒ
ç§ãã¡ãå®çŸ©ããŸã s æäŸããã€ã¹ã®æ°ãšããŠã ãµãŒãã¹ã¡ã«ããºã ã¯ãã®çªå·ã«ãã£ãŠæ±ºå®ãããŸãã åãµãŒãã³ã°ããã€ã¹ã«ã¯ç¬èªã®ãã¥ãŒããããã¢ããªã±ãŒã·ã§ã³ã®ãµãŒãã¹æéã®ç¢ºççååžããããŸãã
å®çŸ©ãã si ãµãŒãã¹ã¯äœæã§ãã i çªç®ã®ã¢ããªã±ãŒã·ã§ã³ EïŒsïŒ ã¢ããªã±ãŒã·ã§ã³ã®å¹³åãµãŒãã¹æéãšã㊠mu= frac1EïŒsïŒ ã¢ããªã±ãŒã·ã§ã³ã®ãµãŒãã¹é床ãšããŠã
ãµãŒãã¹ããã€ã¹ããã¥ãŒããæ¬¡ã®ãã±ãããéžæããããã«äœ¿çšããã«ãŒã«ã¯ãQSãã¥ãŒãã£ã·ããªã³ãšåŒã°ããŸãã æãäžè¬çãªãã¥ãŒã®åéã¯æ¬¡ã®ãšããã§ããåªå 床â顧客ã¯éèŠåºŠã®é«ãé ã«ãµãŒãã¹ãããŸãã FIFO-å çé ; LIFOãã¹ã¿ãã¯ã§ãæåŸã®ãã®ãæåã«æäŸãããŸããã æ¡åŒµKendallã·ã¹ãã åé¡ã¯6æåã䜿çšããŸãïŒA / B / s / q / c / pãããã§Aã¯çä¿¡èŠæ±éã®ééã®ååžãBã¯ãµãŒãã¹ééã®ååžãsã¯ãµãŒããŒæ°ãqã¯ãµãŒãã¹èŠåŸïŒFIFOã§ã¯çç¥ïŒ ã-ã·ã¹ãã 容éïŒç¡éãã¥ãŒã§ã¯çç¥ïŒãp-å¯èœãªãªã¯ãšã¹ãã®æ°ïŒãªãŒãã³ã·ã¹ãã ã§ã¯çç¥ïŒ[17.37]ã ããšãã°ãM / M / 1ã¯ããã¢ãœã³å ¥åã¹ããªãŒã ã1ã€ã®ææ°ãµãŒãã³ã°ããã€ã¹ã1ã€ã®ç¡éFIFOãã¥ãŒãããã³ç¡éæ°ã®ã¢ããªã±ãŒã·ã§ã³ã衚ããŸãã
QSã¯ãç§åŠæè¡ã®ããŸããŸãªåéã®ã¢ããªã³ã°ãšç ç©¶ã«äœ¿çšãããŸãã ããšãã°ããã¥ââãŒã€ã³ã°çè«ã䜿çšããŠãçç£ã·ã¹ãã ãŸãã¯èŒžéã·ã¹ãã ãã¢ããªã³ã°ããã³ç ç©¶ã§ããŸãã ããã«ããµãŒãã¹èŠæ±ã¯ã¢ããªã±ãŒã·ã§ã³ãšèŠãªãããä¿å®æé ã¯ãµãŒãã¹ã¡ã«ããºã ãšèŠãªãããŸãã æ¬¡ã®äŸã¯ããã§ãïŒã³ã³ãã¥ãŒã¿ãŒïŒãããã端æ«èŠæ±ãšãµãŒããŒå¿çïŒãã³ã³ãã¥ãŒã¿ãŒãã«ããã£ã¹ã¯ã¡ã¢ãªã·ã¹ãã ïŒããŒã¿ã®æžã蟌ã¿/èªã¿åãèŠæ±ãå ±æãã£ã¹ã¯ã³ã³ãããŒã©ãŒïŒããã©ã³ã¯ç¡ç·éä¿¡ïŒé»è©±ä¿¡å·ããªããŒã¿ãŒïŒãã³ã³ãã¥ãŒã¿ãŒãããã¯ãŒã¯ïŒèŠæ±ããã£ãã«ïŒ[39 ]ã çç©åŠã§ã¯ããã¥ãŒã€ã³ã°çè«ã䜿çšããŠãé µçŽ ã·ã¹ãã ïŒã¿ã³ãã¯è³ªãäžè¬é µçŽ ïŒãã¢ãã«åã§ããŸãã çååŠã§ã¯ããã¥ãŒã€ã³ã°ãããã¯ãŒã¯ã¢ãã«ã䜿çšããŠãLACãªããã³ã®èª¿ç¯åè·¯ãç ç©¶ã§ããŸãã
3.2ã ãªãå€çžãªã®ãïŒ
çŽåã«æ¥ç¶ãããç¡å¶éã®æ°ã®ã¢ããªã±ãŒã·ã§ã³ãæã€è€æ°ã®ãµãŒãã¹ããã€ã¹ã§æ§æããããã«ããã§ãŒãºQSãæ€èšããŸãã ãªã¯ãšã¹ãéã®æéãšåŠçæéã¯ç¬ç«ããŠãããææ°é¢æ°çã«åæ£ããŠããŸãã ã©ã€ã³ãããã¯ãFIFOãµãŒãã¹ã®åéã§ã¯ç¡éã§ãã ãã«ããã§ãŒãºQSã¯ãåœç¶ããã«ãã³ã¢ã³ã³ãã¥ãŒã¿ãŒã·ã¹ãã ã®ããããžãåæ ããŠããŸãã åŸã§èŠãããã«ãåã¢ãã«ã¯ããã°ã©ãã³ã°èšèªã§ç°¡åã«èšè¿°ãã調æ»ããã³ä¿®æ£ã§ããŸãã ãã®ã¢ãã«ã¯ãããŸããŸãªãã«ãããã»ãã·ã³ã°ã¢ãããŒãã®æ¯èŒç ç©¶ãå¯èœã«ããŸãã ãã«ããã§ãŒãºQSã¢ãã«ãå³12ã«ç€ºããŸãã

å³ 12.ãã«ããã§ãŒãºQSã
3.3ã çè«çæ ¹æ
çµ±èšã¢ããªã³ã°ã®å Žåãã³ã³ãã¥ãŒã¿ãŒã³ãŒããæ€èšŒãããšããåé¡ã«åžžã«çŽé¢ããŠããŸãã ããã°ã©ã ãŸãã¯ã¢ã«ãŽãªãºã ã®ãšã©ãŒã®åé¡ã¯åžžã«æªè§£æ±ºã®ãŸãŸã§ãã ã¢ãã«ã¯å®å šã«åæçã§ã¯ãªããããã°ã©ã ãå®è¡ãããã³ã«ãå ¥å/åºåã«ç°ãªãããŒã¿ããããŸãã ãããã£ãŠãã³ãŒããŸãã¯ã¢ã«ãŽãªãºã ã®æ£ç¢ºæ§ã確èªããã«ã¯ãããŸããŸãªã¢ãããŒããå¿ èŠã§ãïŒå®å šã«ç¢ºå®çãªå ¥åããŒã¿ã®å Žåã«äœ¿çšããã¢ãããŒãããïŒã ãã®åé¡ã解決ããã«ã¯ãç§åŠæç®ã«èšèŒãããŠããããã€ãã®ç ç©¶ã®çè«ççµæãé©çšããå¿ èŠããããŸãã ãããã®çµæã¯ãåºåããŒã¿ã®æ€èšŒãšåæãããã³ã·ãã¥ã¬ãŒã·ã§ã³çµæã®æ£ç¢ºãã®åé¡ã解決ããããã®åºç€ãšãªããŸã[31,32]ã
ãã«ããã§ãŒãºQSã§ã®ã¢ããªã±ãŒã·ã§ã³ã®æ»çæéã調æ»ããŸãã 瀺ã Tjãn ã·ã¹ãã å ã®ã¢ããªã±ãŒã·ã§ã³ãè²»ãããæéãšããŠã SïŒjïŒn jçªç®ã®ãã§ãŒãºã®nçªç®ã®ã¢ããªã±ãŒã·ã§ã³ã®ãµãŒãã¹æéãšããŠã æ€èšãã alphak ã©ããã£ãŠ EïŒ\ã¢ã«ãã¡ïŒ kçªç®ã®ãã§ãŒãºã
ãã®ãããªå®æ°ããããŸã gamma>0 ãã®ãããª
$supn geq2E|SïŒjïŒn|4+ gamma< inftyãj=0ã1ã2ã...ãkïŒ1ïŒ alphak>akâ1>...> alpha1>0ïŒ2ïŒ
å®ç æ¡ä»¶ïŒ1ïŒããã³ïŒ2ïŒãæºããããå Žåã
P biggïŒ overline limn to infty fracTjãnâ alphaj cdotn widetilde sigma cdot alphaïŒnïŒ=1 biggïŒ=P biggïŒ\äžç· limn to infty fracTjãnâ alphaj cdotn widetilde sigma cdot alphaïŒnïŒ=â1 biggïŒ=1ãj=1ã2ã...ã3ãk; alphaïŒnïŒ= sqrtïŒ2n ln lnnïŒ
3.4ã çµ±èšã¢ããªã³ã°
ã¢ãã«ãæ§ç¯ãããåŸããã®ã¢ãã«ã§äžé£ã®å®éšãè¡ãããšãã§ããŸãã ããã«ãããã·ã¹ãã ã®ç¹æ§ã®äžéšã調ã¹ãããšãã§ããŸãã äºæ³ãããå¹³åå€ã§ã©ã³ãã ãªå€ãçæãã調æ»ã«å¿ èŠãªå€ãèšç®ããŸãïŒä»¥äžã«ç€ºãååž°æ¹çšåŒã䜿çšïŒã ãããã®å€ãã©ã³ãã ã«ãªããŸãïŒã¢ãã«ã®å ¥åããŒã¿ã®ç¢ºçè«-ã¢ããªã±ãŒã·ã§ã³ã®å°çãšã©ã³ãã ãªãµãŒãã¹æéã®éã®ã©ã³ãã ãªæéïŒã ãã®çµæããããã®ã©ã³ãã 倿°ïŒå€æ°ïŒã®ãã©ã¡ãŒã¿ãŒïŒå¹³åå€ãšç¢ºçååžïŒãèšç®ã§ããŸãã ãã®æ¹æ³ã¯ãã¢ãã«ã«ã©ã³ãã æ§ãååšãããããçµ±èšã¢ããªã³ã°ãšåŒã°ããŸãã ããæ£ç¢ºãªçµæãå¿ èŠãªå Žåã¯ãã¢ãã«ã§å®éšãç¹°ãè¿ããŠããçµæãçµ±åããç©åç¹æ§ïŒå¹³åå€ãŸãã¯æšæºåå·®ïŒãèšç®ããå¿ èŠããããŸãã ããã¯ã¢ã³ãã«ã«ãæ³ãšåŒã°ããèšäºã§ããå°ã詳ãã説æãããŠããŸãã
3.5ã ååž°æ¹çšåŒ
åè¿°ã®QSã®ã¢ããªã³ã°ã¢ã«ãŽãªãºã ãéçºããã«ã¯ãããã€ãã®æ°åŠçãªæ§é ãåæããå¿ èŠããããŸãã äž»ãªã¿ã¹ã¯ã¯ããã«ããã§ãŒãºQSã§ã®çªå·nã®ã¢ããªã±ãŒã·ã§ã³ã®æ»çæéã調ã¹ãŠèšç®ããããšã§ãã k ãã§ãŒãºã 次ã®ååž°æ¹çšåŒ[12]ãäžããããšãã§ããŸãã tn -å°çæé n çªç®ã®ã¢ããªã±ãŒã·ã§ã³ã SïŒjïŒn ãµãŒãã¹æéã¯ã©ãã§ãã n ç³èŸŒã¿ j 段é alphan=tnâtnâ1;j=1ã2ã...ãk;n=1ã2ã...ãN. ã æ¬¡ã®ç¹°ãè¿ãæ¹çšåŒã¯ãåŸ ã¡æéã«ã€ããŠæãç«ã¡ãŸã Tjãn ã®ããã« n ç³èŸŒã¿ j ãã§ãŒãºïŒ
Tjãn=Tjâ1ãn+Sjn+ maxïŒTjãnâ1âTjâ1ãnâ alphanã0ïŒ;j=1ã2ã...ãk;n=1ã2ã...ãN;Tjã0=0ã forallj;T0ãn=0ã foralln;
æšæž¬ãã ãã«ããã§ãŒãºQSã§ã®ã¢ããªã±ãŒã·ã§ã³ã®æ»çæéãèšç®ããããã®ç¹°ãè¿ãæ¹çšåŒã
蚌æã æéãããã° alphan+Tjâ1ãnâ¥Tjãnâ1 ããã®åŸã®åŸ ã¡æé j 段é n thã¢ããªã±ãŒã·ã§ã³ã¯0ã§ãããã®å Žå alphan+Tjâ1ãn<Tjãnâ1 ã§ã®åŸ ã¡æé j 段é n ç³èŸŒã¿ omeganj=Tjãnâ1âTjâ1ãnâ alphan ãã㊠Tjãn=Tjâ1ãnâ omeganj+SïŒjïŒn ã äžèšã®2ã€ã®ã±ãŒã¹ãèãããšãäºæ³ãããçµæã«ãªããŸãã
ããã§ãåŸããããã¹ãŠã®çè«çµæã«åºã¥ããŠå¿ èŠãªã¢ã«ãŽãªãºã ã®å®è£ ãéå§ã§ããŸãã
4.ãã«ãããã»ãã·ã³ã°çšã®Python
ããã°ã©ãã³ã°èšèªãšããŠã®Pythonã¯ãç§åŠè ãæè²è ã®éã§éåžžã«äººæ°ããããç§åŠæåã®åé¡ã解決ããã®ã«éåžžã«é åçã§ã[3]ã Pythonã¯ãã°ã©ãã£ã«ã«ãŠãŒãã£ãªãã£ãå¹ åºãæ°åŠçããã³çµ±èšããã±ãŒãžãããã³ãã«ãããã»ãã·ã³ã°çšããã±ãŒãžãå«ããã¢ããªã³ã°ãšã·ãã¥ã¬ãŒã·ã§ã³ã®ããã®åŒ·åãªãã©ãããã©ãŒã ãæäŸããŸãã å®è¡æéãççž®ããã«ã¯ãPythonã³ãŒããšCã³ãŒããçµã¿åãããå¿ èŠããããŸãã ããããã¹ãŠããçµ±èšããŒã¿ãšåŠççµæãååŸããããã®åŒ·åãªã¢ããªã³ã°ãã©ãããã©ãŒã ãæäŸããŸãã ã¢ããªã³ã°ã§ãéèŠãªPythonã®éèŠãªæŠå¿µã¯ããã³ã¬ãŒã¿ãã³ã«ãŒãã³ãyieldåŒããã«ãããã»ãã·ã³ã°ãããã³ãã¥ãŒã§ãã ããŒãºãªãŒã¯æ¬ã®äžã§ãããã®ç¹ãéåžžã«ãã調ã¹ãŠãã[2]ã ããã«ãé¢ããããããã»ã¹ééä¿¡ãæŽçããæ¹æ³ã¯ããã€ãããããã¥ãŒã®äœ¿çšããå§ããŸããããã¯ãQSã®ç ç©¶ã®èгç¹ããéåžžã«èªç¶ãªããšã§ãã
以äžã¯ããã«ãããã»ãã·ã³ã°ã䜿çšããŠããã°ã©ã ã³ãŒãã®å¹çãšæå¹æ§ãé«ããå©ç¹ã®ç°¡åãªäŸã§ãã åŠçã¯ãã¹ãŒããŒã³ã³ãã¥ãŒã¿ãŒãŸãã¯ã¯ã©ã¹ã¿ãŒã·ã¹ãã ã§äžŠåèšç®ã䜿çšããããšã«ãããã·ãã¥ã¬ãŒã·ã§ã³çµæãæ¹åã§ããŸã[28ã29]ã äžæ¹ã§ããã«ãããã»ãã·ã³ã°ã䜿çšãããšããã«ããã§ãŒãºã¢ãã«ããã«ãã³ã¢ããã»ããµã®ãªãœãŒã¹ãšæ¯èŒã§ããŸãã仿¹ã§ã¯ããã«ãããã»ãã·ã³ã°ã䜿çšããŠäžé£ã®äžŠåã¢ã³ãã«ã«ããã¹ããå®è¡ã§ããŸãã æ¬¡ã®ã»ã¯ã·ã§ã³ã§ã¯ãããã2ã€ã®ã¢ãããŒããæ€èšããŸãã ããæ°ã®ããåŠçã®ããã«ãPythonã䜿çšãããã«ãããã»ãã·ã³ã°ã®ç°¡åãªç޹ä»ã以äžã«ç€ºããŸãã
ãŸããmpi4pyã¢ãžã¥ãŒã«ã䜿çšããŸãã ããã¯ãMPIãã©ã®ããã«æ©èœãããã®äž»èŠãªã¢ã€ãã¢ãæç€ºããããã«éèŠã§ãã æäŸãããããã°ã©ã ããŠãŒã¶ãŒå®çŸ©ã®ããã»ããµã³ã¢ã®1ã€ã«ã³ããŒããgatherïŒïŒã¡ãœããã䜿çšããåŸã«çµæãçµ±åããŸãã Pythonã³ãŒãã®äŸïŒå³13ïŒãšã·ãã¥ã¬ãŒã·ã§ã³çµæïŒå³14ïŒã以äžã«ç€ºããŸãã
#!/usr/bin/python import pylab import random import numpy as np from mpi4py import MPI dice=200 trials= 150000 rank = MPI.COMM_WORLD.Get_rank() size = MPI.COMM WORLD.Get_size() name = MPI.Get_processor_name() random.seed(rank) ## Each process - one throwing of a number of six-sided dice values= np.zeros(trials) for i in range(trials): sum=0 for j in range(dice): sum+=random.randint(l,6) values[i]=sum data=np.array(MPI.COMM_WORLD.gather(values, root=0)) if rank == 0: data=data.flatten() mean=pylab.mean(data) std=pylab.std(data) print "Number of trials =", size*trials, "times." print "Mean =", mean print "Standard deviation =", std pylab.hist(data,20) pylab.xlabel('Value') pylab.ylabel('Number of times') pylab.savefig('multi_dice_mpi.png')
å³ 13. MPIã䜿çšããæ¡åŒµæ£èŠååžã®Pythonã¢ãã«ã
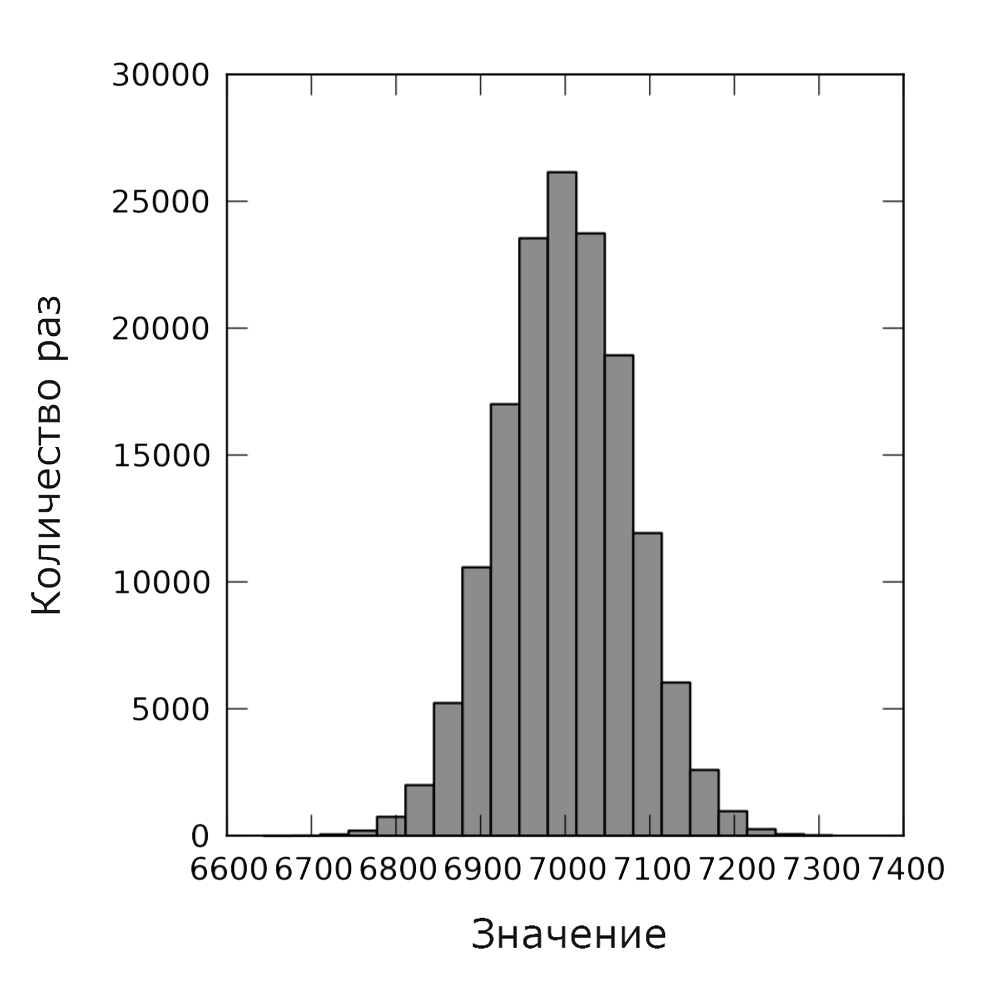
å³ 14. MPIã䜿çšããæ£èŠååžã
5.ã¢ããªã³ã°ããŒã¹ã®æè²ã¢ãããŒã
ãã«ããã§ãŒãºQSã¯ãé©åãªã·ãã¥ã¬ãŒã·ã§ã³ããŒã¹ã®ã¢ãããŒããéçºããããã®ã³ã¢ãæäŸããŸãã ãã®ã¢ãããŒãã«ã¯ãåã®ã»ã¯ã·ã§ã³ã§èª¬æããåºæ¬çãªæŠå¿µãšãããè€éãªçè«çãªçµæã𿹿³ãå«ãŸããŸãã äž»ãªã¢ã€ãã¢ã¯ãæ¬è³ªçã«ç¢ºçè«çã§ãïŒã©ã³ãã 倿°ãã©ã³ãã ãªæ°å€ååžãä¹±æ°ãžã§ãã¬ãŒã¿ãŒãäžå€®æ¥µéå®çã Pythonããã°ã©ãã³ã°æ§æïŒ
ãã³ã¬ãŒã¿ãã³ã«ãŒãã³ãããã³yeildåŒã ããè€éãªçµæã«ã¯ãã·ã¹ãã ã§ã®ã¢ããªã±ãŒã·ã§ã³ã®æ»çæéãQSã§ã®ã¢ããªã±ãŒã·ã§ã³ã®æ»çæéãèšç®ããããã®ååž°æ¹çšåŒã確ççã¢ããªã³ã°ææ³ããã«ãããã»ããµãã¯ãããžãŒãªã©ã®çè«çæŠå¿µãå«ãŸããŸãã å³15ã¯ãåŠæŽã説æããäž»ãªæŠèŠã瀺ããŠããŸãã

å³ 15.ã¢ããªã³ã°ããŒã¹ã®æè²ã¢ãããŒã
ããããã¹ãŠã®çè«çããã³ããã°ã©ã çæ§é ã«ãããåŠçã¯å€çžQSã®ããŸããŸãªã¢ãã«ã§å®éšãè¡ãããšãã§ããŸãã ãã®ãããªå®éšã®ç®çã¯2ã€ãããŸãã 第äžã«ãããã«ãããåŠçã¯æ¬¡ã®ã·ãŒã±ã³ã¹ãçè§£ããããšãã§ããŸããããã¯ãããããç§åŠç ç©¶ã§éèŠã§ãïŒçè«çäºå®ãæ°åŠã¢ãã«ããœãããŠã§ã¢æ§æãã³ã³ãã¥ãŒã¿ãŒã¢ãã«ã確çã¢ãã«ãããã³åŠç¿ã«å¿ èŠãªã·ãã¥ã¬ãŒã·ã§ã³çµæã®èгå¯ã ããã«ãããåŠçã¯äžè¬çãªç ç©¶ã®å šäœåãåŸãããšãã§ããŸãïŒå³16ïŒã
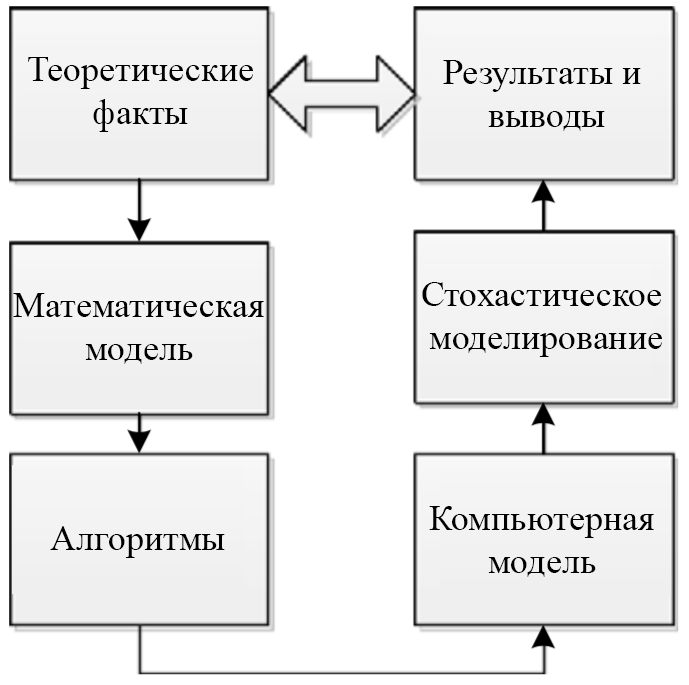
å³ 16.ç ç©¶åé
ãã®ãããªã¢ãããŒãã¯ã確ççã¢ããªã³ã°ãšããã«ãããã»ãã·ã³ã°ã䞊åããã°ã©ãã³ã°ãªã©ã®åºæ¬çãªãœãããŠã§ã¢æ§æã®ããæ·±ãçè§£ãæäŸããŸãã ãããã®èŠå®ã¯ãç§åŠèšç®ã®åéã§æãéèŠã§ãã
5.1ã ã¢ãã«å®éš
ãã®ã»ã¯ã·ã§ã³ã§ã¯ããã«ããã§ãŒãºQSã®3ã€ã®ã³ã³ãã¥ãŒã¿ãŒã¢ãã«ã«ã€ããŠæ€èšããŸãã ãããã®ã¢ãã«ã¯ãã¹ãŠãå²åŠçããã³äž»èŠãªæ©èœãç°ãªããŸãã å®éšã®ç®çã¯çµ±èšã¢ãã«ãäœæããå€çžã·ã¹ãã ã®äž»ãªãã©ã¡ãŒã¿ãŒãç ç©¶ããããšã§ãããšããäºå®ã«ããããããããããã®ã¢ãã«ã®æŠå¿µçãªèãæ¹ã¯å®å šã«ç°ãªã£ãŠããŸãã ãããã®åºæ¬çãªèãæ¹ãæ¯èŒããããšã§ãåŠçã¯äžŠåèšç®ããã«ãããã»ããµçµ±èšãã·ãã¥ã¬ãŒã·ã§ã³ã¢ããªã³ã°ã®åºç€ãšãªãåºæ¬ååãçè§£ããã®ã«åœ¹ç«ã¡ãŸãã
æåã«ç޹ä»ããã¢ãã«ã¯ãªã¢ã«ã¿ã€ã ã®èšé²ã«åºã¥ããŠãããã·ãã¥ã¬ãŒã·ã§ã³ã¢ãã«ãšåŒã°ããŸãã Pythonãã«ãããã»ããµã¢ãžã¥ãŒã«ã䜿çšããŸãããã®ã¢ãã«ã®ç²ŸåºŠã¯ãtimeïŒïŒã¡ãœããã®ç²ŸåºŠãšè§£å床ã«äŸåããŸããããŸããŸãªæ±çšãªãã¬ãŒãã£ã³ã°ã·ã¹ãã ã®å Žåã¯éåžžã«äœãããªã¢ã«ã¿ã€ã ã·ã¹ãã ã®å Žåã¯éåžžã«é«ããªããŸããåŠçã¯ã以åã«ææ¡ãããå埩æ¹çšåŒã䜿çšããŠãã®ã¢ãã«ã倿ŽãïŒã·ã¹ãã ã§ã®ã¢ããªã±ãŒã·ã§ã³ã®æ»çæéãèšç®ããããïŒãäž¡æ¹ã®ã±ãŒã¹ã§çµæãæ¯èŒã§ããŸãã
次ã®ã¢ãã«ã¯ãã·ã¹ãã å ã®ã¢ããªã±ãŒã·ã§ã³ã®æ»çæéãèšç®ãã確ççã¢ããªã³ã°ã«åºã¥ããŠããŸããã¢ãã«ã¯ãã«ãããã»ããµãçŽæ¥äœ¿çšããŸããããã«ãããã»ãã·ã³ã°ã¯ãPythonã®yieldåŒã䜿çšããŠãšãã¥ã¬ãŒããããŸãã
Python MPI mpi4pyã¢ãžã¥ãŒã«ã䜿çšããŠãææ°ã¢ãã«ãããã«è¡šç€ºãããŸããããã§ã¯ãçµ±èšã¢ããªã³ã°ã«å®éã®MPIïŒãã«ãããã»ããµïŒã¢ãããŒãã䜿çšããŸããããã«ãããã¢ã³ãã«ã«ãæ³ã®ãã¹ãæ°ãå¢ããããšãã§ããŸãã
äžè¬ã«ãåŠçã®èª²é¡ã¯ãæäŸãããã¢ãã«ã䜿çšããŠäžé£ã®å®éšãäœæãããã«ããã§ãŒãºQSã§ã®ã¢ããªã±ãŒã·ã§ã³ã®æ»çæéã«å¯Ÿããå埩察æ°ã®æ³åã®å®éšç蚌æ ãååŸããããšã§ãã
5.2ã ãã«ãããã»ããµãµãŒãã¹ã䜿çšããã·ãã¥ã¬ãŒã·ã§ã³ã¢ãã«
以äžã¯ã·ãã¥ã¬ãŒã·ã§ã³ã¢ãã«ã§ããæ€èšãã¹ãäž»ãªåé¡ã¯ãã·ãã¥ã¬ãŒã·ã§ã³ã¢ãã«ãšçµ±èšã¢ãã«ã®éãã§ãããã1ã€ã®éèŠãªåé¡ã¯ãã·ãã¥ã¬ãŒã·ã§ã³ã¢ãã«ã®æ£ç¢ºããšç²ŸåºŠã§ãããŸããæç€ºãããã¢ãã«ã®æ£ç¢ºããšç²ŸåºŠã®åé¡ãéèŠã§ããåŠçã¯ãåŠçã®ééãé »åºŠãã¢ããªã±ãŒã·ã§ã³ã®æ°ããµãŒãã³ã°ããŒãã®æ°ãªã©ã®ããŸããŸãªãã©ã¡ãŒã¿ãŒã«å¿ããŠãã·ãã¥ã¬ãŒã·ã§ã³çµæã調ã¹ãŠæ¯èŒã§ããŸããã¢ãã«ã®äžè¬çãªã¹ããŒã ãå³17ã«ç€ºããŸãã

å³ 17.ã·ãã¥ã¬ãŒã·ã§ã³ã¢ãã«
ããã°ã©ã ã³ãŒãã³ãŒãã¯2ã€ã®äž»èŠéšåã§æ§æãããŠããŸããåè ã¯èšç®ãçŽæ¥ç®çãšããæ¬¡ã¯çµæãæ§ç¯ããããã®ãã®ã§ããèšç®çšã®ã¢ãžã¥ãŒã«ã«ã¯ã3ã€ã®äž»èŠãªæ©èœãå«ãŸããŠããŸããproducerïŒïŒ-ã¢ããªã±ãŒã·ã§ã³ãåãåããããããé 眮ããããã®ãã®ããµãŒããŒïŒïŒ-ã¢ããªã±ãŒã·ã§ã³ã®ãµãŒãã¹çšã consumerïŒïŒ-çµæãååŸããŸãããã®ãœãããŠã§ã¢ã¢ãã«ã¯å®éã®ã·ãã¥ã¬ãŒã·ã§ã³ã«åºã¥ããŠãããèšç®ã«æ°åŒã䜿çšããŸããããã®ç²ŸåºŠã¯ãPythonäžæã¢ãžã¥ãŒã«ã®ç²ŸåºŠã«äŸåããååãšããŠããªãã¬ãŒãã£ã³ã°ã·ã¹ãã ã«äŸåããŸãããµãŒãã³ã°ããã€ã¹ã®åäœã®èšç®ã¯ããã«ãããã»ããµã·ã¹ãã å ã®ããŸããŸãªããã»ã¹éã§åæ£ãããŸããäžèšã®ã¢ãã«ãå®è£ ããããã®ã³ã³ãã¥ãŒã¿ãŒã³ãŒããå³18ã«ç€ºããŸãã
import multiprocessing import time import random import numpy as np def server(input_q,next_q,i): while True: item = input_q.get() if i==0:item.st=time.time() ## start recording time ## (first phase) timc.sleep(random.expovariate(glambda|i])) ##stop recording time (last phase) if i==M-1 :item.st=time.time()-item.st next_q.put(item) input_q.task_done() print("Server%d stop" % i) ##will be never printed why? def producer(sequence,output_q): for item in sequence: time.sleep(random.expovariate(glambda[0])) output_q.put(ilem) def consumer(input_q): "Finalizing procedures" ## start recording processing time ptime=time.time() in_seq=[] while True: item = input_q.get() in_scq+=[item] input_q.task_done() if item.cid == N-1: break print_results(in_seq) print("END") print("Processing time sec. %d" %(time.time()-ptime)) ## stop recording processing time printf("CPU used %d" %(multiprocessing.cpu_count())) def print_resulls(in_seq): "Output rezults" f=open("out.txt","w") f.write("%d\n" % N) for l in range(M): f.write("%d%s" % (glambda[t],",")) f.write("%d\n" % glambda[M]) for t in range(N-1): f.write("%f%s" % (in_seq[t].st,",")) f.write("%f\n" % (in_seq[N-1].st)) f.close() class Client(object): "Class client" def __init__(self,cid,st): self.cid=cid ## customer id self.st=st ## sojourn time of the customer ###GLOBALS N=100 ## total number of customers arrived M=5 ## number of servers ### glambda - arrival + servicing frequency ### = customers/per time unit glambda=np.array([30000]+[i for i in np.linspace(25000,5000,M)]) ###START if __name__ == "__main__": all_clients=[Client(num,0) for num in range(0,N)] q=[multiprocessing.JoinableQueue() for i in range(M+1)] for i in range(M): serv = multiprocessing.Process(target=server,args=(q[i],q[i+1],i)) serv.daemon=True serv.start() cons = multiprocessing.Process(target=consumer,args=(q[M],)) cons.start() ### start 'produsing' customers producer(all_clients,q[0]) for i in q: i.join()
å³ 18.ãã«ãããã»ããµãµãŒãã¹ã䜿çšããã·ãã¥ã¬ãŒã·ã§ã³ã¢ãã«ã®Pythonã³ãŒãã
å匷ãã質åïŒ
- ã°ããŒãã«å€æ°ã¯ããã»ã¹ã«ã©ã®ããã«æäŸãããããã»ã¹éã§å ±æãããŸããïŒ
- ããŸããŸãªãµãŒãã¹ããã€ã¹ã«é¢é£ä»ããããããã»ã¹ãå®äºããæ¹æ³
- ç°ãªãããã»ã¹éã§æ å ±ãããŒã¯ã©ã®ããã«éä¿¡ãããŸããïŒ
- ã¢ãã«ã®æ£ç¢ºãã¯ã©ãã§ããïŒ
- ã¢ãã«ã®æå¹æ§ã¯ã©ãã§ãããç°ãªãããã»ã¹éã§æ å ±ã亀æããã®ã«ã©ããããæéãããããŸããïŒ
ããã§ãmatplotlibã¢ãžã¥ãŒã«ã䜿çšããŠçµæãå°å·ãããã£ãŒãã衚瀺ããåŸãçµæãèŠèŠçã«åæã§ããŸããã¢ãã«ãããã«æ¹åããå¿ èŠãããããšãããããŸãïŒå³19ïŒããããã£ãŠããã匷åãªã¢ãã«ã«é²ãããšãã§ããŸãã
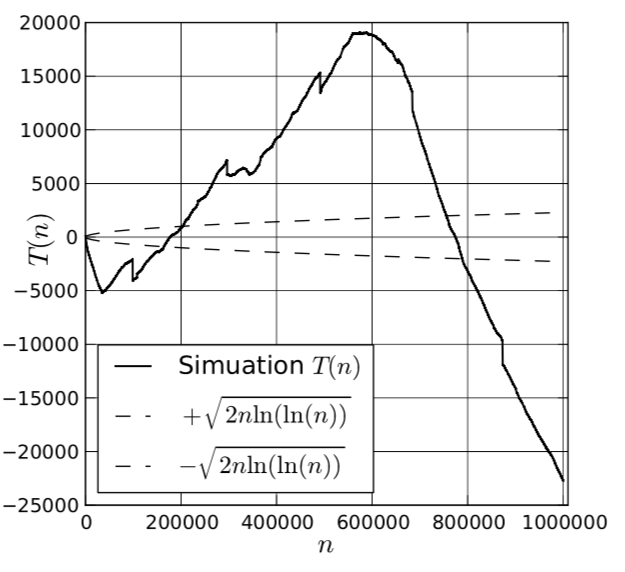
å³ 19.ãã«ãããã»ããµãµãŒãã¹ã®ã·ãã¥ã¬ãŒã·ã§ã³ã¢ãã«ãã¢ãã«åããçµæã
5.3ã çµ±èšã¢ãã«åäœããã»ã¹
çµ±èšã¢ãã«ã®äž»ãªç¹åŸŽã¯æ¬¡ã®ãšããã§ããçŸåšãååž°æ¹çšåŒã䜿çšããŠãã·ã¹ãã å ã®ã¢ããªã±ãŒã·ã§ã³ã®æ»çæéãæ£ç¢ºã«èšç®ããŸããç¹å®ã®Pythonã³ã«ãŒãã³é¢æ°ã䜿çšããŠããã¹ãŠã®ããŒã¿ãåäžã®ããã»ã¹ã§åŠçããŸããèšç®ã®ä¿¡é Œæ§ãæå€§éã«é«ããããã«ãäžå®æ°ã®ã¢ã³ãã«ã«ãã·ãã¥ã¬ãŒã·ã§ã³ãå®è¡ããŸãããã®ã¢ãã«ã«ãããã·ã¹ãã å ã®ã¢ããªã±ãŒã·ã§ã³ã®æ»çæéããæ£ç¢ºã«ãèšç®ã§ããŸããã¢ãã«ã®äž»ãªæŠèŠãå³20ã«ç€ºããŸããåŠçã¯ãã·ãã¥ã¬ãŒã·ã§ã³ã¢ãã«ãšçµ±èšã¢ãã«ã®éãã調ã¹ãããšãã§ããŸãã

å³ 20.çµ±èšã¢ãã«ã®åäžããã»ã¹ïŒ
äžèšã®ã¢ãã«ãå®è£ ããããã®ããã°ã©ã ã³ãŒããå³21ã«ç€ºããŸããã·ãã¥ã¬ãŒã·ã§ã³çµæãå³22ã«ç€ºããŸãã
#!/usr/bin/python import random import time import numpy as np from numpy import linspace def coroutine(func): del start(*args,**kwargs): g = func(*args,**kwargs) g.next() return g return start def print_header(): "Output rezults - header" f=open("out.txt","w") f.write("%d\n" % N) ##number of points in printing template f.write("%d\n" % TMPN) for t in range(M): f.write("%d%s" % (glambda[t],",")) f.write("%d\n" % glambda[M]) f.close() def print_results(in_seq): "Output rezults" f=open("out.txt","a") k=() for i in range(N-2): if in_seq[i].cid==template[k]: f.write("%f%s" % (in_seq[i].st,",")) k+=1 f.write("%f\n" % (in_seq[N-1 ].st)) f.close() coroutine def server(i): ST=0 ##sojourn time for the previous client item=None while True: item = (yield item) ##get item if item == None: ##new Monte Carlo iteration ST=0 continue waiting_time=max(0.0,ST-item.st-item.tau) item.st+=random.expovariate(glambda[i+1])+waiting_time ST=item.st def producer(): results=[] i=0 while True: if i == N: break c=Client(i,0.,0.) if i!=0: c.tau=random.expovariate(glambda[0]) i+= 1 for s in p: c=s.send(c) results+=[c] for s in p: c=s.send(None) ##final signal return results class Client(object): def __init__(self,cid,st,tau): self.cid=cid self.st=st self.tau=tau def params(self): return (self.cid,self.st,self.tau) stt=time.time() N=1000000 ## Clients M=5 ## Servers ## Input/sevice frequency glambda= [30000]+[i for i in linspace(25000,5000,M)] MKS=20 ## Monte Carlo simulation results ## Number of points in the printing template TMPN=N/10000 ##printing template template= map(int,linspace(0,N-1,TMPN)) print_header() p=[] for i in range(M):p +=[server(i)] for i in range! MKS): print_results(producer()) print("Step=%d" % i) sys.stdout.write("Processing time:%d\n" % int(time.time()-stt))
å³ 21.åäžããã»ã¹çµ±èšã¢ãã«ã®Pythonã³ãŒã
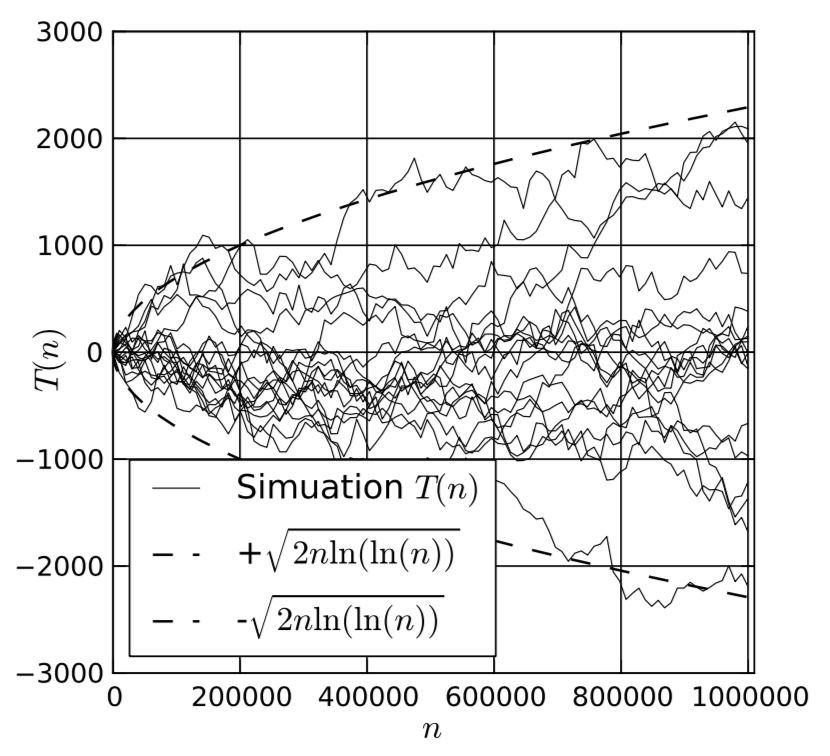
å³ 22.çµ±èšã¢ãã«ã®åäžããã»ã¹ã®ã·ãã¥ã¬ãŒã·ã§ã³çµæ
5.4ã MPIã®çµ±èšã¢ãã«
ã¢ãã«éçºã®æ¬¡ã®ã¹ãããã¯ãPython MPIã¢ãžã¥ãŒã«-mpi4pyã®äœ¿çšã§ããããã«ããã倿°ã®ã¢ã³ãã«ã«ãã·ãã¥ã¬ãŒã·ã§ã³ã§äœæ¥ããã¯ã©ã¹ã¿ãŒã䜿çšããŠã¢ãã«ãå®è¡ããã³ãã¹ãã§ããŸããæ¬¡ã®ã¹ãããã¯ãPythonçšã®Cããã°ã©ãã³ã°èšèªããå®éã®ãMPIãŸãã¯SWIGïŒhttps://ru.wikipedia.org/wiki/SWIGïŒãã¯ãããžãŒã®äœ¿çšã«åºã¥ããŠãã¢ãã«ãããã«æ¹åããããšã§ãããã®ã¢ãã«ã¯ä»¥åã®ã¢ãã«ãšã»ãšãã©åãã§ãããmpi4pyããã«ãããã»ãã·ã³ã°ãšçµæã®çµ±åã«äœ¿çšãããç¹ãç°ãªããŸãïŒå³23ïŒã

å³ 23.çµ±èšMPIã¢ãã«
åã®ã¢ãã«ã«å ããŠãããã€ãã®è¿œå ã¢ãžã¥ãŒã«ãã€ã³ããŒãããå¿ èŠããããŸãããã®æ®µéã§ããã«ãã¹ããè¡ããããprint_resultsïŒïŒé¢æ°ãæžãæããå¿ èŠããããŸãããŸããããã°ã©ã ã®äž»èŠéšåãæžãæããå¿ èŠããããŸããå³24ã§ã¯ã以åã®ã¢ãã«ã®ã³ãŒããšã¯ç°ãªãããã°ã©ã ã³ãŒãã®éšåã®ã¿ãæäŸããŠããŸããã·ãã¥ã¬ãŒã·ã§ã³çµæãå³25ã«ç€ºããŸãã
.................... import sys from mpi4py import MPI .................... def print_results(in_seq): "Output rezults" f=open("out.txt","a") for m in range(int(size)): for j in range(MKS): for i in range(TMPN-l): f.write("%f%s" % (in_seq[m][i+j*TMPN].st,",")) f.write("%f\n" % (in_seq[m][(TMPN-l)+j*TMPN].st)) f.close() .................... stt=time.time() #start time for the process rank = MPI.COMM_WORLD.Get_rank() size = MPI.COMM_WORLD.Get_size() name = MPI.Get_processor_name() N= 10**3 ## Clients M=5 ## Servers ## Input/sevice frequency glambda=[30000]+[i for i in linspace(25000,5000,M)] ## Number of Monte-Carlo simulations for this particuar process MKS=20 TMPN=200 ## Number of points in printing templat template= map(int,linspace(0,N-1,TMPN)) ## points for printing p=[] results=[] ## this process results total_results=[] ## overall results for i in range(M):p +=[server(i)] for i in range(MKS):results+=producer() total_results=MPI.COMM_WORLD.gather(results,0) random.seed(rank) if rank == 0: print_header() print_results(total_results) sys.stdout.write("Processing time: %d\n" % int(time.time()-stt))
å³ 24. MPIããŒã¹ã®çµ±èšã¢ãã«ã®Pythonã³ãŒã
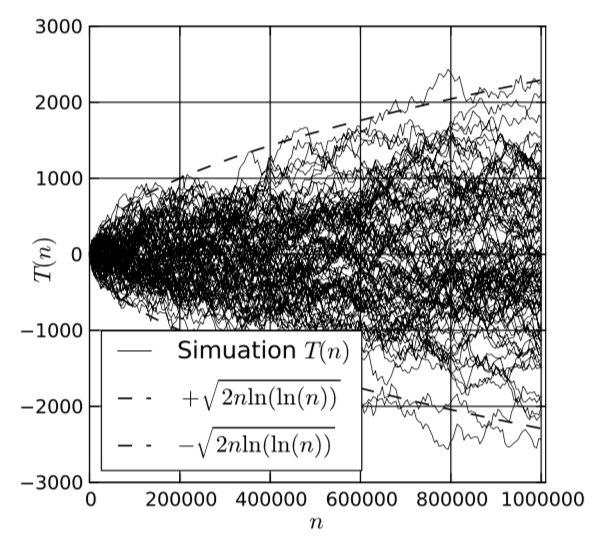
å³ 25.çµ±èšã¢ãã«MPIã®ã·ãã¥ã¬ãŒã·ã§ã³çµæ
6.çµè«
ãã®èšäºã§ã¯ãã·ãã¥ã¬ãŒã·ã§ã³ããŒã¹ã®åŠç¿ã®ããã®ããã€ãã®ã¢ãã«ã調ã¹ãŸããããããã®ã¢ãã«ã«ãããåŠçã¯äžé£ã®å®éšã宿œããç§åŠæ å ±åŠã®åéã«å¯Ÿããçè§£ãæ·±ããããšãã§ããŸããæç€ºãããã¢ãã«ãšãã®ãããªã¢ãã«ã®å®éšã«ã¯ãããã€ãã®ã¬ãã«ã®è€éãããããŸããæåã®ã¬ãã«ã¯åºæ¬ã§ããã©ã³ãã 倿°ã®çè§£ã«ã€ãªããããŸãç§åŠç ç©¶ã®åéã®äž»èŠãªçè§£ãäžããŸããæ¬¡ã®ã¬ãã«ã¯ããè€éã§ã䞊åããã°ã©ãã³ã°ãšç¢ºççã¢ããªã³ã°ã®ããæ·±ãçè§£ãæäŸããŸããé¢é£ããçè«çç¥èãæç€ºãããå¿ èŠã«å¿ããŠã远å è³æãšããŠäœ¿çšã§ããŸãããããã¯ãã¹ãŠãç§åŠæ å ±åŠå ¥éçšã®åºæ¬çãªããŒã«ããããæäŸããŸãããããŠãçµè«ãšããŠãã¢ãã«ã®ãããªãç ç©¶ãšæ¹åã®ããã®ææ¡ãããããšæããŸãã
6.1ã ã¢ãã«ã®ç·åœ¢æ§ãšQSã®çµ±èšãã©ã¡ãŒã¿ãŒ
ãã®èšäºã§ç޹ä»ããå€çžQSã¢ãã«ã¯ç·åœ¢ã§ã¯ãããŸãã[12]ãããã¯ãéç·åœ¢æ°åŠé¢æ°maxãå«ããããååž°æ¹çšåŒããæããã«ãªããŸããç¹ã«QSã®çµ±èšãã©ã¡ãŒã¿ãŒãèšç®ããå Žåã«ãæ£ããã·ãã¥ã¬ãŒã·ã§ã³çµæãååŸããå Žåã¯ãèšç®ã«éšåç·åœ¢ã¢ãã«ã䜿çšããå¿ èŠããããŸããããã¯ãããŒããããŠããªããã©ã³ã¹ããŒãã·ã¹ãã ã«ãšã£ãŠç¹ã«éèŠã§ããããããªããšãèšç®ã§ããªã倧ããªèª€å·®ãçããå¯èœæ§ãããããã§ãã
6.2ã Pythonã¢ãžã¥ãŒã«ã®æ¡åŒµæ©èœãšCããã°ã©ãã³ã°
çç·ŽããåŠçã«ãšã£ãŠãããã°ã©ã ã³ãŒãã®æå¹æ§ãæ¹åãç¶ããããšã¯è峿·±ããããããŸãããããã¯ãSWINGãã¯ãããžãŒã䜿çšããŠå®è£ ãããC颿°ã§Pythonã¢ãžã¥ãŒã«ãæ¡åŒµããããšã§å®è¡ã§ããŸããCythonãCããã°ã©ãã³ã°èšèªããå®éã®ãMPIãã¯ãããžãŒãããã³ã¯ã©ã¹ã¿ãŒã·ã¹ãã ã®HTCïŒé«æ§èœã³ã³ãã¥ãŒãã£ã³ã°ïŒã䜿çšããŠãã³ãŒãèšç®ãæ¹åããèšç®ãé«éåããããšãã§ããŸã[5ã28ã29]ã
6.3ããœãããŠã§ã¢ãœãªã¥ãŒã·ã§ã³ã®å¹çæ§ãšãããªãéçº
ãã®ã»ã¯ã·ã§ã³ã§ã¯ãåŠçã¯ããŸããŸãªãœãããŠã§ã¢ãœãªã¥ãŒã·ã§ã³ã®æå¹æ§ã調ã¹ãããšãã§ããŸãããã®ãããã¯ã¯ã䞊åèšç®ã«åºã¥ããœãããŠã§ã¢ã¢ãã«ã«ãšã£ãŠéèŠã§ããåŠçã¯ãããŸããŸãªãœãããŠã§ã¢ã¢ãã«ã®æå¹æ§ãç ç©¶ããæ®µéçã«ã¢ã«ãŽãªãºã ãæ¹åããããšããããšãã§ããŸããããã§ã®éèŠãªãã€ã³ãã¯ãæ å ±ãããŒã®æ°ãšããŸããŸãªãœãããŠã§ã¢ããã»ã¹ã®èšç®ã®æ¯çã®ç ç©¶ã§ãããããã£ãŠããã®æ¯çã¯ã䞊åã³ã³ãã¥ãŒãã£ã³ã°ã䜿çšããããã°ã©ã ã®æã广çãªéçºãæ§ç¯ããäžã§éèŠã§ããå¥ã®è峿·±ããããã¯ã¯ãã¢ã«ãŽãªãºã æ§é ãHTCã¯ã©ã¹ã¿ãŒæ§é ã«å€æããå¯èœæ§ã®ç ç©¶ã§ãã
ç ç©¶ã®è¿œå ã¿ã¹ã¯ãšããŠãèè ã¯QSã¢ããªã³ã°ãæ€èšããŸããQSã¢ããªã³ã°ã¯ã¢ãã«åããã³åæããå¿ èŠããããŸããQSã®æ¯èŒçè€éãªæ§è³ªãšå¯Ÿå¿ããçš®é¡ã®ã¢ããªã±ãŒã·ã§ã³ã§ã¯ãããåºç¯ãªããã°ã©ãã³ã°ææ³ã䜿çšããå¿ èŠããããŸãããã®ããã«ããŠãç¶æ¿ãã«ãã»ã«åãããªã¢ãŒãã£ãºã ãªã©ã®äžè¬çãªããã°ã©ãã³ã°æŠå¿µãå®è£ ããããã®åªããåºæ¬ãã©ãããã©ãŒã ã衚瀺ãããŸããäžæ¹ãã³ã³ãã¥ãŒã¿ãŒãµã€ãšã³ã¹ã®åºæ¬çãªçè«çæŠå¿µã匷調ããå¿ èŠããããŸããããããã¹ãŠã«å ããŠãQSã®çµ±èšããã³ã·ãã¥ã¬ãŒã·ã§ã³ã¢ããªã³ã°ã«ã¯ã確ççè«ã®åéã§ã®ããé«åºŠãªç¥èãããå€ãã®ã³ã³ãã¥ãŒãã£ã³ã°ãªãœãŒã¹ã®äœ¿çšãå®éã®ç§åŠçã³ã³ãã¥ãŒãã£ã³ã°ç°å¢ã®æäŸãããã³äžçŽåŠçã«å¯Ÿããåªããã¢ãããŒã·ã§ã³ãå¿ èŠã§ãã
æåŠ
åç
§ã®å®å
šãªãªã¹ã
[1] A. Arazi, E. Ben-Jacob and U. Yechiali, Bridging genetic net- works and queueing theory, Physica A: Statistical Mechanics and Its Applications 332 (2004), 585â616.
[2] DM Beazley, Python Essential Reference, Addison-Wesley Professional, 2009.
[3] J. Bernard, Use Python for scientific computing, Linux Journal 175 (2008), 7.
[4] UN Bhat, An Introduction to Queueing Theory Modeling and Analysis in Applications, BirkhÀuser, Boston, MA, 2008.
[5] KJ Bogacev, Basics of Parallel Programming, Binom, Moscow, 2003.
[6] RN Caine and G. Caine, Making Connections: Teaching andthe Human Brain, Association for Supervision and Curriculum Development, Alexandria, 1991.
[7] J. Clement and MA Rea, Model Based Learning and Instruction in Science, Springer, The Netherlands, 2008.
[8] NA Cookson, WH Mather, T. Danino, O. Mondragón- Palomino, RJ Williams, LS Tsimring and J. Hasty, Queue- ing up for enzymatic processing: correlated signaling through coupled degradation, Molecular Systems Biology 7 (2011), 1. [9] AS Gibbons, Model-centered instruction, Journal of Structural Learning and Intelligent Systems 4 (2001), 511â540. [10] MT Heath, Scientific Computing an Introductory Survey, McGraw-Hill, New York, 1997.
[11] A. Hellander, Stochastic Simulation and Monte Carlo Meth- ods, 2009.
[12] GI Ivcenko, VA Kastanov and IN Kovalenko, Queuing System Theory, Visshaja Shkola, Moscow, 1982.
[13] ZL Joel, NW Wei, J. Louis and TS Chuan, Discreteâevent
simulation of queuing systems, in: Sixth Youth Science Con- ference, Singapore Ministry of Education, Singapore, 2000, pp. 1â5.
[14] E. Jones, Introduction to scientific computing with Python, in: SciPy, California Institute of Technology, Pasadena, CA, 2007, p. 333.
[15] M. Joubert and P. Andrews, Research and developments in probability education internationally, in: British Congress for Mathematics Education, 2010, p. 41ã
[16] GE Karniadakis and RM Kyrby, Parallel Scientific Comput- ing in C++ and MPI. A Seamless Approach to Parallel Al- gorithms and Their Implementation, Cambridge Univ. Press, 2003.
[17] DG Kendall, Stochastic processes occurring in the theory of queues and their analysis by the method of the imbedded Markov chain, The Annals of Mathematical Statistics 1 (1953), 338â354.
[18] MS Khine and IM Saleh, Models and modeling, cognitive tools for scientific enquiry, in: Models and Modeling in Science Education, Springer, 2011, p. 290.
[19] T. Kiesling and T. Krieger, Efficient parallel queuing system simulation, in: The 38th Conference on Winter Simulation, Winter Simulation Conference, 2006, pp. 1020â1027.
[20] J. Kiusalaas, Numerical Methods in Engineering with Python, Cambridge Univ. Press, 2010.
[21] A. Kumar, Python for Education. Learning Maths & Science Using Python and Writing Them in LATEX, Inter University Accelerator Centre, New Delhi, 2010.
[22] HP Langtangen, Python Scripting for Computational Science, Springer-Verlag, Berlin, 2009.
[23] HP Langtangen, A Primer on Scientific Programming with Python, Springer-Verlag, Berlin, 2011.
[24] HP Langtangen, Experience with using Python as a primary language for teaching scientific computing at the University of Oslo, University of Oslo, 2012.
[25] R. Lehrer and L. Schauble, Cultivating model-based reason- ing in science education, in: The Cambridge Handbook of the Learning Sciences, Cambridge Univ. Press, 2005, pp. 371â388.
[26] G. Levy, An introduction to quasi-random numbers, in: Nu- merical Algorithms, Group, 2012.
[27] JS Liu, Monte Carlo Strategies in Scientific Computing, Har- vard Univ., 2001.
[28] VE Malishkin and VD Korneev, Parallel Programming of Multicomputers, Novosibirsk Technical Univ., Novosibirsk, 2006.
[29] N. Matloff, Programming on Parallel Machines: GPU, Multi- core, Clusters and More, University of California, 2012.
[30] M. Milrad, JM Spector and PI Davidsen, Model facilitated learning, in: Instructional Design, Development and Evalua- tion, Syracuse Univ. Press, 2003.
[31] S. MinkevicËius, On the law of the iterated logarithm in multi- phase queueing systems, Informatica II (1997), 367â376.
[32] S. MinkevicËius and V. Dolgopolovas, Analysis of the law of the iterated logarithm for the idle time of a customer in multiphase queues, Int. J. Pure Appl. Math. 66 (2011), 183â190.
[33] Model-Centered Learning, Pathways to mathematical under- standing using GeoGebra, in: Modeling and Simulations for Learning and Instruction, Sense Publishers, The Netherlands, 2011.
[34] CR Myers and JP Sethna, Python for education: Computa- tional methods for nonlinear systems, Computing in Science & Engineering 9 (2007), 75â79.
[35] H. Niederreiter, Random Number Generation and Quasi- Monte Carlo Methods, SIAM, 1992.
[36] FB Nilsen, Queuing systems: Modeling, analysis and simu- lation, Department of Informatics, University of Oslo, Oslo, 1998.
[37] RP Sen, Operations Research: Algorithms and Applications, PHI Learning, 2010.
[38] F. Stajano, Python in education: Raising a generation of native speakers, in: 8th International Python Conference, Washing- ton, DC, 2000, pp. 1â5.
[39] J. Sztrik, Finite-source queueing systems and their applica- tions, Formal Methods in Computing 1 (2001), 7â10.
[40] L. Xue, Modeling and simulation in scientific computing ed- ucation, in: International Conference on Scalable Computing and Communications, 2009, pp. 577â580.
[2] DM Beazley, Python Essential Reference, Addison-Wesley Professional, 2009.
[3] J. Bernard, Use Python for scientific computing, Linux Journal 175 (2008), 7.
[4] UN Bhat, An Introduction to Queueing Theory Modeling and Analysis in Applications, BirkhÀuser, Boston, MA, 2008.
[5] KJ Bogacev, Basics of Parallel Programming, Binom, Moscow, 2003.
[6] RN Caine and G. Caine, Making Connections: Teaching andthe Human Brain, Association for Supervision and Curriculum Development, Alexandria, 1991.
[7] J. Clement and MA Rea, Model Based Learning and Instruction in Science, Springer, The Netherlands, 2008.
[8] NA Cookson, WH Mather, T. Danino, O. Mondragón- Palomino, RJ Williams, LS Tsimring and J. Hasty, Queue- ing up for enzymatic processing: correlated signaling through coupled degradation, Molecular Systems Biology 7 (2011), 1. [9] AS Gibbons, Model-centered instruction, Journal of Structural Learning and Intelligent Systems 4 (2001), 511â540. [10] MT Heath, Scientific Computing an Introductory Survey, McGraw-Hill, New York, 1997.
[11] A. Hellander, Stochastic Simulation and Monte Carlo Meth- ods, 2009.
[12] GI Ivcenko, VA Kastanov and IN Kovalenko, Queuing System Theory, Visshaja Shkola, Moscow, 1982.
[13] ZL Joel, NW Wei, J. Louis and TS Chuan, Discreteâevent
simulation of queuing systems, in: Sixth Youth Science Con- ference, Singapore Ministry of Education, Singapore, 2000, pp. 1â5.
[14] E. Jones, Introduction to scientific computing with Python, in: SciPy, California Institute of Technology, Pasadena, CA, 2007, p. 333.
[15] M. Joubert and P. Andrews, Research and developments in probability education internationally, in: British Congress for Mathematics Education, 2010, p. 41ã
[16] GE Karniadakis and RM Kyrby, Parallel Scientific Comput- ing in C++ and MPI. A Seamless Approach to Parallel Al- gorithms and Their Implementation, Cambridge Univ. Press, 2003.
[17] DG Kendall, Stochastic processes occurring in the theory of queues and their analysis by the method of the imbedded Markov chain, The Annals of Mathematical Statistics 1 (1953), 338â354.
[18] MS Khine and IM Saleh, Models and modeling, cognitive tools for scientific enquiry, in: Models and Modeling in Science Education, Springer, 2011, p. 290.
[19] T. Kiesling and T. Krieger, Efficient parallel queuing system simulation, in: The 38th Conference on Winter Simulation, Winter Simulation Conference, 2006, pp. 1020â1027.
[20] J. Kiusalaas, Numerical Methods in Engineering with Python, Cambridge Univ. Press, 2010.
[21] A. Kumar, Python for Education. Learning Maths & Science Using Python and Writing Them in LATEX, Inter University Accelerator Centre, New Delhi, 2010.
[22] HP Langtangen, Python Scripting for Computational Science, Springer-Verlag, Berlin, 2009.
[23] HP Langtangen, A Primer on Scientific Programming with Python, Springer-Verlag, Berlin, 2011.
[24] HP Langtangen, Experience with using Python as a primary language for teaching scientific computing at the University of Oslo, University of Oslo, 2012.
[25] R. Lehrer and L. Schauble, Cultivating model-based reason- ing in science education, in: The Cambridge Handbook of the Learning Sciences, Cambridge Univ. Press, 2005, pp. 371â388.
[26] G. Levy, An introduction to quasi-random numbers, in: Nu- merical Algorithms, Group, 2012.
[27] JS Liu, Monte Carlo Strategies in Scientific Computing, Har- vard Univ., 2001.
[28] VE Malishkin and VD Korneev, Parallel Programming of Multicomputers, Novosibirsk Technical Univ., Novosibirsk, 2006.
[29] N. Matloff, Programming on Parallel Machines: GPU, Multi- core, Clusters and More, University of California, 2012.
[30] M. Milrad, JM Spector and PI Davidsen, Model facilitated learning, in: Instructional Design, Development and Evalua- tion, Syracuse Univ. Press, 2003.
[31] S. MinkevicËius, On the law of the iterated logarithm in multi- phase queueing systems, Informatica II (1997), 367â376.
[32] S. MinkevicËius and V. Dolgopolovas, Analysis of the law of the iterated logarithm for the idle time of a customer in multiphase queues, Int. J. Pure Appl. Math. 66 (2011), 183â190.
[33] Model-Centered Learning, Pathways to mathematical under- standing using GeoGebra, in: Modeling and Simulations for Learning and Instruction, Sense Publishers, The Netherlands, 2011.
[34] CR Myers and JP Sethna, Python for education: Computa- tional methods for nonlinear systems, Computing in Science & Engineering 9 (2007), 75â79.
[35] H. Niederreiter, Random Number Generation and Quasi- Monte Carlo Methods, SIAM, 1992.
[36] FB Nilsen, Queuing systems: Modeling, analysis and simu- lation, Department of Informatics, University of Oslo, Oslo, 1998.
[37] RP Sen, Operations Research: Algorithms and Applications, PHI Learning, 2010.
[38] F. Stajano, Python in education: Raising a generation of native speakers, in: 8th International Python Conference, Washing- ton, DC, 2000, pp. 1â5.
[39] J. Sztrik, Finite-source queueing systems and their applica- tions, Formal Methods in Computing 1 (2001), 7â10.
[40] L. Xue, Modeling and simulation in scientific computing ed- ucation, in: International Conference on Scalable Computing and Communications, 2009, pp. 577â580.